Deep learning for tumor margin identification in electromagnetic imaging
Scientific Reports(2023)
Abstract
In this work, a novel method for tumor margin identification in electromagnetic imaging is proposed to optimize the tumor removal surgery. This capability will enable the visualization of the border of the cancerous tissue for the surgeon prior or during the excision surgery. To this end, the border between the normal and tumor parts needs to be identified. Therefore, the images need to be segmented into tumor and normal areas. We propose a deep learning technique which divides the electromagnetic images into two regions: tumor and normal, with high accuracy. We formulate deep learning from a perspective relevant to electromagnetic image reconstruction. A recurrent auto-encoder network architecture (termed here DeepTMI) is presented. The effectiveness of the algorithm is demonstrated by segmenting the reconstructed images of an experimental tissue-mimicking phantom. The structure similarity measure (SSIM) and mean-square-error (MSE) average of normalized reconstructed results by the DeepTMI method are about 0.94 and 0.04 respectively, while that average obtained from the conventional backpropagation (BP) method can hardly overcome 0.35 and 0.41 respectively.
MoreTranslated text
Key words
Hardware and infrastructure,Image processing,Machine learning,Preclinical research,Translational research,Science,Humanities and Social Sciences,multidisciplinary
AI Read Science
Must-Reading Tree
Example
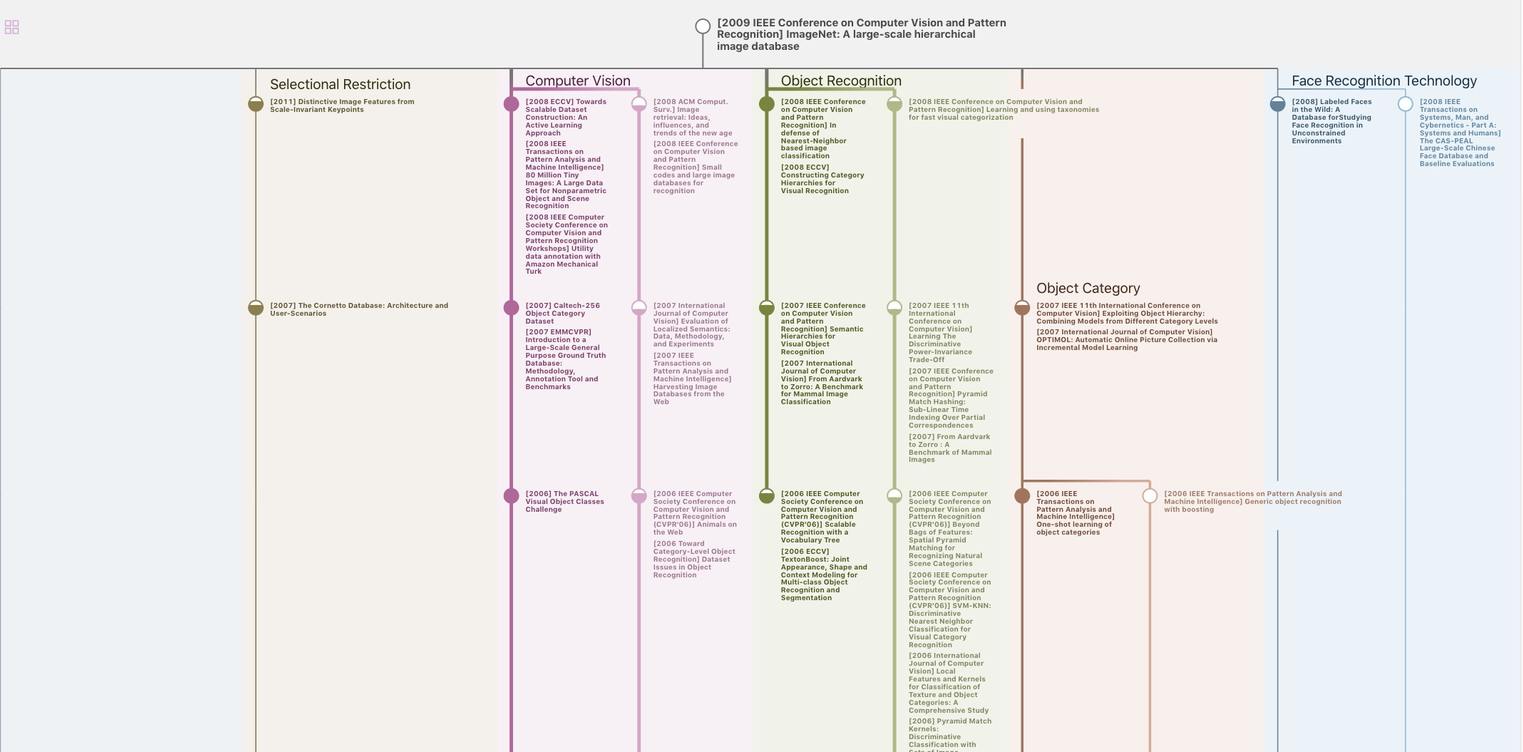
Generate MRT to find the research sequence of this paper
Chat Paper
Summary is being generated by the instructions you defined