Groupwise structural sparsity for discriminative voxels identification.
Frontiers in neuroscience(2023)
摘要
This paper investigates the selection of voxels for functional Magnetic Resonance Imaging (fMRI) brain data. We aim to identify a comprehensive set of discriminative voxels associated with human learning when exposed to a neutral visual stimulus that predicts an aversive outcome. However, due to the nature of the unconditioned stimuli (typically a noxious stimulus), it is challenging to obtain sufficient sample sizes for psychological experiments, given the tolerability of the subjects and ethical considerations. We propose a stable hierarchical voting (SHV) mechanism based on stability selection to address this challenge. This mechanism enables us to evaluate the quality of spatial random sampling and minimizes the risk of false and missed detections. We assess the performance of the proposed algorithm using simulated and publicly available datasets. The experiments demonstrate that the regularization strategy choice significantly affects the results' interpretability. When applying our algorithm to our collected fMRI dataset, it successfully identifies sparse and closely related patterns across subjects and displays stable weight maps for three experimental phases under the fear conditioning paradigm. These findings strongly support the causal role of aversive conditioning in altering visual-cortical activity.
更多查看译文
关键词
fMRI,groupwise regularization,voxel selection,stable hierarchical voting (SHV),randomized structural sparsity (RSS),effective vote ratio (EVR)
AI 理解论文
溯源树
样例
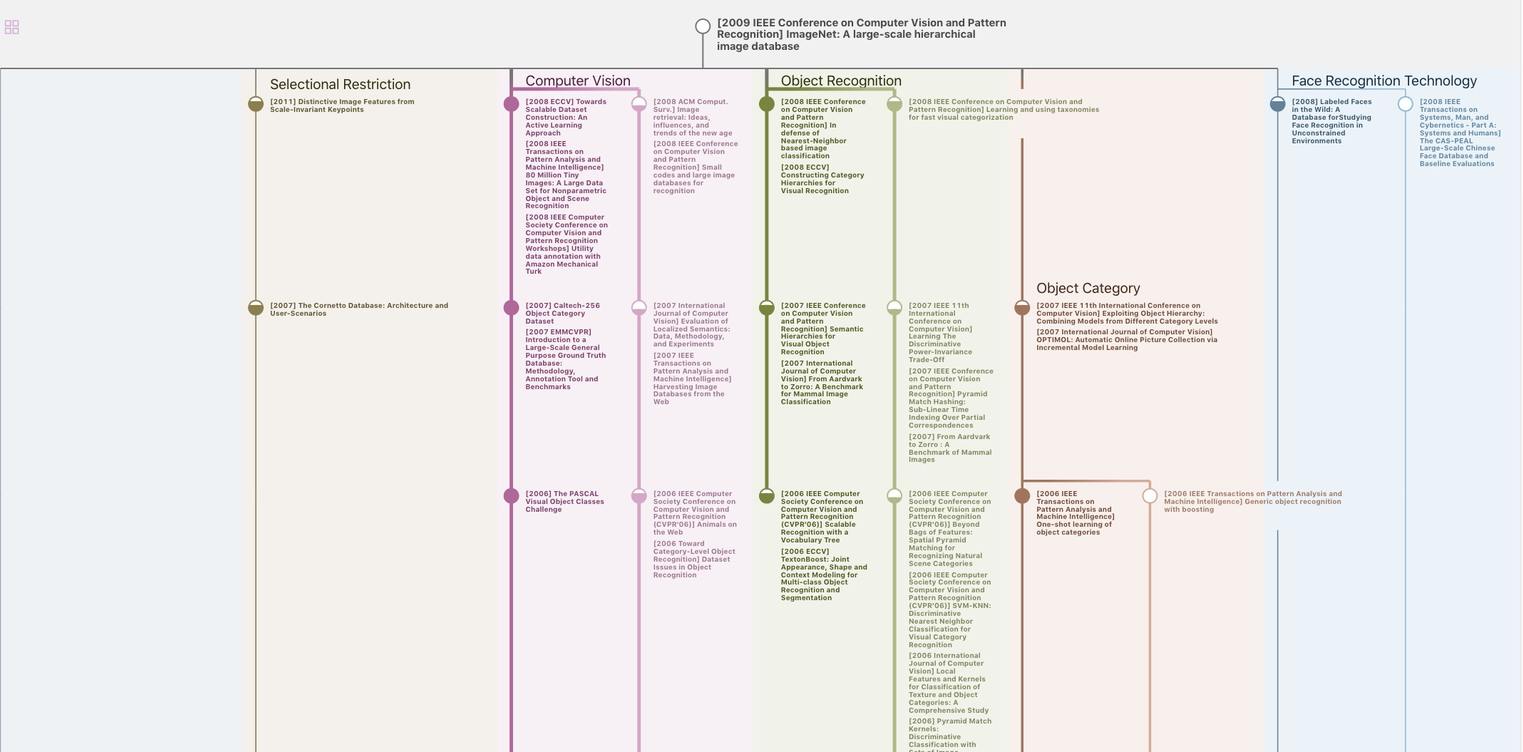
生成溯源树,研究论文发展脉络
Chat Paper
正在生成论文摘要