Building the I (Interoperability) of FAIR for Performance Reproducibility of Large-Scale Composable Workflows in RECUP
2023 IEEE 19th International Conference on e-Science (e-Science)(2023)
摘要
Scientific computing communities increasingly run their experiments using complex data- and compute-intensive workflows that utilize distributed and heterogeneous architectures targeting numerical simulations and machine learning, often executed on the Department of Energy Leadership Computing Facilities (LCFs). We argue that a principled, systematic approach to implementing FAIR principles at scale, including fine-grained metadata extraction and organization, can help with the numerous challenges to performance reproducibility posed by such workflows. We extract workflow patterns, propose a set of tools to manage the entire life cycle of performance metadata, and aggregate them in an HPC-ready framework for reproducibility (RECUP). We describe the challenges in making these tools interoperable, preliminary work, and lessons learned from this experiment.
更多查看译文
关键词
High performance computing,HPC,performance reproducibility,workflow execution patterns,workflow execution provenance,metadata capture,research software engineering,FAIR4RS,FAIR4HPC,RO-Crate
AI 理解论文
溯源树
样例
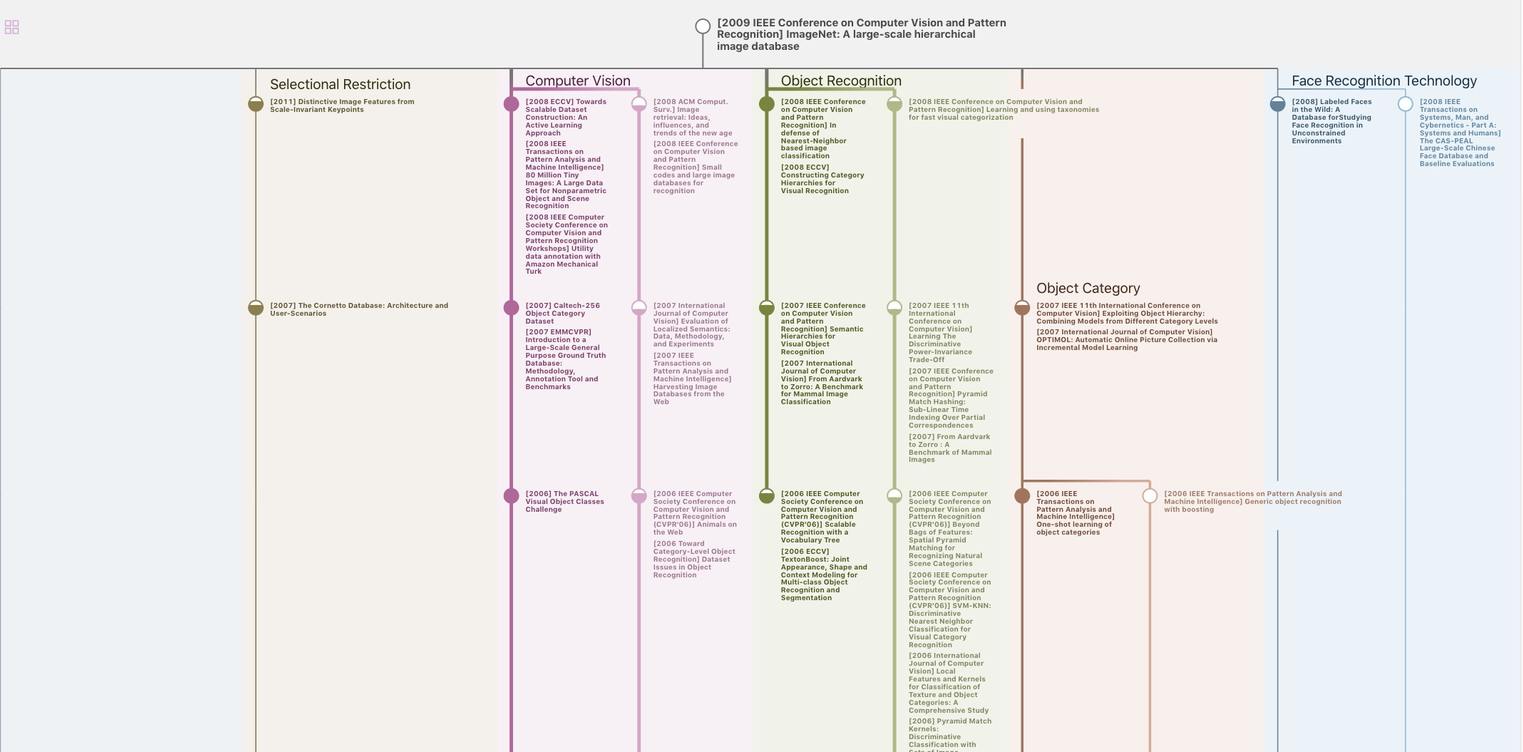
生成溯源树,研究论文发展脉络
Chat Paper
正在生成论文摘要