State2Explanation: Concept-Based Explanations to Benefit Agent Learning and User Understanding
NeurIPS(2023)
Abstract
With more complex AI systems used by non-AI experts to complete daily tasks, there is an increasing effort to develop methods that produce explanations of AI decision making understandable by non-AI experts. Towards this effort, leveraging higher-level concepts and producing concept-based explanations have become a popular method. Most concept-based explanations have been developed for classification techniques, and we posit that the few existing methods for sequential decision making are limited in scope. In this work, we first contribute a desiderata for defining "concepts" in sequential decision making settings. Additionally, inspired by the Protege Effect which states explaining knowledge often reinforces one's self-learning, we explore the utility of concept-based explanations providing a dual benefit to the RL agent by improving agent learning rate, and to the end-user by improving end-user understanding of agent decision making. To this end, we contribute a unified framework, State2Explanation (S2E), that involves learning a joint embedding model between state-action pairs and concept-based explanations, and leveraging such learned model to both (1) inform reward shaping during an agent's training, and (2) provide explanations to end-users at deployment for improved task performance. Our experimental validations, in Connect 4 and Lunar Lander, demonstrate the success of S2E in providing a dual-benefit, successfully informing reward shaping and improving agent learning rate, as well as significantly improving end user task performance at deployment time.
MoreTranslated text
Key words
agent learning
AI Read Science
Must-Reading Tree
Example
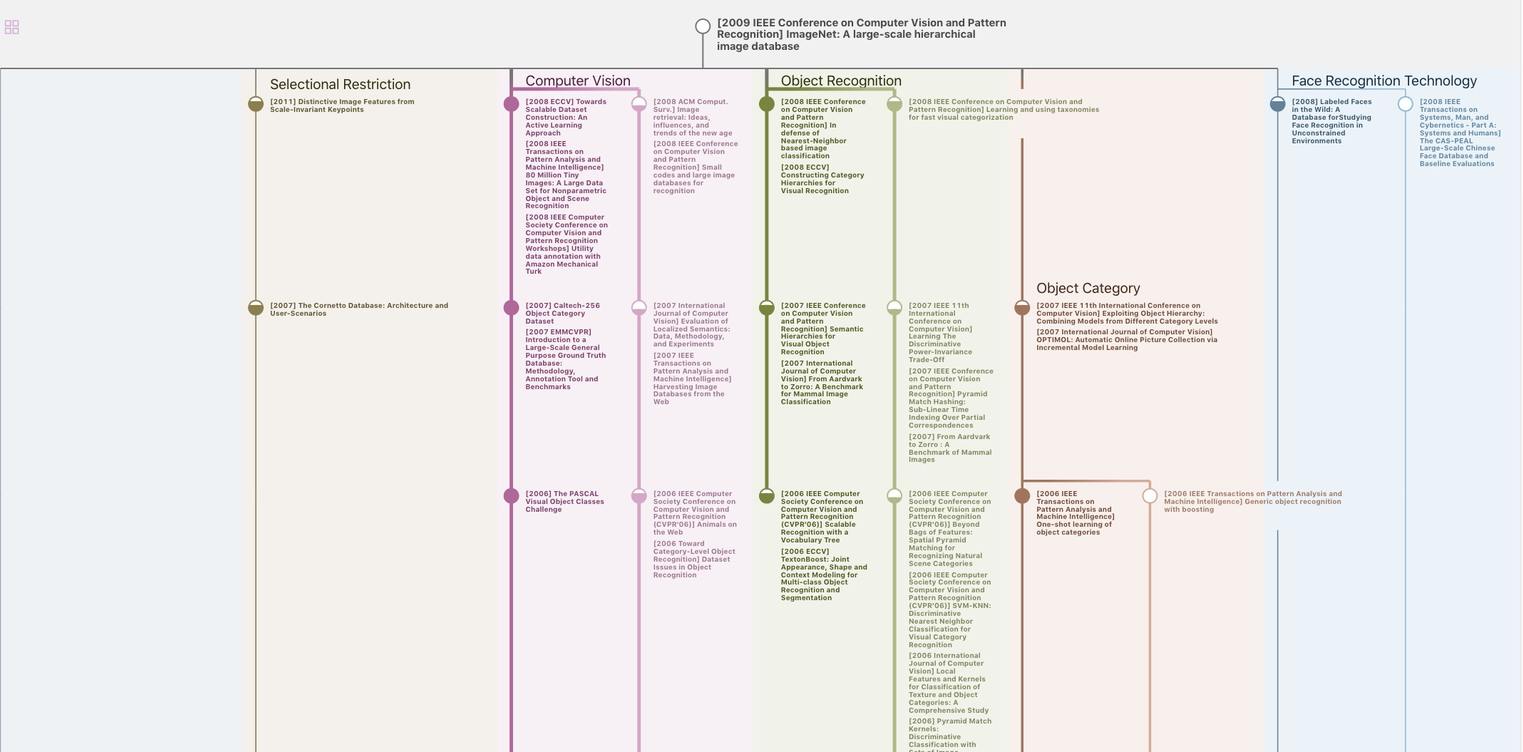
Generate MRT to find the research sequence of this paper
Chat Paper
Summary is being generated by the instructions you defined