A closer look at parameter identifiability, model selection and handling of censored data with Bayesian Inference in mathematical models of tumour growth
arXiv (Cornell University)(2023)
Abstract
Mathematical models (MMs) are a powerful tool to help us understand and predict the dynamics of tumour growth under various conditions. In this work, we use 5 MMs with an increasing number of parameters to explore how certain (often overlooked) decisions in estimating parameters from data of experimental tumour growth affect the outcome of the analysis. In particular, we propose a framework for including tumour volume measurements that fall outside the upper and lower limits of detection, which are normally discarded. We demonstrate how excluding censored data results in an overestimation of the initial tumour volume and the MM-predicted tumour volumes prior to the first measurements, and an underestimation of the carrying capacity and the MM-predicted tumour volumes beyond the latest measurable time points. We show in which way the choice of prior for the MM parameters can impact the posterior distributions, and illustrate that reporting the highest-likelihood parameters and their 95% credible interval can lead to confusing or misleading interpretations. We hope this work will encourage others to carefully consider choices made in parameter estimation and to adopt the approaches we put forward herein.
MoreTranslated text
Key words
bayesian inference,parameter identifiability,model selection,mathematical models
AI Read Science
Must-Reading Tree
Example
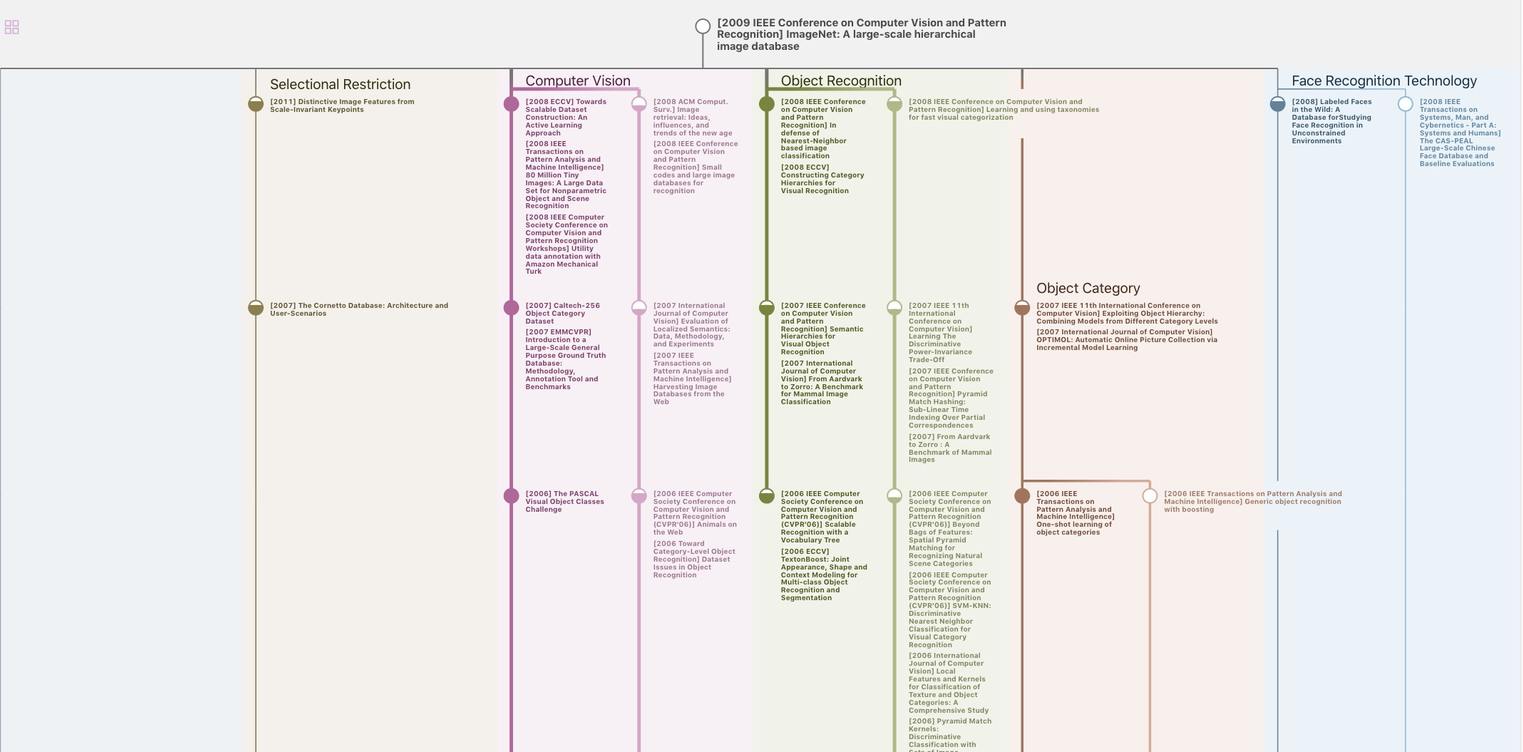
Generate MRT to find the research sequence of this paper
Chat Paper
Summary is being generated by the instructions you defined