Evolvable transformation of knowledge graphs into human-oriented formats
JOURNAL OF INTELLIGENT INFORMATION SYSTEMS(2023)
摘要
Along with the ongoing digitalization of society, we witness a strong movement to make scientific data FAIR, machine-actionable, and available in the form of knowledge graphs. On the other hand, converting machine-actionable data from knowledge graphs back into human-oriented formats, including documents, graphical, or voice user interfaces, poses significant challenges. The solutions often build on various templates tailored to specific platforms on top of the shared underlying data. These templates suffer from limited reusability, making their adaptations difficult. Moreover, the continuous evolution of data or technological advancements requires substantial efforts to maintain these templates over time. In general, these challenges increase software development costs and are error-prone. In this paper, we propose a solution based on Normalized Systems Theory to address this challenge with the aim of achieving evolvability and sustainability in the transformation process of knowledge graphs into human-oriented formats with broad applicability across domains and technologies. We explain the theoretical foundation and design theorems used in our solution and outline the approach and implementation details. We theoretically evaluate our solution by comparing it to the traditional approach, where the systems are crafted manually. The evaluation shows that our solution is more efficient and effective on a large scale, reducing the human labor required to maintain various templates and supported target platforms. Next, we demonstrate the technical feasibility of our solution on a proof-of-concept implementation in a domain of data management planning that may also serve as a basis for future development.
更多查看译文
关键词
Knowledge graph,Evolvability,Ontology,Normalized systems theory
AI 理解论文
溯源树
样例
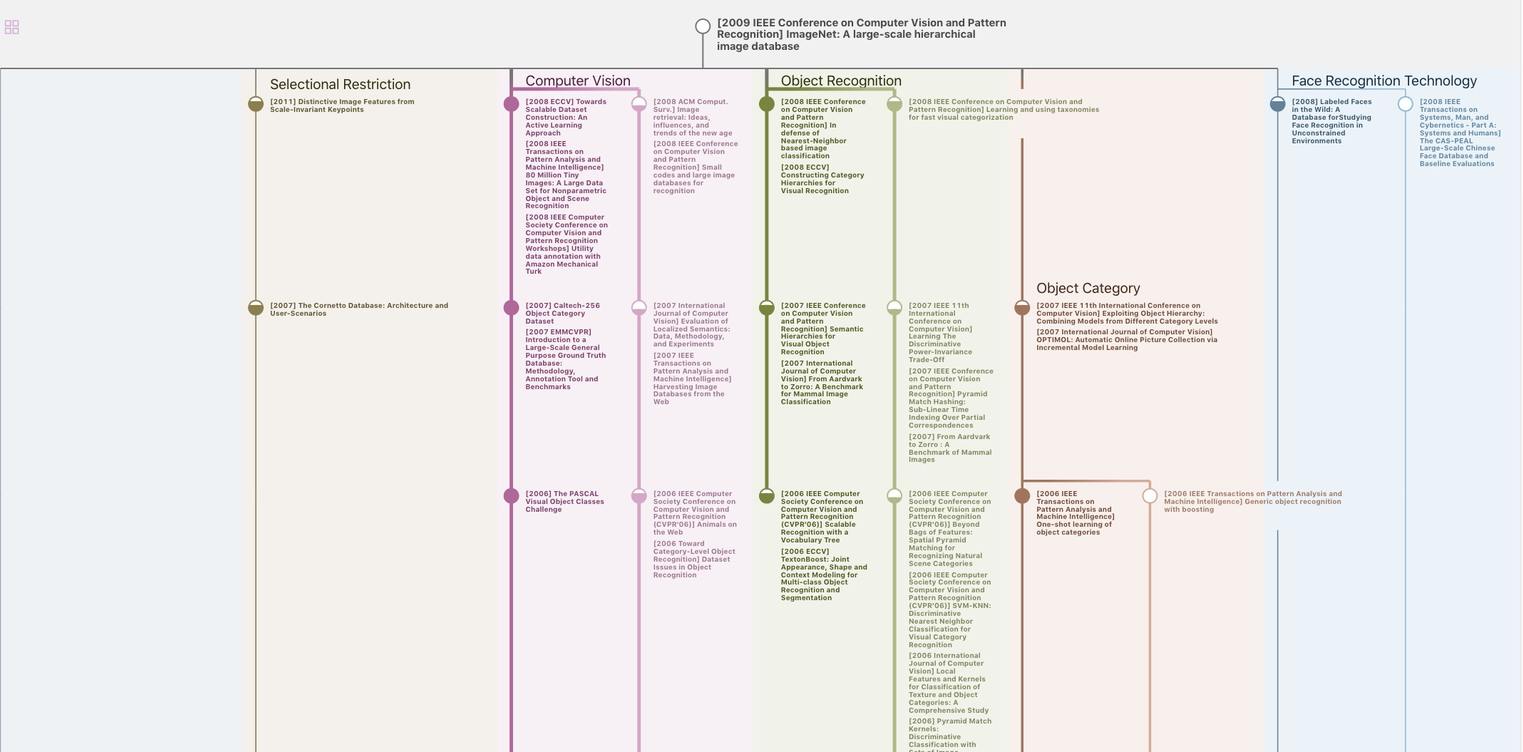
生成溯源树,研究论文发展脉络
Chat Paper
正在生成论文摘要