Forecasting Response to Treatment with Global Deep Learning and Patient-Specific Pharmacokinetic Priors.
CoRR(2024)
摘要
Forecasting healthcare time series is crucial for early detection of adverse outcomes and for patient monitoring. Forecasting, however, can be difficult in practice due to noisy and intermittent data. The challenges are often exacerbated by change points induced via extrinsic factors, such as the administration of medication. To address these challenges, we propose a novel hybrid global-local architecture and a pharmacokinetic encoder that informs deep learning models of patient-specific treatment effects. We showcase the efficacy of our approach in achieving significant accuracy gains for a blood glucose forecasting task using both realistically simulated and real-world data. Our global-local architecture improves over patient-specific models by 9.2-14.6%. Additionally, our pharmacokinetic encoder improves over alternative encoding techniques by 4.4% on simulated data and 2.1% on real-world data. The proposed approach can have multiple beneficial applications in clinical practice, such as issuing early warnings about unexpected treatment responses, or helping to characterize patient-specific treatment effects in terms of drug absorption and elimination characteristics.
更多查看译文
关键词
pharmacokinetic
AI 理解论文
溯源树
样例
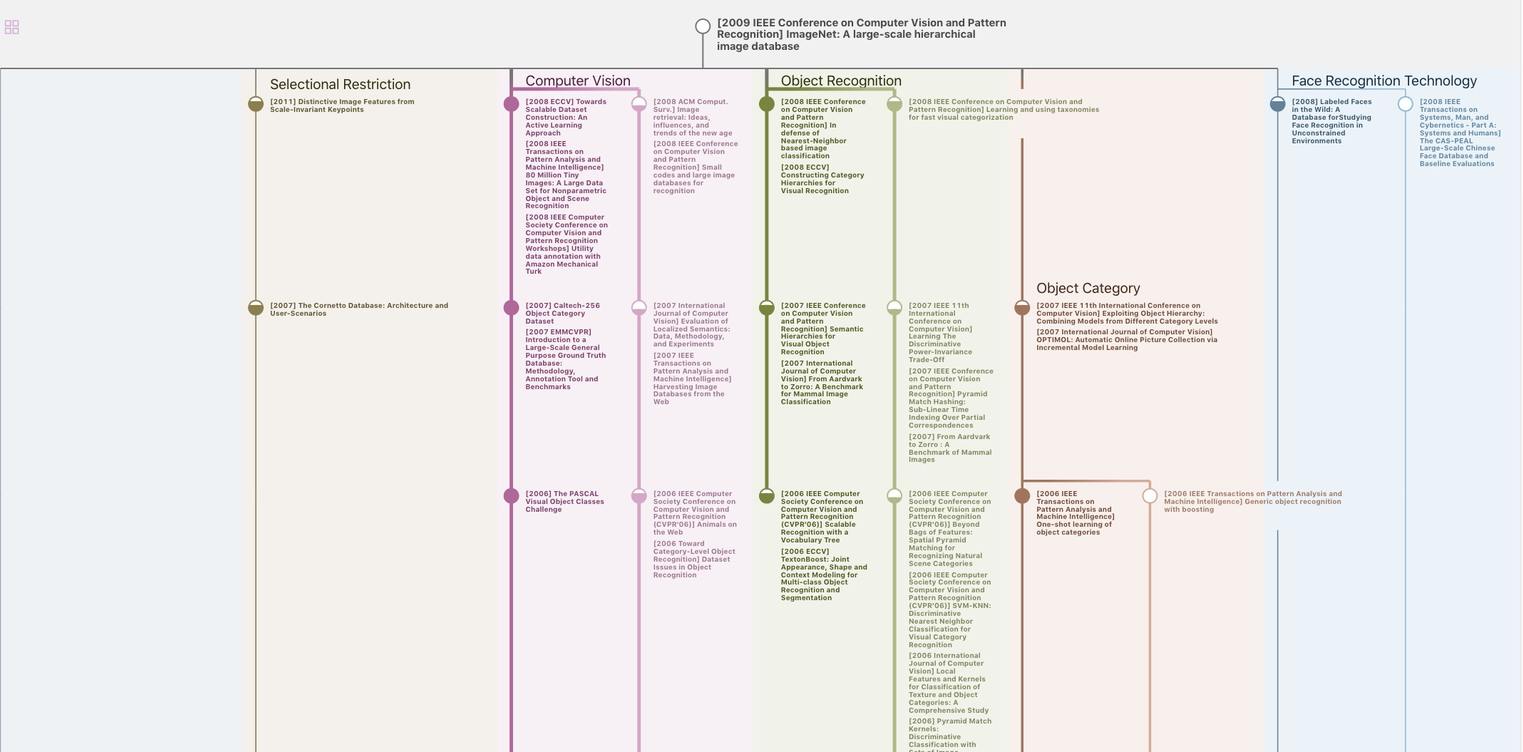
生成溯源树,研究论文发展脉络
Chat Paper
正在生成论文摘要