Fast-HuBERT: An Efficient Training Framework for Self-Supervised Speech Representation Learning
CoRR(2023)
摘要
Recent years have witnessed significant advancements in self-supervised learning (SSL) methods for speech-processing tasks. Various speech-based SSL models have been developed and present promising performance on a range of downstream tasks including speech recognition. However, existing speech-based SSL models face a common dilemma in terms of computational cost, which might hinder their potential application and in-depth academic research. To address this issue, we first analyze the computational cost of different modules during HuBERT pre-training and then introduce a stack of efficiency optimizations, which is named Fast-HuBERT in this paper. The proposed Fast-HuBERT can be trained in 1.1 days with 8 V100 GPUs on the Librispeech 960h benchmark, without performance degradation, resulting in a 5.2x speedup, compared to the original implementation. Moreover, we explore two well-studied techniques in the Fast-HuBERT and demonstrate consistent improvements as reported in previous work.
更多查看译文
关键词
Speech recognition,Self-supervised learning,Efficiency optimization
AI 理解论文
溯源树
样例
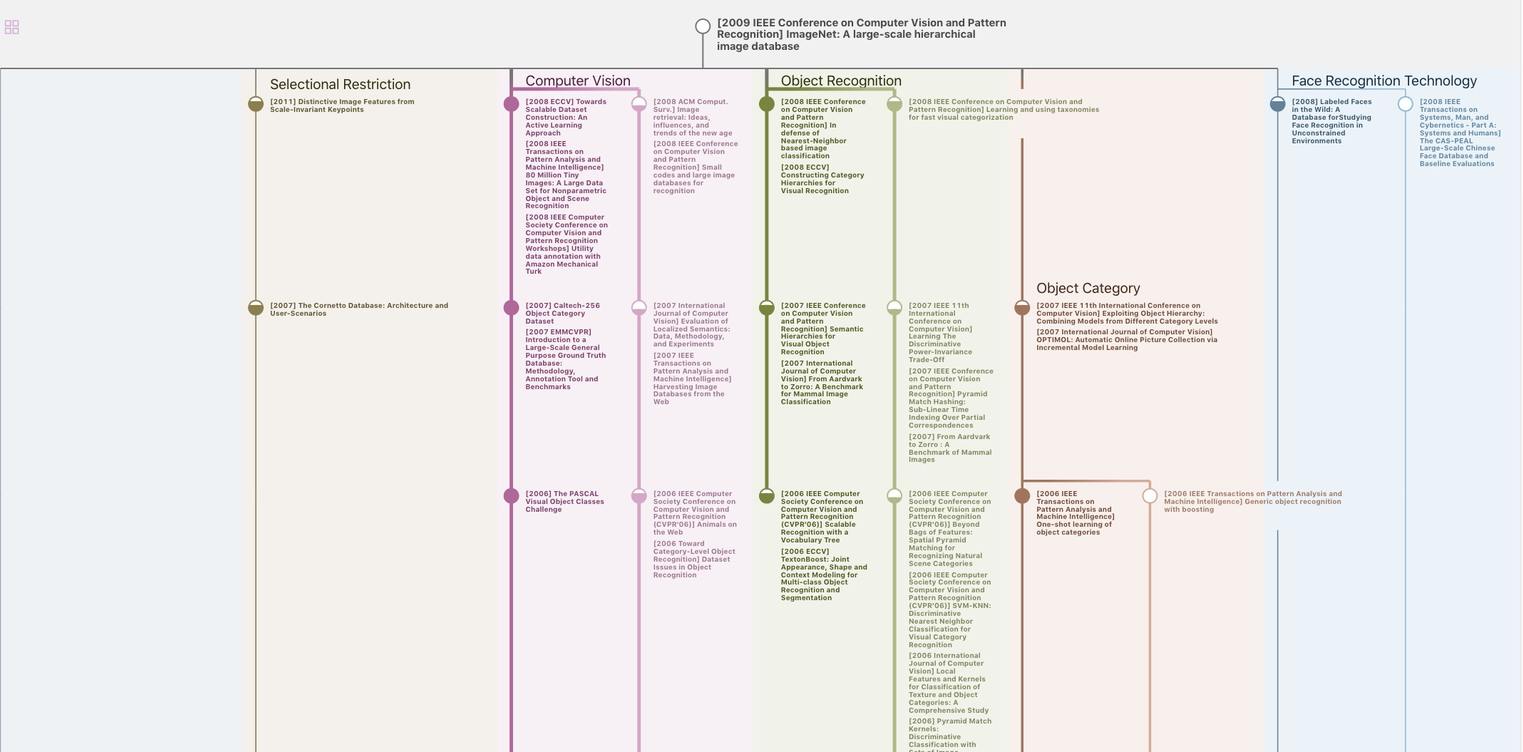
生成溯源树,研究论文发展脉络
Chat Paper
正在生成论文摘要