Attention-based Sound Classification Pipeline with Sound Spectrum
2023 IEEE Sensors Applications Symposium (SAS)(2023)
摘要
Urban soundscape research and their impact study are gaining more prominence with regard to a livable environment. Machine learning models have been used extensively to classify sounds where the input sound data, commonly in wave form, needs to be collected in its full frequency spectrum. However, in an application like NoiseCapture, the sound spectrum is divided into 23 frequency bands and thus some information or features are lost. Given the recent success in training a deep learning model to classify sounds with a limited sound spectrum, we developed a pipeline for maximizing the performance of sound spectrum input with attention-based model. Using data from ESC-50, we discover that the use of transformers improve accuracy over the conventional neural networks by 22.5%; however the limited frequency bands in NoiseCapture sound spectrum impairs the model accuracy, necessitating the use of data augmentation. The data pipeline is analyzed for our case study of Singapore, where selected sound labels, curated to fit the local context, are used to train the model, resulting in an improvement in base transformer accuracy by 12.7%.
更多查看译文
关键词
Sound Spectrum,Transformer,Sound Classification,Data Pipeline
AI 理解论文
溯源树
样例
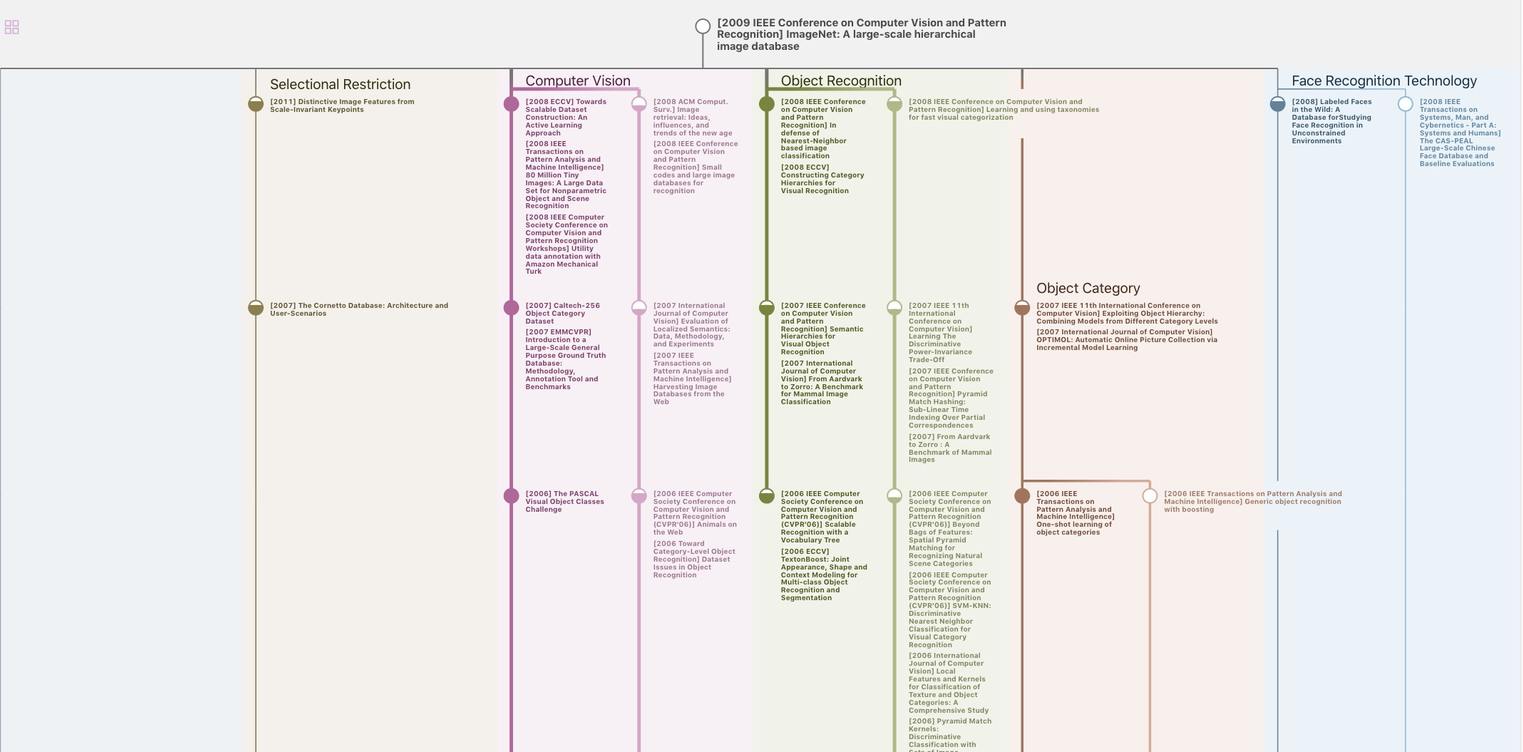
生成溯源树,研究论文发展脉络
Chat Paper
正在生成论文摘要