(Predictable) performance bias in unsupervised anomaly detection
EBIOMEDICINE(2024)
摘要
Background With the ever-increasing amount of medical imaging data, the demand for algorithms to assist clinicians has amplified. Unsupervised anomaly detection (UAD) models promise to aid in the crucial first step of disease detection. While previous studies have thoroughly explored fairness in supervised models in healthcare, for UAD, this has so far been unexplored. Methods In this study, we evaluated how dataset composition regarding subgroups manifests in disparate performance of UAD models along multiple protected variables on three large-scale publicly available chest X-ray datasets. Our experiments were validated using two state-of-the-art UAD models for medical images. Finally, we introduced subgroup-AUROC (sAUROC), which aids in quantifying fairness in machine learning. Findings Our experiments revealed empirical "fairness laws" (similar to "scaling laws" for Transformers) for trainingdataset composition: Linear relationships between anomaly detection performance within a subpopulation and its representation in the training data. Our study further revealed performance disparities, even in the case of balanced training data, and compound effects that exacerbate the drop in performance for subjects associated with multiple adversely affected groups. Interpretation Our study quantified the disparate performance of UAD models against certain demographic subgroups. Importantly, we showed that this unfairness cannot be mitigated by balanced representation alone. Instead, the representation of some subgroups seems harder to learn by UAD models than that of others. The empirical "fairness laws" discovered in our study make disparate performance in UAD models easier to estimate and aid in determining the most desirable dataset composition. Funding European Research Council Deep4MI. Copyright (c) 2024 The Author(s). Published by Elsevier B.V. This is an open access article under the CC BY license (http://creativecommons.org/licenses/by/4.0/).
更多查看译文
关键词
Arti fi cial intelligence,Machine learning,Algorithmic bias,Subgroup disparities,Anomaly detection
AI 理解论文
溯源树
样例
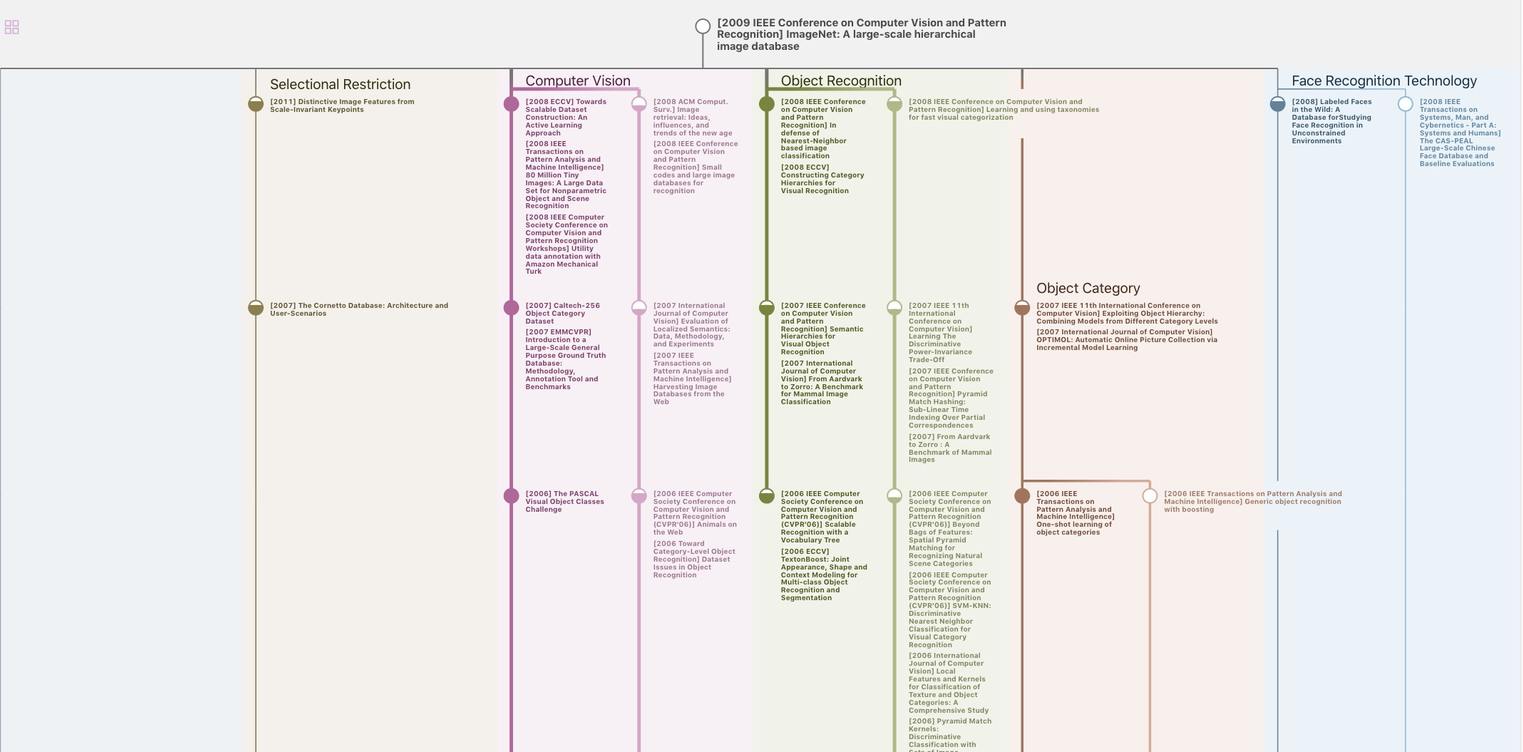
生成溯源树,研究论文发展脉络
Chat Paper
正在生成论文摘要