Genome-wide family prediction unveils molecular mechanisms underlying the regulation of agronomic traits in Urochloa ruziziensis
biorxiv(2023)
Abstract
Tropical forage grasses, especially species of the genus Urochloa , play an important role in cattle production and are the main food source for animals in tropical/subtropical regions. Most of the species are apomictic and tetraploid, which gives special importance to U. ruziziensis , a sexual diploid species that can be tetraploidized for use in interspecific crosses with apomictic species. As a means to assist in breeding programs, this study investigates the applicability of genome-wide family prediction (GWFP) in U. ruziziensis half-sibling families to predict growth and biomass production. Machine learning and feature selection algorithms were used to reduce the necessary number of markers for prediction and to enhance the predictive ability across the phenotypes. Beyond that, to investigate the regulation of agronomic traits, the positions of the markers with more importance for the prediction were considered putatively associated to quantitative trait loci (QTLs), and in a multiomic approach, genes obtained in the species transcriptome were mapped and linked to those markers. Furthermore, a gene coexpression network was modeled, enabling the investigation of not only the mapped genes but also their coexpressed genes. The functional annotation showed that the mapped genes are mainly associated with auxin transport and biosynthesis of lignin, flavonol and folic acid, while the coexpressed genes are associated with DNA metabolism, stress response and circadian rhythm. The results provide a viable marker-assisted breeding approach for tropical forages and identify target regions for future molecular studies on these agronomic traits.
### Competing Interest Statement
The authors have declared no competing interest.
MoreTranslated text
Key words
feature selection,forage grasses,gene coexpression networks,genomic prediction,machine learning,major importance markers,RNA-Seq
AI Read Science
Must-Reading Tree
Example
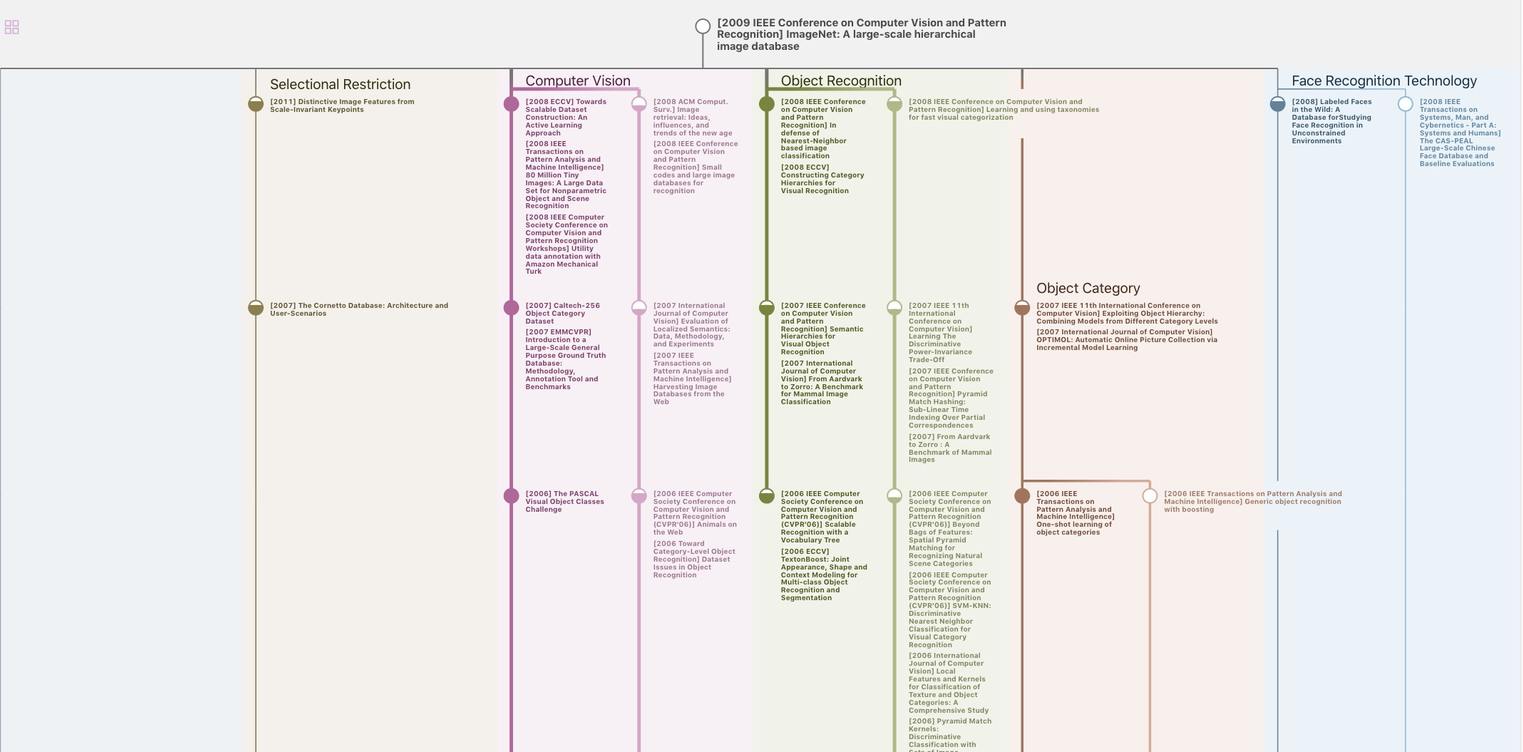
Generate MRT to find the research sequence of this paper
Chat Paper
Summary is being generated by the instructions you defined