The Role of Document Embedding in Research Paper Recommender Systems: To Breakdown or to Bolster Disciplinary Borders?
CoRR(2023)
摘要
In the extensive recommender systems literature, novelty and diversity have been identified as key properties of useful recommendations. However, these properties have received limited attention in the specific sub-field of research paper recommender systems. In this work, we argue for the importance of offering novel and diverse research paper recommendations to scientists. This approach aims to reduce siloed reading, break down filter bubbles, and promote interdisciplinary research. We propose a novel framework for evaluating the novelty and diversity of research paper recommendations that leverages methods from network analysis and natural language processing. Using this framework, we show that the choice of representational method within a larger research paper recommendation system can have a measurable impact on the nature of downstream recommendations, specifically on their novelty and diversity. We introduce a novel paper embedding method, which we demonstrate offers more innovative and diverse recommendations without sacrificing precision, compared to other state-of-the-art baselines.
更多查看译文
关键词
research paper recommender systems,bolster disciplinary borders,document
AI 理解论文
溯源树
样例
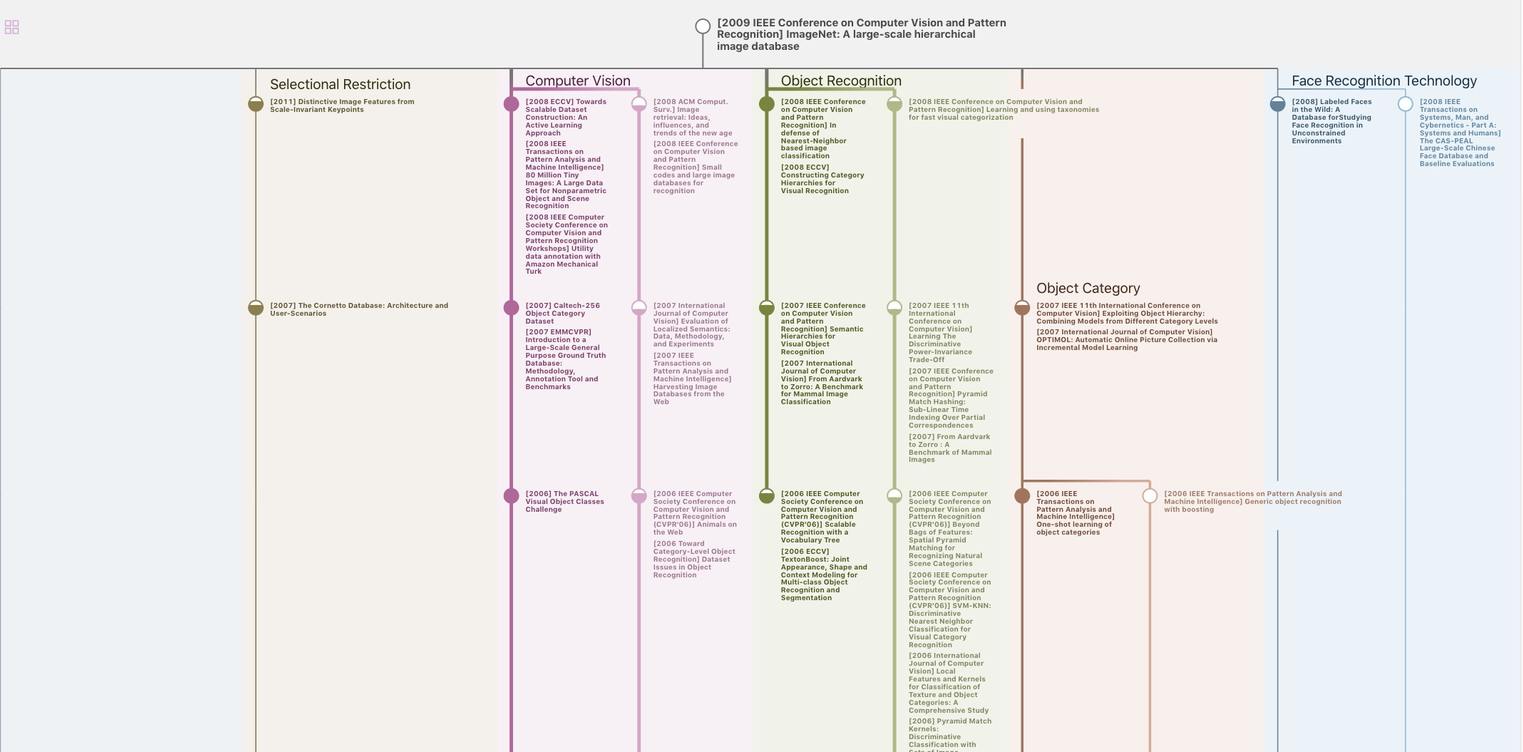
生成溯源树,研究论文发展脉络
Chat Paper
正在生成论文摘要