FedGen: Generalizable Federated Learning for Sequential Data
2023 IEEE 16th International Conference on Cloud Computing (CLOUD)(2023)
摘要
Existing federated learning models that follow the standard risk minimization paradigm of machine learning often fail to generalize in the presence of spurious correlations in the training data. In many real-world distributed settings, spurious correlations exist due to biases and data sampling issues on distributed devices or clients that can erroneously influence models. Current generalization approaches are designed for centralized training and attempt to identify features that have an invariant causal relationship with the target, thereby reducing the effect of spurious features. However, such invariant risk minimization approaches rely on apriori knowledge of training data distributions which is hard to obtain in many applications. In this work, we present a generalizable federated learning framework called FedGen, which allows clients to identify and distinguish between spurious and invariant features in a collaborative manner without prior knowledge of training distributions. We evaluate our approach on real-world datasets from different domains and show that FedGen results in models that achieve significantly better generalization and can outperform the accuracy of current federated learning approaches by over 24%.
更多查看译文
关键词
generalizable federated learning,sequential data,fedgen
AI 理解论文
溯源树
样例
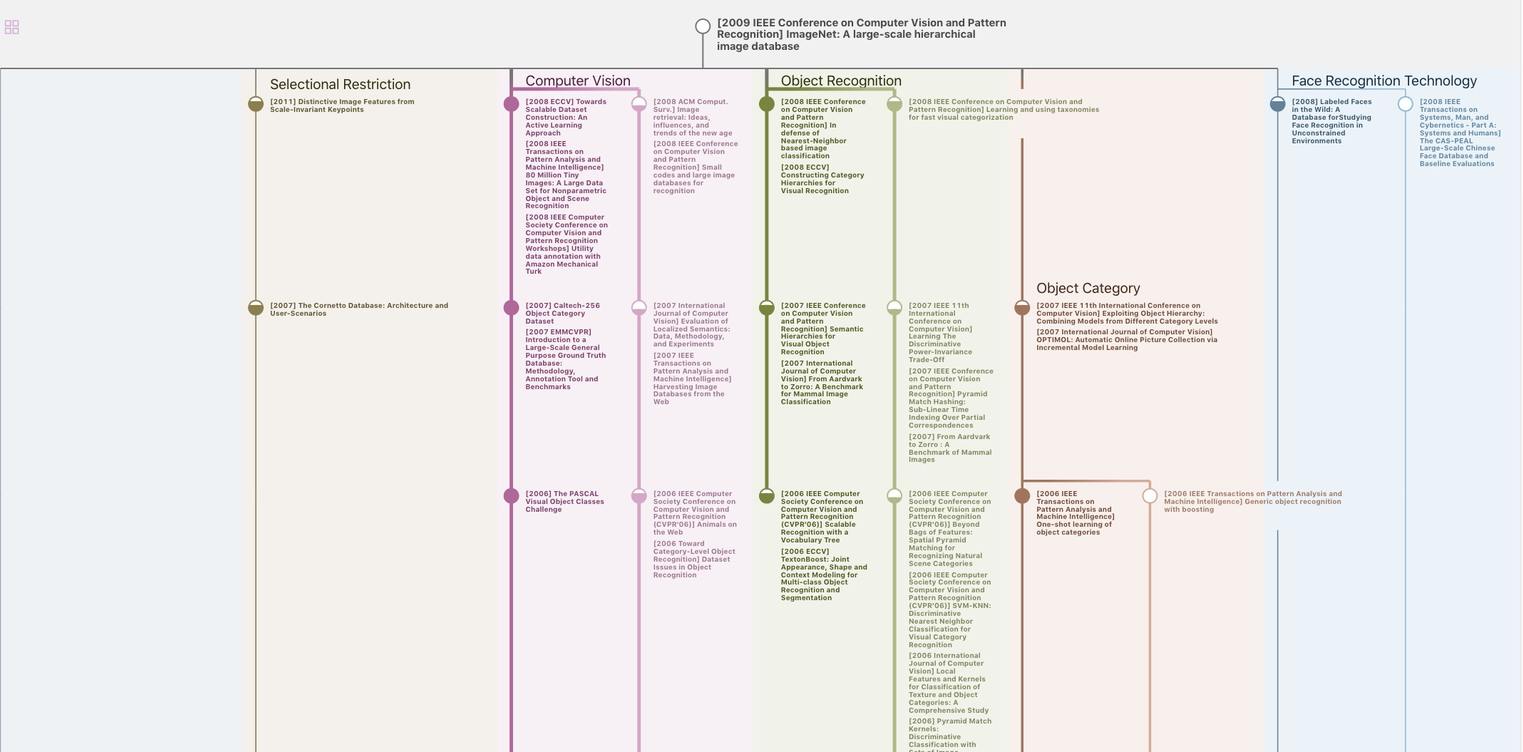
生成溯源树,研究论文发展脉络
Chat Paper
正在生成论文摘要