MFD-Net: Modality Fusion Diffractive Network for Segmentation of Multimodal Brain Tumor Image
IEEE JOURNAL OF BIOMEDICAL AND HEALTH INFORMATICS(2023)
摘要
Automatic brain tumor segmentation using multi-parametric magnetic resonance imaging (mpMRI) holds substantial importance for brain diagnosis, monitoring, and therapeutic strategy planning. Given the constraints inherent to manual segmentation, adopting deep learning networks for accomplishing accurate and automated segmentation emerges as an essential advancement. In this article, we propose a modality fusion diffractive network (MFD-Net) composed of diffractive blocks and modality feature extractors for the automatic and accurate segmentation of brain tumors. The diffractive block, designed based on Fraunhofer's single-slit diffraction principle, emphasizes neighboring high-confidence feature points and suppresses low-quality or isolated feature points, enhancing the interrelation of features. Adopting a global passive reception mode overcomes the issue of fixed receptive fields. Through a self-supervised approach, the modality feature extractor effectively utilizes the inherent generalization information of each modality, enabling the main segmentation branch to focus more on multimodal fusion feature information. We apply the diffractive block on nn-UNet in the MICCAI BraTS 2022 challenge, ranked first in the pediatric population data and third in the BraTS continuous evaluation data, proving the superior generalizability of our network. We also train separately on the BraTS 2018, 2019, and 2021 datasets. Experiments demonstrate that the proposed network outperforms state-of-the-art methods.
更多查看译文
关键词
Brain tumor segmentation,diffractive block,magnetic resonance images
AI 理解论文
溯源树
样例
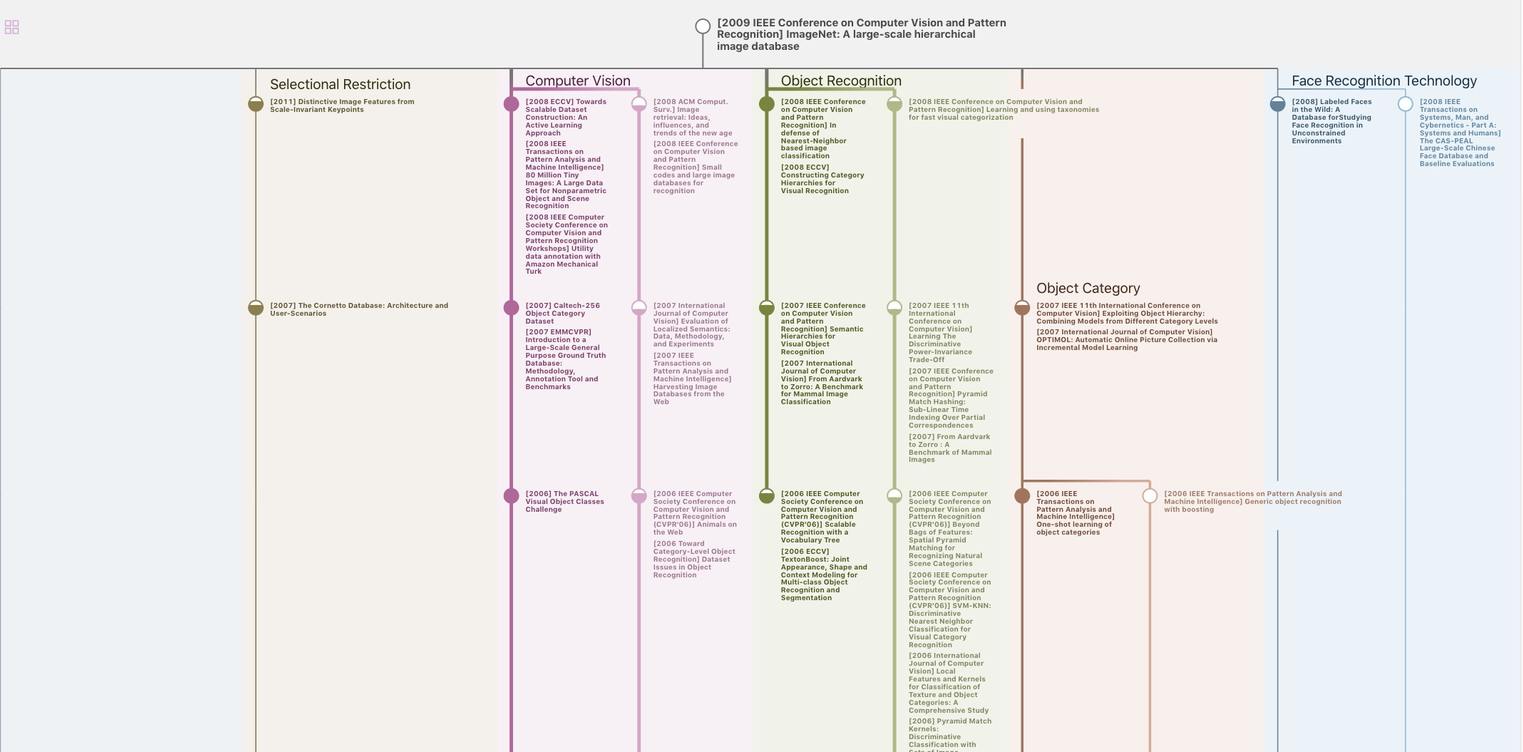
生成溯源树,研究论文发展脉络
Chat Paper
正在生成论文摘要