Reinforcement Learning Based Voltage Control Using Multiple Control Devices
2023 IEEE Power & Energy Society General Meeting (PESGM)(2023)
Abstract
The increased penetration of renewable energy resources has increased system complexity and uncertainty. Operators are facing challenges dealing with voltage problems with a limited solution timeframe after disturbances. This paper presents a novel reinforcement learning (RL) based method to provide voltage control that can quickly remedy voltage violations under different operating conditions. Multiple types of devices, continuous ratio control based adjustable voltage ratio (AVR) transformers and discretely controlled switched shunts, are considered as controlled devices. A modified deep deterministic policy gradient (DDPG) algorithm is applied to accommodate both the continuous and discrete control action spaces of different devices, in addition to dealing with large action and state spaces. The proposed DDPG-based voltage control agent learns by interacting with the power system environment and eventually masters the control policy to determine the transformers’ ratios as well as the group size of the switched shunts. A case study conducted on the WECC 240-Bus system with transformers controlled only, and transformers and switched shunts controlled jointly validates the effectiveness of the proposed method and demonstrates that the multiple devices control method performs much better than a single device control.
MoreTranslated text
Key words
Reinforcement learning, voltage control, multi-device, DDPG, transformer, switched shunt
AI Read Science
Must-Reading Tree
Example
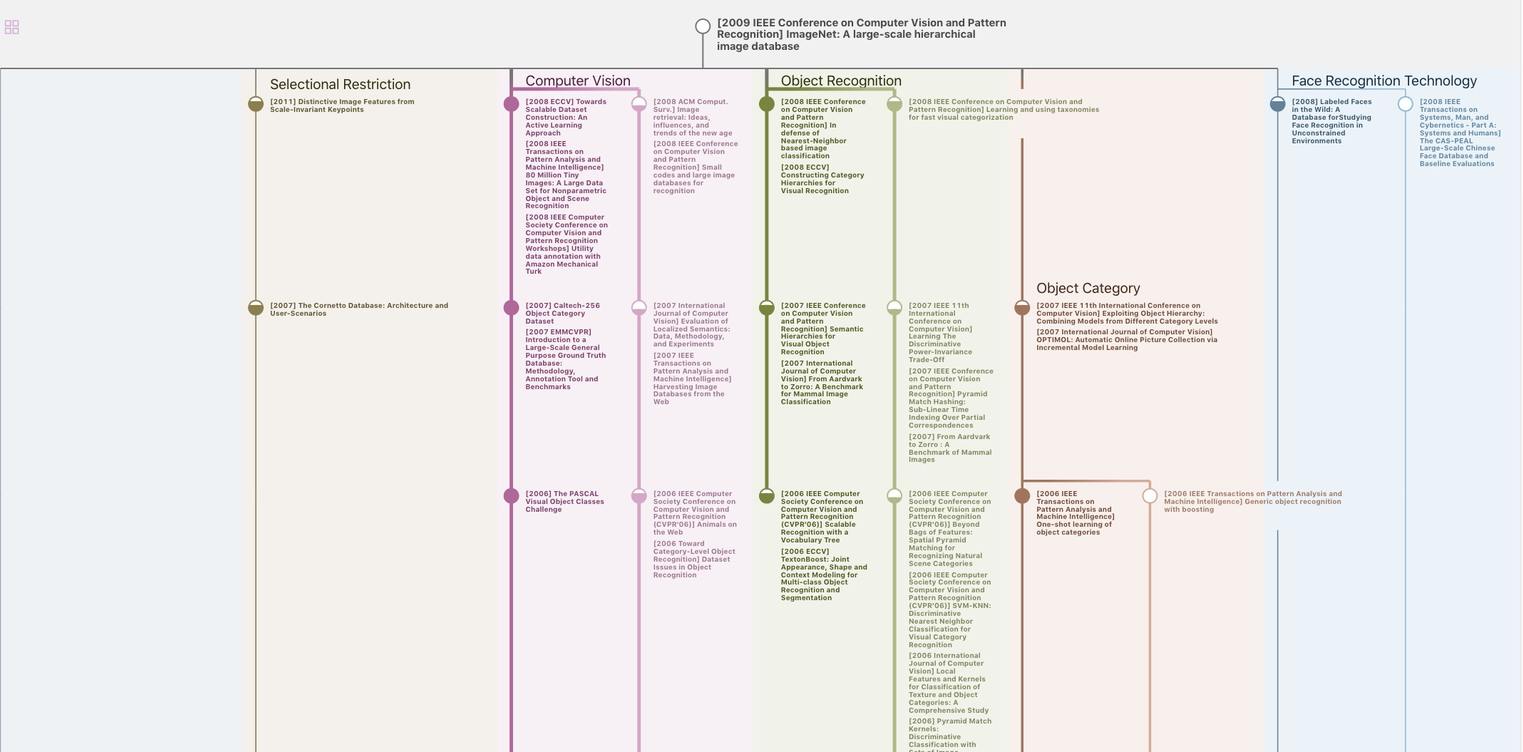
Generate MRT to find the research sequence of this paper
Chat Paper
Summary is being generated by the instructions you defined