A Linear Programming Enhanced Genetic Algorithm for Hyperparameter Tuning in Machine Learning
2023 IEEE Congress on Evolutionary Computation (CEC)(2024)
Abstract
In this paper, we formulate the hyperparameter tuning problem in machine
learning as a bilevel program. The bilevel program is solved using a micro
genetic algorithm that is enhanced with a linear program. While the genetic
algorithm searches over discrete hyperparameters, the linear program
enhancement allows hyper local search over continuous hyperparameters. The
major contribution in this paper is the formulation of a linear program that
supports fast search over continuous hyperparameters, and can be integrated
with any hyperparameter search technique. It can also be applied directly on
any trained machine learning or deep learning model for the purpose of
fine-tuning. We test the performance of the proposed approach on two datasets,
MNIST and CIFAR-10. Our results clearly demonstrate that using the linear
program enhancement offers significant promise when incorporated with any
population-based approach for hyperparameter tuning.
MoreTranslated text
AI Read Science
Must-Reading Tree
Example
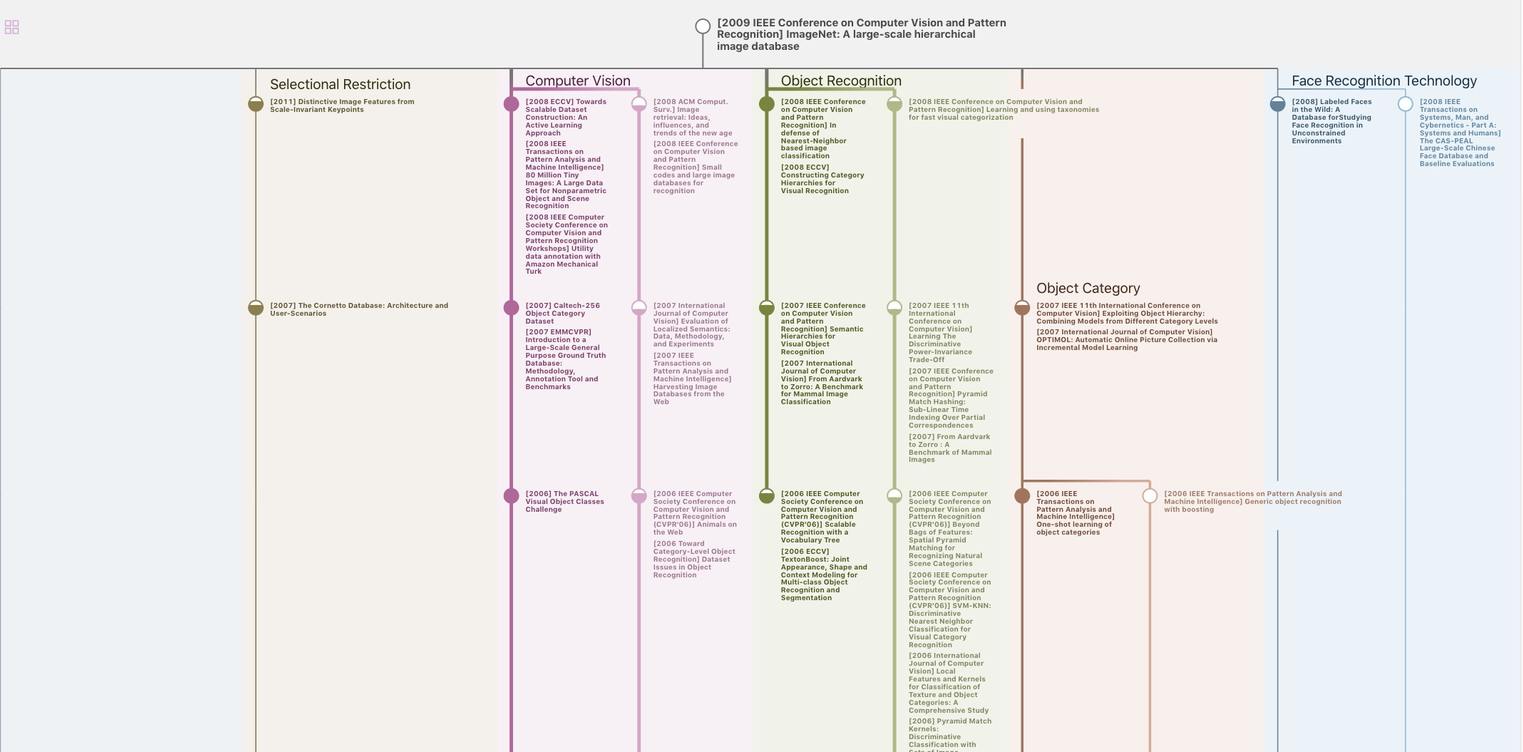
Generate MRT to find the research sequence of this paper
Chat Paper
Summary is being generated by the instructions you defined