Word embeddings-based transfer learning for boosted relational dependency networks
Machine Learning(2024)
摘要
Conventional machine learning methods assume data to be independent and identically distributed (i.i.d.) and ignore the relational structure of the data, which contains crucial information about how objects participate in relationships and events. Statistical Relational Learning (SRL) combines elements from statistical and probabilistic modeling to relational learning to represent and learn in domains with complex relational and rich probabilistic structures. SRL models do not suppose data to be i.i.d., but, as conventional machine learning models, they also assume training and testing data are sampled from the same distribution. Transfer learning has emerged as an essential technique to handle scenarios where such an assumption does not hold. It aims to provide methods with the ability to recognize knowledge previously learned in a source domain and apply it to a new model in a target domain to start solving a new task. For SRL models, the primary challenge is to transfer the learned structure, mapping the vocabulary across different domains. In this work, we propose TransBoostler, an algorithm that uses pre-trained word embeddings to guide the mapping. We follow the assumption that the name of a predicate has a semantic connotation that can be mapped to a vector space model. Next, TransBoostler employs theory revision to adapt the mapped model to the target data. Experimental results showed that TransBoostler successfully transferred trees across different domains. It performs equally well as, or better than, previous systems and requires less training time for some investigated scenarios.
更多查看译文
关键词
Transfer learning,Statistical relational learning,Word embeddings,Relational domains
AI 理解论文
溯源树
样例
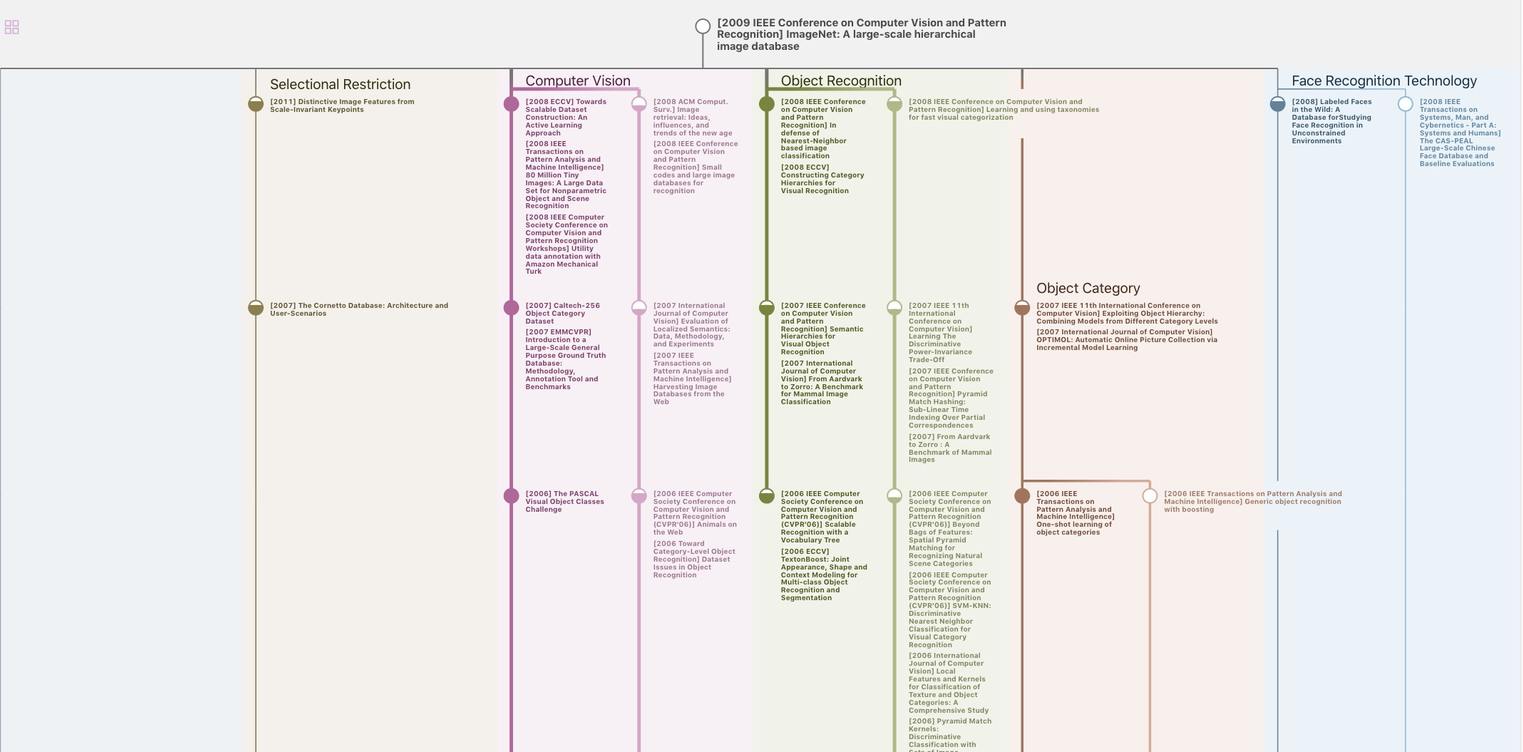
生成溯源树,研究论文发展脉络
Chat Paper
正在生成论文摘要