Anomaly detection speed-up by quantum restricted Boltzmann machines
Communications Physics(2023)
摘要
Quantum machine learning promises to revolutionize traditional machine learning by efficiently addressing hard tasks for classical computation. While claims of quantum speed-up have been announced for gate-based quantum computers and photon-based boson samplers, demonstration of an advantage by adiabatic quantum annealers (AQAs) is open. Here we quantify the computational cost and the performance of restricted Boltzmann machines (RBMs), a widely investigated machine learning model, by classical and quantum annealing. Despite the lower computational complexity of the quantum RBM being lost due to physical implementation overheads, a quantum speed-up may arise as a reduction by orders of magnitude of the computational time. By employing real-world cybersecurity datasets, we observe that the negative phase on sufficiently challenging tasks is computed up to 64 times faster by AQAs during the exploitation phase. Therefore, although a quantum speed-up highly depends on the problem’s characteristics, it emerges in existing hardware on real-world data.
更多查看译文
关键词
Computational science,Quantum physics,Physics,general
AI 理解论文
溯源树
样例
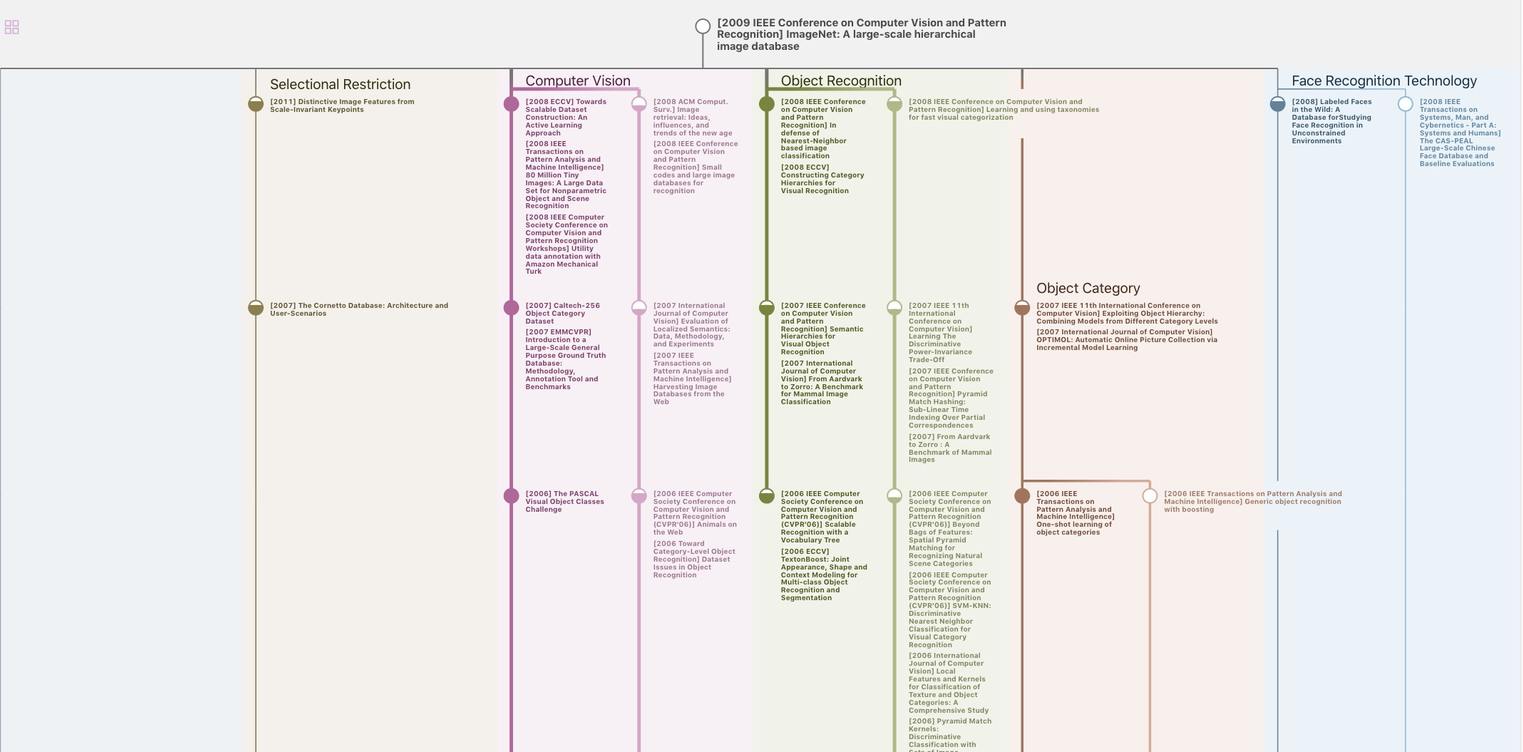
生成溯源树,研究论文发展脉络
Chat Paper
正在生成论文摘要