Federated Short-Term Load Forecasting with Personalization Layers for Heterogeneous Clients
CoRR(2023)
摘要
The advent of smart meters has enabled pervasive collection of energy consumption data for training short-term load forecasting (STLF) models. In response to privacy concerns, federated learning (FL) has been proposed as a privacy-preserving approach for training, but the quality of trained models degrades as client data becomes heterogeneous. In this paper we alleviate this drawback using personalization layers, wherein certain layers of an STLF model in an FL framework are trained exclusively on the clients' own data. To that end, we propose a personalized FL algorithm (PL-FL) enabling FL to handle personalization layers. The PL-FL algorithm is implemented by using the Argonne Privacy-Preserving Federated Learning package. We test the forecast performance of models trained on the NREL ComStock dataset, which contains heterogeneous energy consumption data of multiple commercial buildings. Superior performance of models trained with PL-FL demonstrates that personalization layers enable classical FL algorithms to handle clients with heterogeneous data.
更多查看译文
关键词
heterogeneous clients,personalization layers,load,short-term
AI 理解论文
溯源树
样例
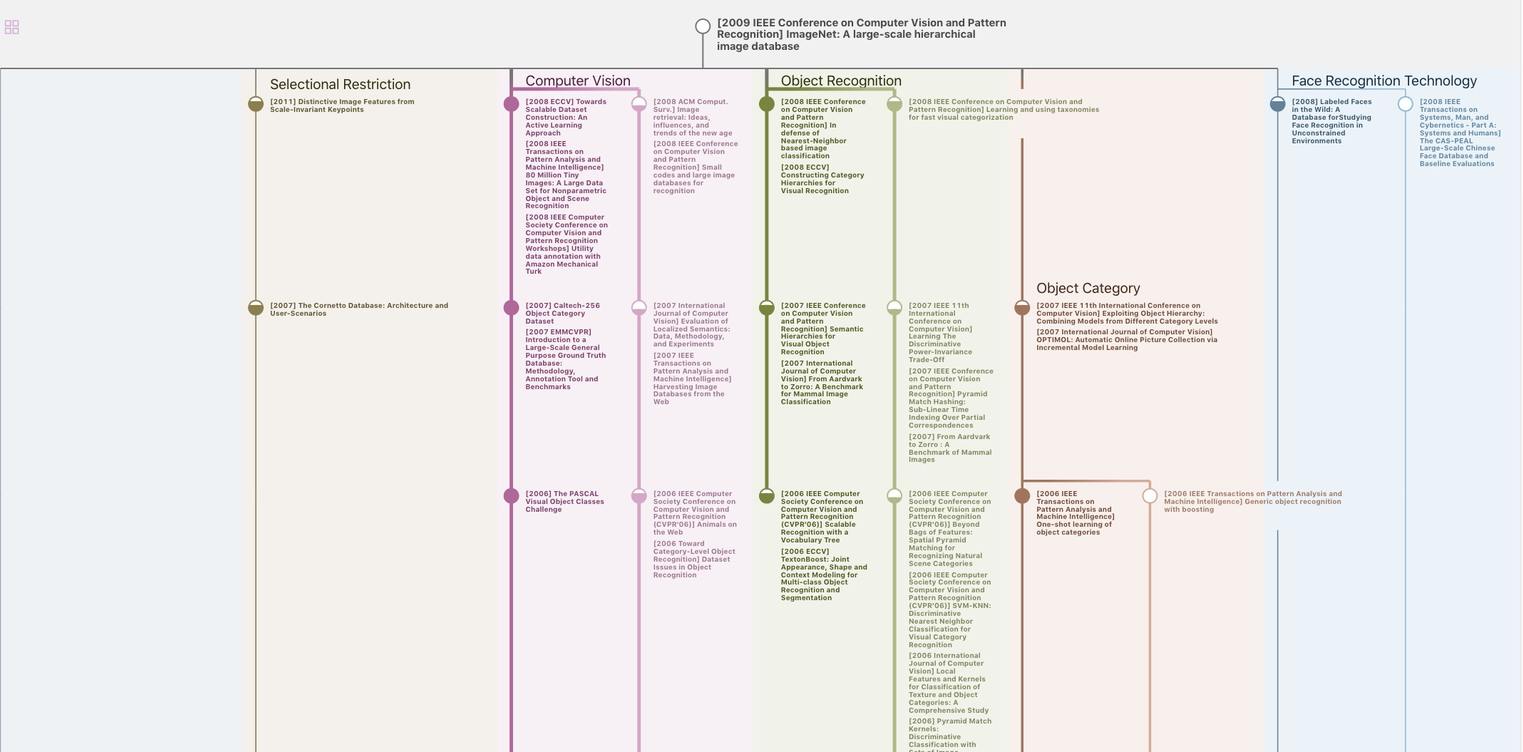
生成溯源树,研究论文发展脉络
Chat Paper
正在生成论文摘要