Unbiased curriculum learning enhanced global-local graph neural network for protein thermodynamic stability prediction
Bioinformatics (Oxford, England)(2023)
摘要
Motivation Proteins play crucial roles in biological processes, with their functions being closely tied to thermodynamic stability. However, measuring stability changes upon point mutations of amino acid residues using physical methods can be time-consuming. In recent years, several computational methods for protein thermodynamic stability prediction (PTSP) based on deep learning have emerged. Nevertheless, these approaches either overlook the natural topology of protein structures or neglect the inherent noisy samples resulting from theoretical calculation or experimental errors.Results We propose a novel Global-Local Graph Neural Network powered by Unbiased Curriculum Learning for the PTSP task. Our method first builds a Siamese graph neural network to extract protein features before and after mutation. Since the graph's topological changes stem from local node mutations, we design a local feature transformation module to make the model focus on the mutated site. To address model bias caused by noisy samples, which represent unavoidable errors from physical experiments, we introduce an unbiased curriculum learning method. This approach effectively identifies and re-weights noisy samples during the training process. Extensive experiments demonstrate that our proposed method outperforms advanced protein stability prediction methods, and surpasses state-of-the-art learning methods for regression prediction tasks.Availability and implementation All code and data is available at https://github.com/haifangong/UCL-GLGNN.
更多查看译文
关键词
protein thermodynamic stability prediction,curriculum,neural network,global-local
AI 理解论文
溯源树
样例
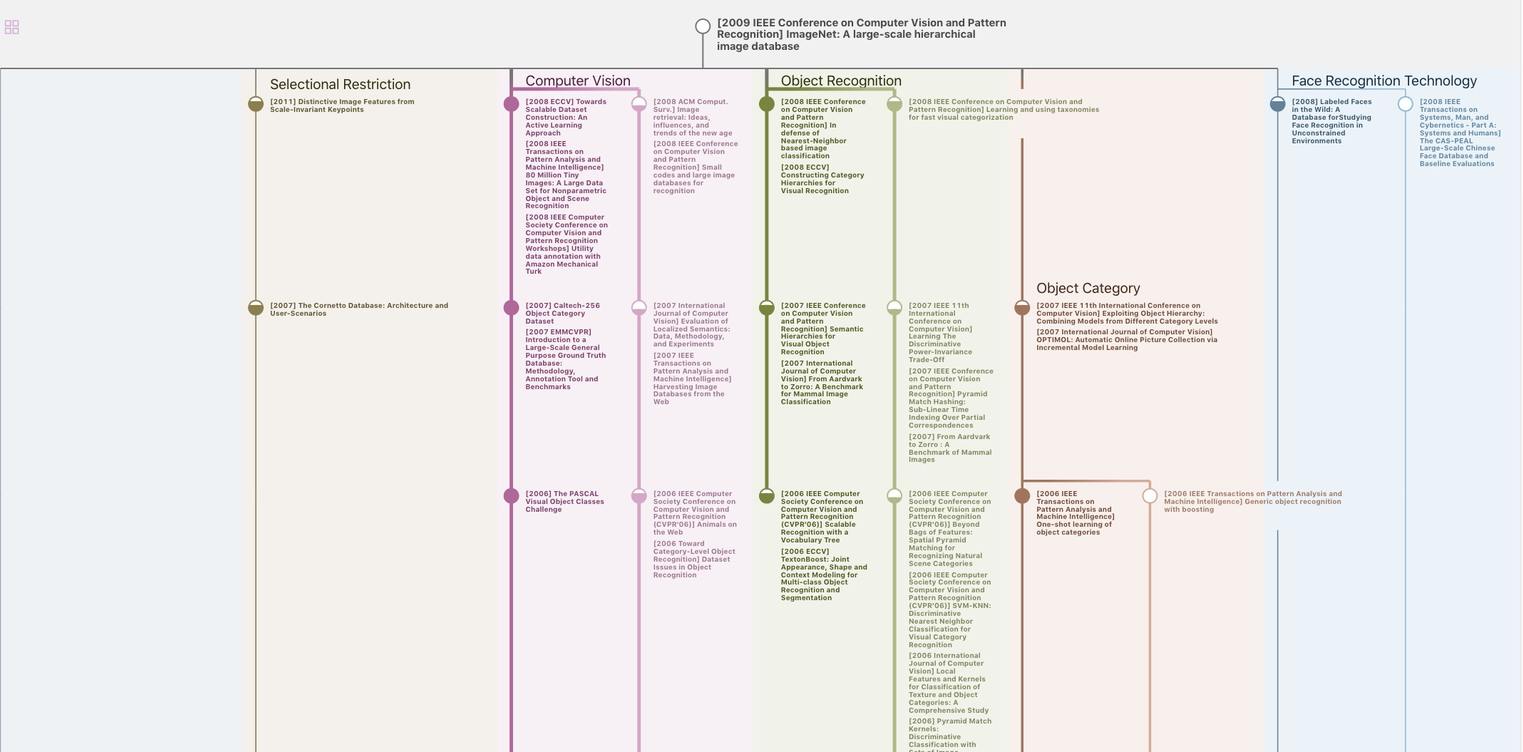
生成溯源树,研究论文发展脉络
Chat Paper
正在生成论文摘要