Informational feedback accelerates learning in multi-alternative perceptual judgements of orientation.
Vision research(2023)
摘要
Experience or training can substantially improve perceptual performance through perceptual learning, and the extent and rate of these improvements may be affected by feedback. In this paper, we first developed a neural network model based on the integrated reweighting theory (Dosher et al., 2013) to account for perceptual learning and performance in n-alternative identification tasks and the dependence of learning on different forms of feedback. We then report an experiment comparing the effectiveness of response feedback (RF) versus accuracy feedback (AF) or no feedback (NF) (full versus partial versus no supervision) in learning a challenging eight-alternative visual orientation identification (8AFC) task. Although learning sometimes occurred in the absence of feedback (NF), RF had a clear advantage above AF or NF in this task. Using hybrid supervision learning rules, a new n-alternative identification integrated reweighting theory (I-IRT) explained both the differences in learning curves given different feedback and the dynamic changes in identification confusion data. This study shows that training with more informational feedback (RF) is more effective, though not necessary, in these challenging n-alternative tasks, a result that has implications for developing training paradigms in realistic tasks.
更多查看译文
关键词
informational feedback,orientation,learning,multi-alternative
AI 理解论文
溯源树
样例
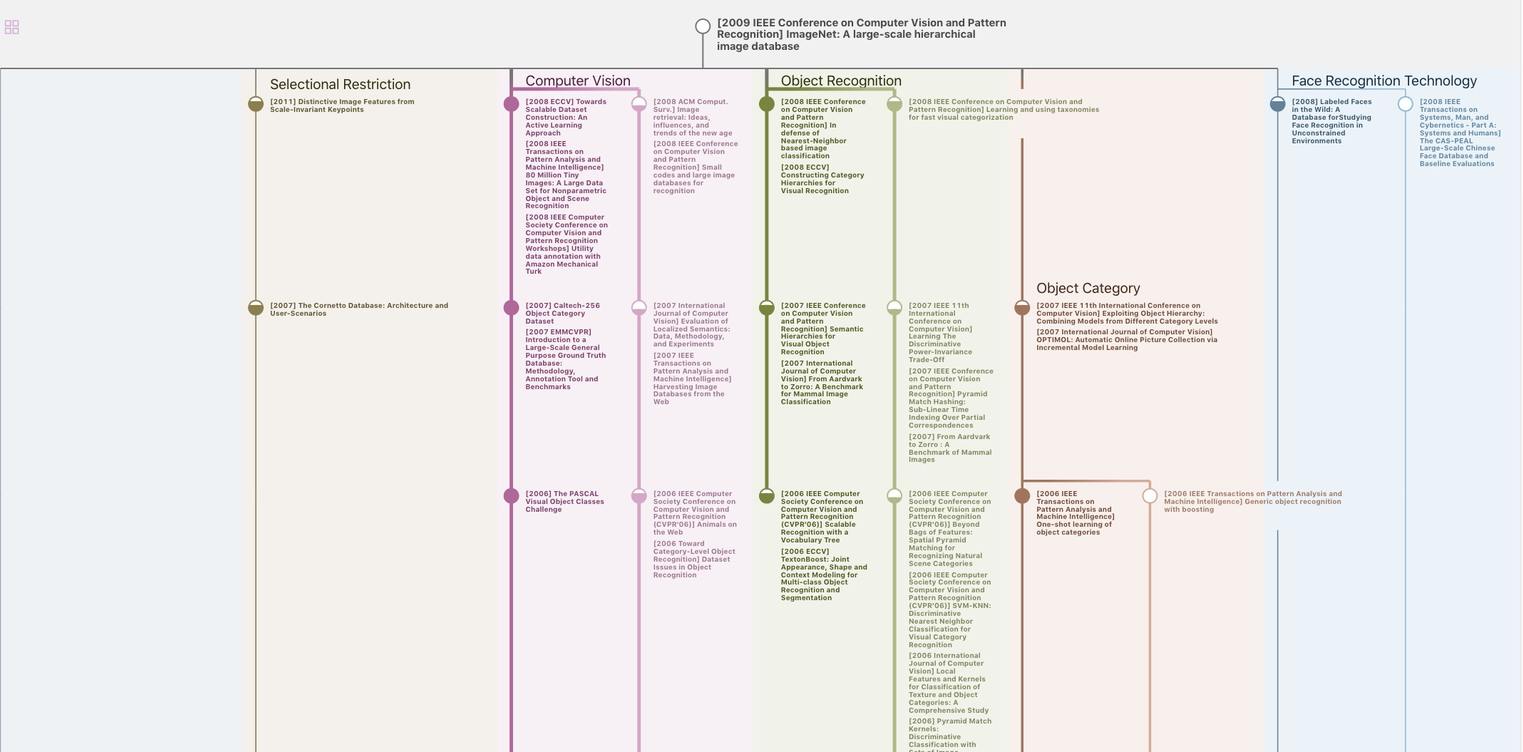
生成溯源树,研究论文发展脉络
Chat Paper
正在生成论文摘要