Boosting Offline Reinforcement Learning for Autonomous Driving with Hierarchical Latent Skills
ICRA 2024(2024)
摘要
Vehicle planning is receiving increasing attention with the emergence of diverse driving simulators and large-scale driving datasets. While offline reinforcement learning (RL) is well suited for these safety-critical tasks, it still struggles to plan over extended periods. In this work, we present a skill-based framework that enhances offline RL to overcome the long-horizon vehicle planning challenge. Specifically, we design a variational autoencoder (VAE) to learn skills from offline demonstrations. To mitigate the posterior collapse problem, we introduce a two-branch sequence encoder to capture both discrete options and continuous variations of the complex driving skills. The final policy treats learned skills as actions and can be trained by any off-the-shelf offline RL algorithms. This facilitates a shift in focus from per-step actions to temporally extended skills, thereby enabling long-term reasoning into the future. Extensive results on CARLA prove that our model consistently outperforms strong baselines at both training and new scenarios. Additional visualizations and experiments demonstrate the interpretability and transferability of extracted skills.
更多查看译文
关键词
Autonomous Vehicle Navigation,Reinforcement Learning,Integrated Planning and Learning
AI 理解论文
溯源树
样例
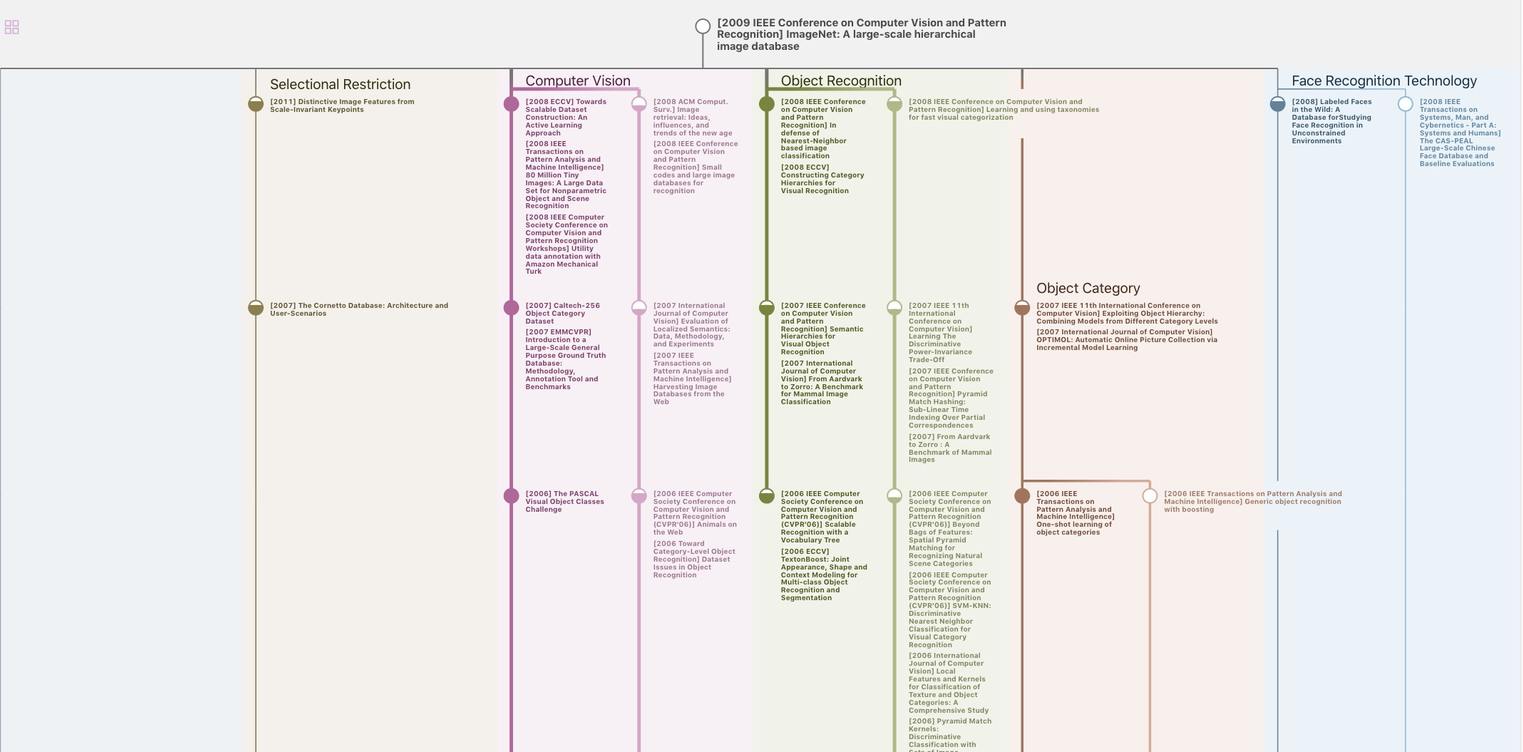
生成溯源树,研究论文发展脉络
Chat Paper
正在生成论文摘要