An Energy-Efficient Bayesian Neural Network Accelerator With CiM and a Time-Interleaved Hadamard Digital GRNG Using 22-nm FinFET
IEEE Journal of Solid-State Circuits(2023)
摘要
Bayesian neural networks (BNNs) have been proposed to address the problems of overfitting and overconfident decision making, common in conventional neural networks (NNs), due to their ability to model and express uncertainty in their predictions. However, BNNs require multiple inference passes to produce the necessary posterior distributions used to generate these highly desirable uncertainty estimates. As such, BNNs require not only an efficient, high-performance multiply-accumulation (MAC) operation but also an efficient Gaussian random number generator (GRNG) with high-quality statistics. In this article, an NN accelerator chip, leveraging a multi-bit analog compute-in-memory (CiM) static random-access memory (SRAM) macro, with a tightly coupled and highly efficient GRNG scheme, is presented in the Intel 22FFL process. The CiM macro achieves a peak energy efficiency of 32.2 TOP/sW, with 8-bit precision, while ensuring accurate on-chip matrix-vector multiplications (MVMs) with a computation error less than 0.5%. The variable precision GRNG achieves a peak throughput of 7.31 GSamp/s for an energy efficiency of
$\sim $
1 TSamp/J. Overall, our proposed system achieves a peak energy efficiency of 1170 GOP/s/W, a 35–133
$\times $
improvement over the state-of-the-art BNN accelerators, with 98.14% accuracy for the MNIST dataset.
更多查看译文
关键词
energy-efficient,time-interleaved
AI 理解论文
溯源树
样例
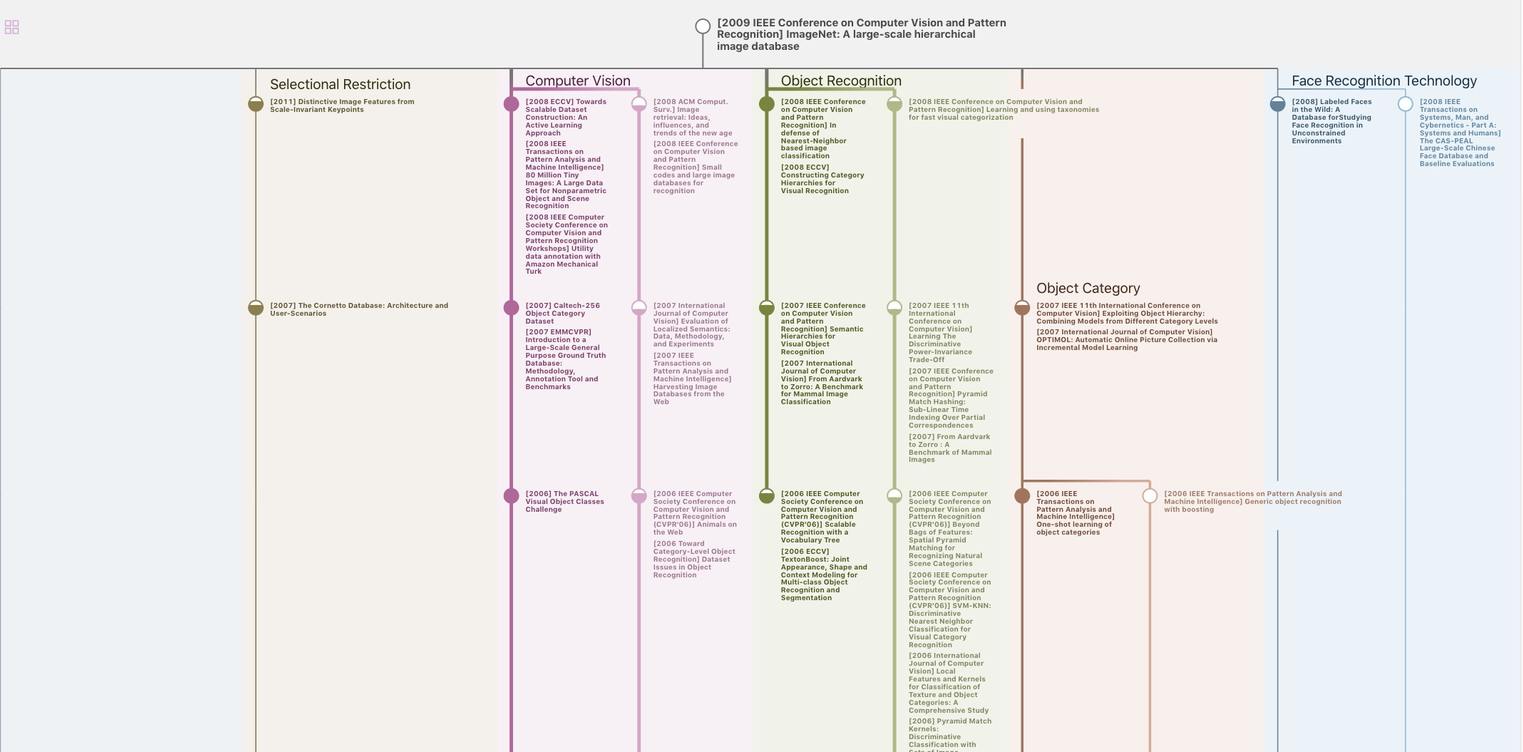
生成溯源树,研究论文发展脉络
Chat Paper
正在生成论文摘要