Deep Learning Empowers the Discovery of Self-Assembling Peptides with Over 10 Trillion Sequences.
Advanced science (Weinheim, Baden-Wurttemberg, Germany)(2023)
摘要
Self-assembling of peptides is essential for a variety of biological and medical applications. However, it is challenging to investigate the self-assembling properties of peptides within the complete sequence space due to the enormous sequence quantities. Here, it is demonstrated that a transformer-based deep learning model is effective in predicting the aggregation propensity (AP) of peptide systems, even for decapeptide and mixed-pentapeptide systems with over 10 trillion sequence quantities. Based on the predicted AP values, not only the aggregation laws for designing self-assembling peptides are derived, but the transferability relation among the APs of pentapeptides, decapeptides, and mixed pentapeptides is also revealed, leading to discoveries of self-assembling peptides by concatenating or mixing, as consolidated by experiments. This deep learning approach enables speedy, accurate, and thorough search and design of self-assembling peptides within the complete sequence space of oligopeptides, advancing peptide science by inspiring new biological and medical applications.
更多查看译文
关键词
aggregation laws,deep learning,oligopeptides,self‐assembling
AI 理解论文
溯源树
样例
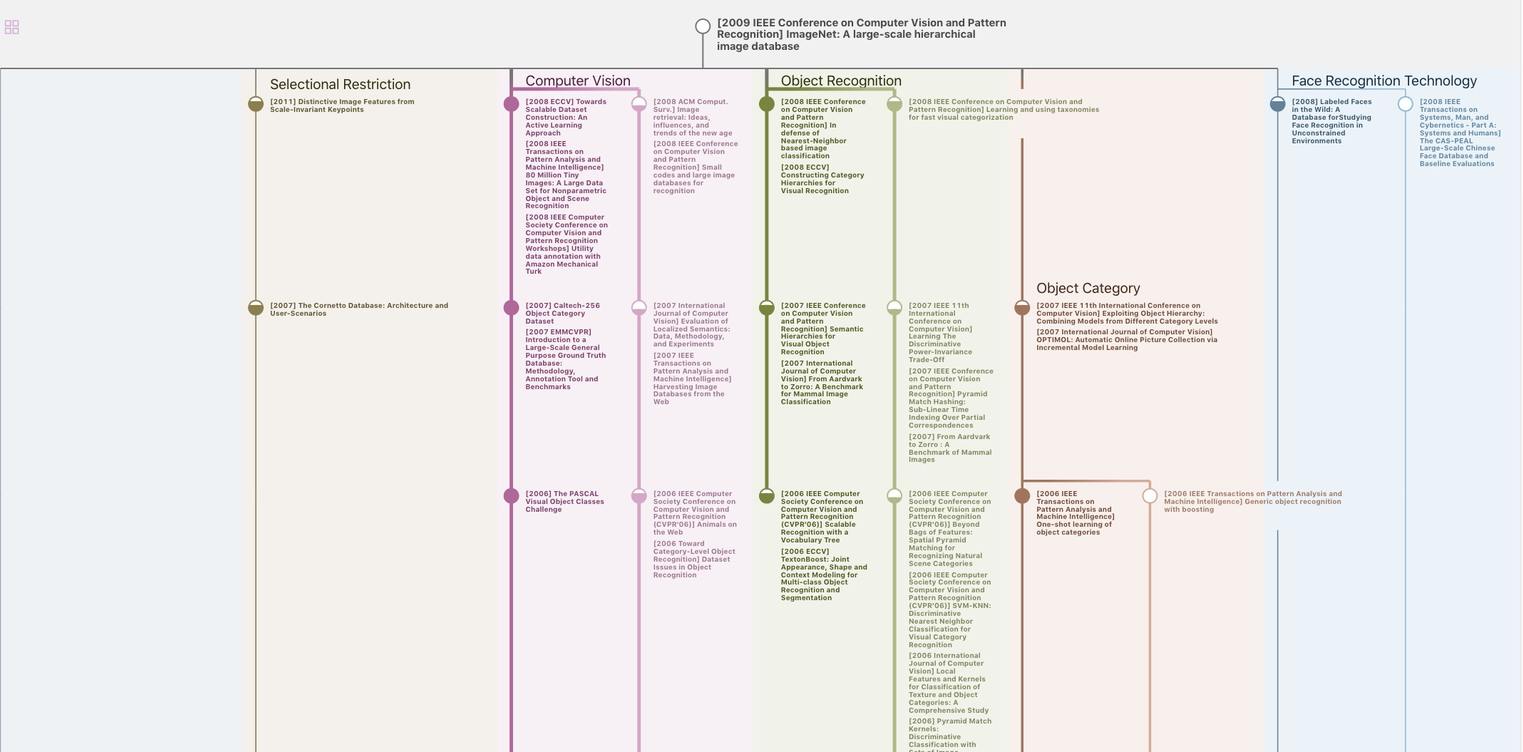
生成溯源树,研究论文发展脉络
Chat Paper
正在生成论文摘要