Anytime valid and asymptotically optimal statistical inference driven by predictive recursion
arXiv (Cornell University)(2023)
摘要
Distinguishing two classes of candidate models is a fundamental and practically important problem in statistical inference. Error rate control is crucial to the logic but, in complex nonparametric settings, such guarantees can be difficult to achieve, especially when the stopping rule that determines the data collection process is not available. In this paper we develop a novel e-value construction that leverages the so-called predictive recursion (PR) algorithm designed to recursively fit nonparametric mixture models. The resulting PRe-value affords anytime valid inference uniformly over stopping rules and is shown to be efficient in the sense that it achieves the maximal growth rate under the alternative relative to the mixture model being fit by PR. In the special case of testing the density for log-concavity, the PRe-value test is shown empirically to be significantly more efficient than a recently proposed anytime valid test based on universal inference.
更多查看译文
关键词
optimal statistical inference
AI 理解论文
溯源树
样例
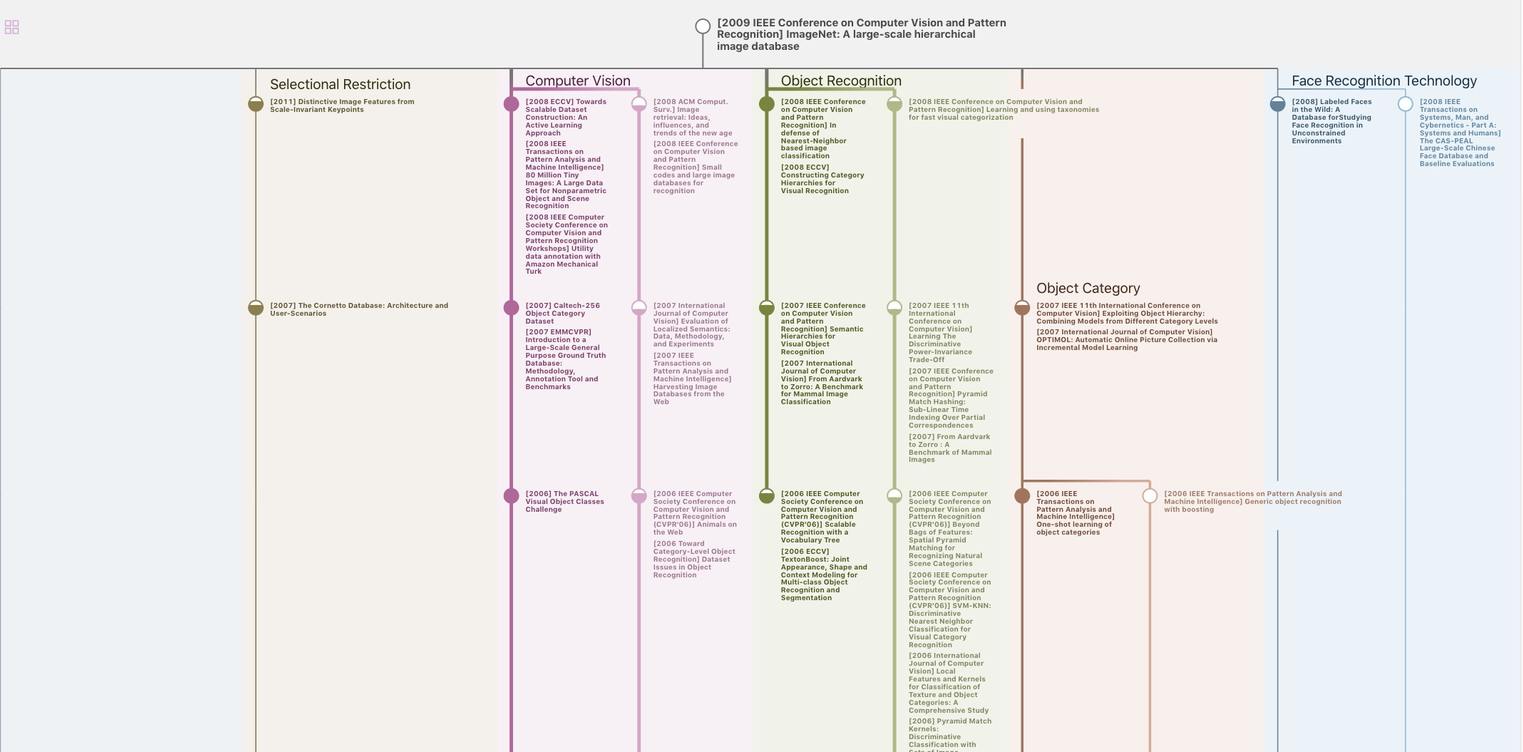
生成溯源树,研究论文发展脉络
Chat Paper
正在生成论文摘要