Learning to Coordinate with Anyone
2023 5TH INTERNATIONAL CONFERENCE ON DISTRIBUTED ARTIFICIAL INTELLIGENCE, DAI 2023(2023)
摘要
In open multi-agent environments, the agents may encounter unexpected teammates. Classical multi-agent learning approaches train agents that can only coordinate with seen teammates. Recent studies attempted to generate diverse teammates in order to enhance the generalizable coordination ability, but were restricted by pre-defined teammates. In this work, our aim is to train agents with strong coordination ability by generating teammates that fully cover the teammate policy space, so that agents can coordinate with any teammates. Since the teammate policy space is too huge to be enumerated, we find only dissimilar teammates that are incompatible with controllable agents, which highly reduces the number of teammates that needed to be trained with. However, it is hard to determine the number of such incompatible teammates beforehand. We therefore introduce a continual multi-agent learning process, in which the agent learns to coordinate with different teammates until no more incompatible teammates can be found. The above idea is implemented in the proposed Macop (Multi-agent compatible policy learning) algorithm. We conduct experiments in 8 scenarios from 4 environments that have distinct coordination patterns. Experiments show that Macop generates training teammates with much lower compatibility than previous methods. As a result, in all scenarios Macop achieves the best overall coordination ability while never significantly worse than the baselines, showing strong generalization ability.
更多查看译文
关键词
Multi-agent System,Coordination and Cooperation,Continual Learning,Reinforcement Learning
AI 理解论文
溯源树
样例
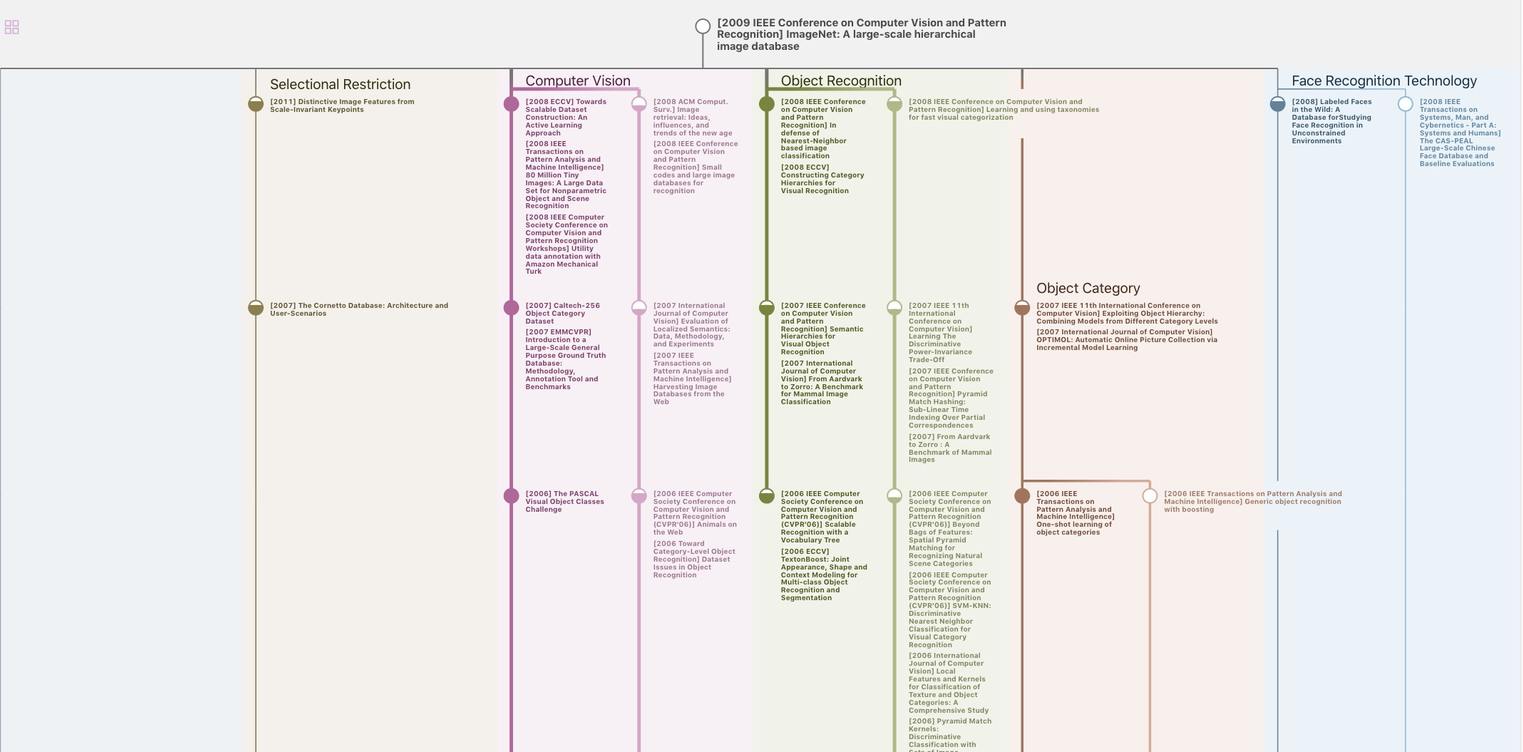
生成溯源树,研究论文发展脉络
Chat Paper
正在生成论文摘要