RTrack: Accelerating Convergence for Visual Object Tracking via Pseudo-Boxes Exploration
CoRR(2023)
摘要
Single object tracking (SOT) heavily relies on the representation of the target object as a bounding box. However, due to the potential deformation and rotation experienced by the tracked targets, the genuine bounding box fails to capture the appearance information explicitly and introduces cluttered background. This paper proposes RTrack, a novel object representation baseline tracker that utilizes a set of sample points to get a pseudo bounding box. RTrack automatically arranges these points to define the spatial extents and highlight local areas. Building upon the baseline, we conducted an in-depth exploration of the training potential and introduced a one-to-many leading assignment strategy. It is worth noting that our approach achieves competitive performance to the state-of-the-art trackers on the GOT-10k dataset while reducing training time to just 10% of the previous state-of-the-art (SOTA) trackers' training costs. The substantial reduction in training costs brings single-object tracking (SOT) closer to the object detection (OD) task. Extensive experiments demonstrate that our proposed RTrack achieves SOTA results with faster convergence.
更多查看译文
关键词
visual object tracking,pseudo-boxes
AI 理解论文
溯源树
样例
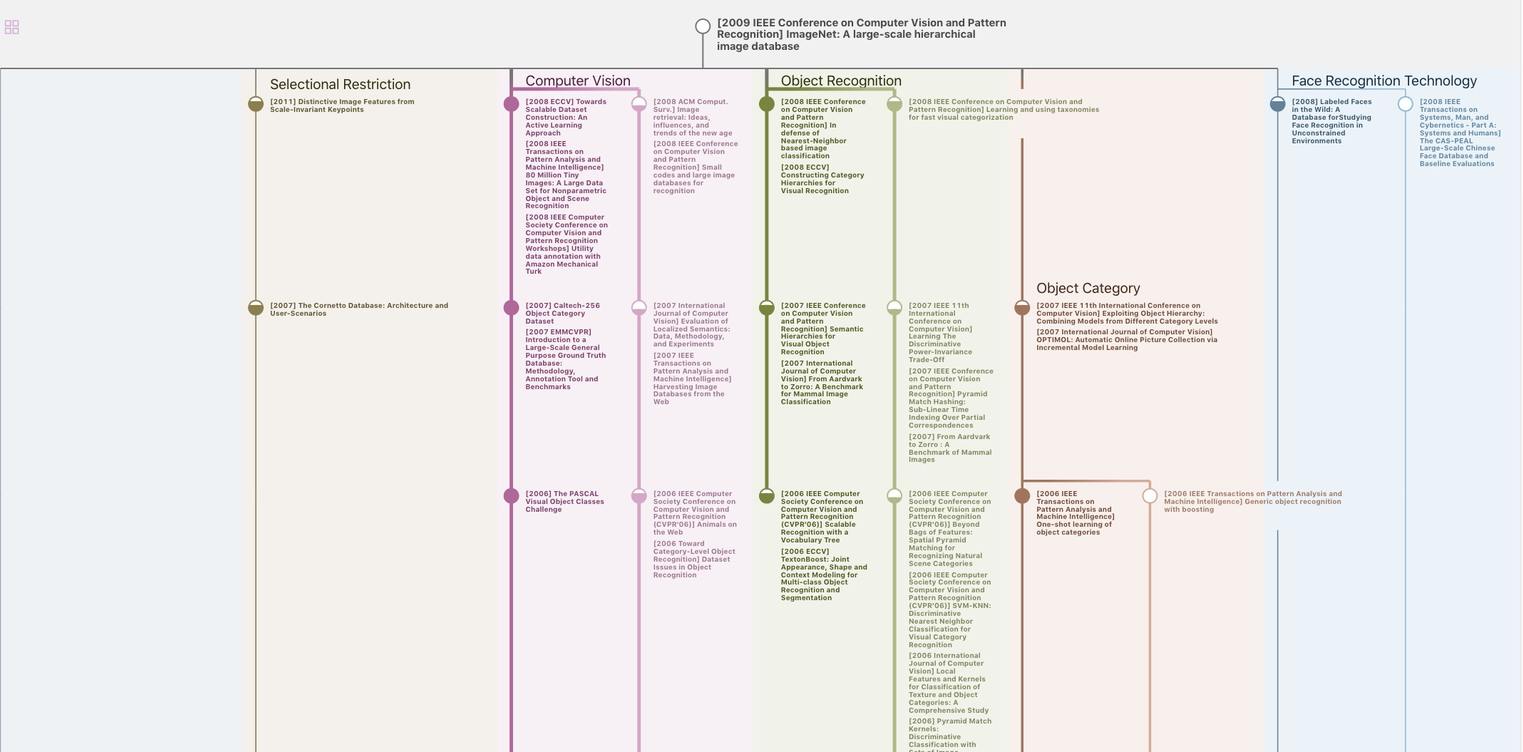
生成溯源树,研究论文发展脉络
Chat Paper
正在生成论文摘要