Analysis of Prospective Genetic Indicators for Prenatal Exposure to Arsenic in Newborn Cord Blood of Using Machine Learning
BIOLOGICAL TRACE ELEMENT RESEARCH(2024)
摘要
Using a machine learning methods, we aim to find biological effect biomarkers of prenatal arsenic exposure in newborn cord blood. From the Gene Expression Omnibus (GEO) database, two datasets (GSE48354 and GSE7967) pertaining to cord blood sequencing while exposed to arsenic were retrieved and merged for additional study. Using the "limma" package in the R, differentially expressed genes (DEGs) were eliminated. Machine learning techniques of the LASSO regression algorithm and SVM-RFE algorithm were used to find potential biological effect biomarkers for cord blood sequencing in pregnant women exposed to arsenic. To evaluate the efficacy of biomarkers, a receiver operating characteristic (ROC) curve was used. Furthermore, we investigated the proportion of invading immune cells in each sample using CIBERSORT, and we investigated the relationship between biomarkers and immune cells using the Spearman approach. Using LASSO regression and the SVM-RFE technique, 28 DEGs were discovered, and the main biomarkers of cord blood exposed to arsenic were discovered to be DENND2D, OLIG1, RGS18, CXCL16, DDIT4, FOS, G0S2, GPR183, JMJD6, and SOCS3. According to an immune infiltration analysis and correlation analysis, key biomarkers were substantially associated with the invading immune cells. Ten genes are important biomarkers of cord blood exposed to arsenic connected with infiltrating immune cells, and infiltrating immune cells may play important roles in cord blood exposed to arsenic, according to the study's findings.
更多查看译文
关键词
Arsenic,Machine learning,Biomarker,Prenatal exposure
AI 理解论文
溯源树
样例
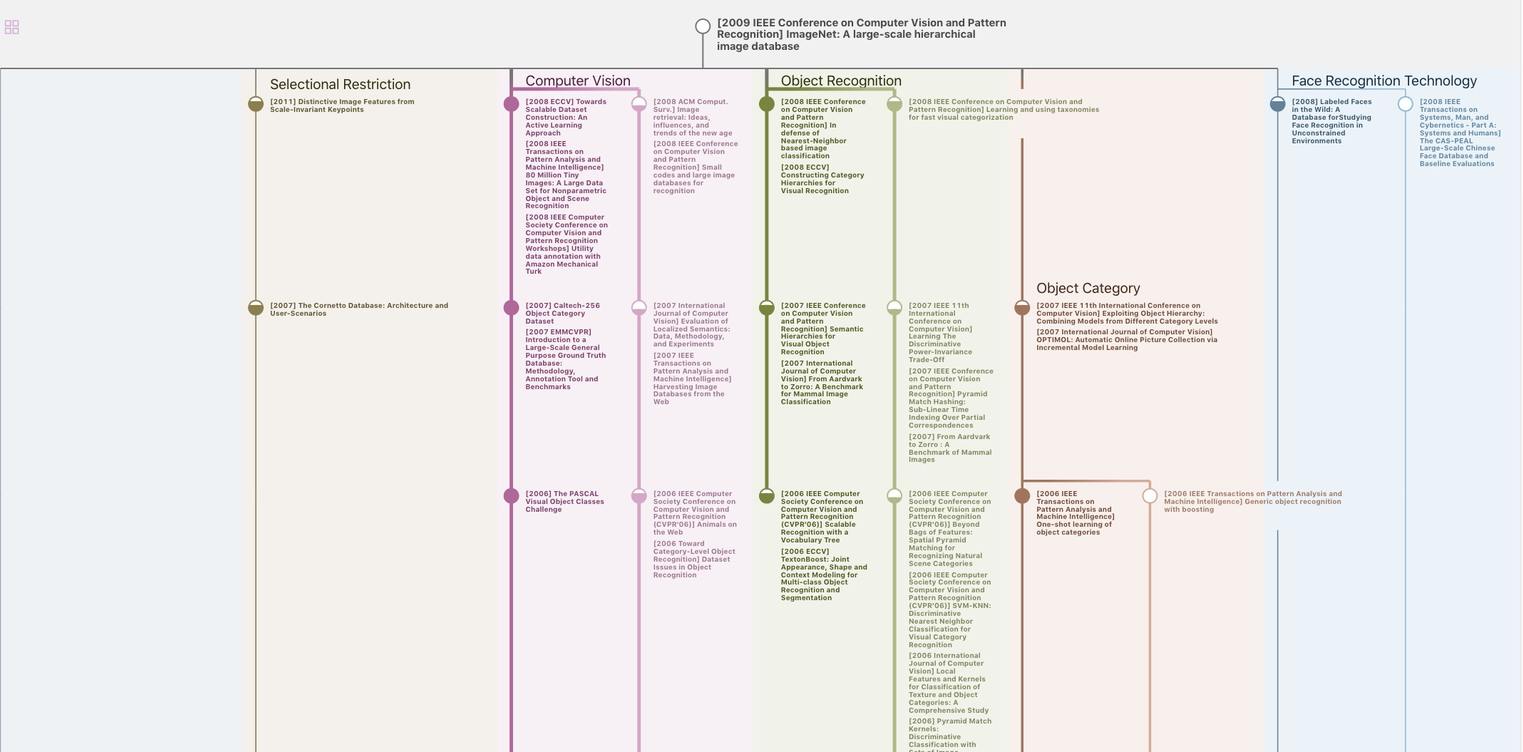
生成溯源树,研究论文发展脉络
Chat Paper
正在生成论文摘要