An In-Memory-Computing STT-MRAM Macro with Analog ReLU and Pooling Layers for Ultra-High Efficient Neural Network
2023 IEEE 12th Non-Volatile Memory Systems and Applications Symposium (NVMSA)(2023)
摘要
In-memory computing (IMC) technology has great potential for neural network accelerators. However, the energy efficiency of current mainstream IMC designs is limited, since the peripheral circuitry (e.g., ADC) for multiply-and-accumulations (MACs) and nonlinear operations (e.g., max pooling, ReLU etc.) are expensive. This paper proposes an in-memory computing STT-MRAM macro with analog ReLU and pooling layers for efficient neural networks. By implementing the activation and max pooling layers before the ADC through analog domain, the overhead (both latency and energy) of the ADC can be significantly reduced. The macro was implemented in an industrialized 22nm process and the results show that our STT-MRAM IMC macro can reduce 2.02~2.71x energy and 1.8x latency in comparison with various scales of the macro.
更多查看译文
关键词
in-memory computing, STT-MRAM, neural network accelerator
AI 理解论文
溯源树
样例
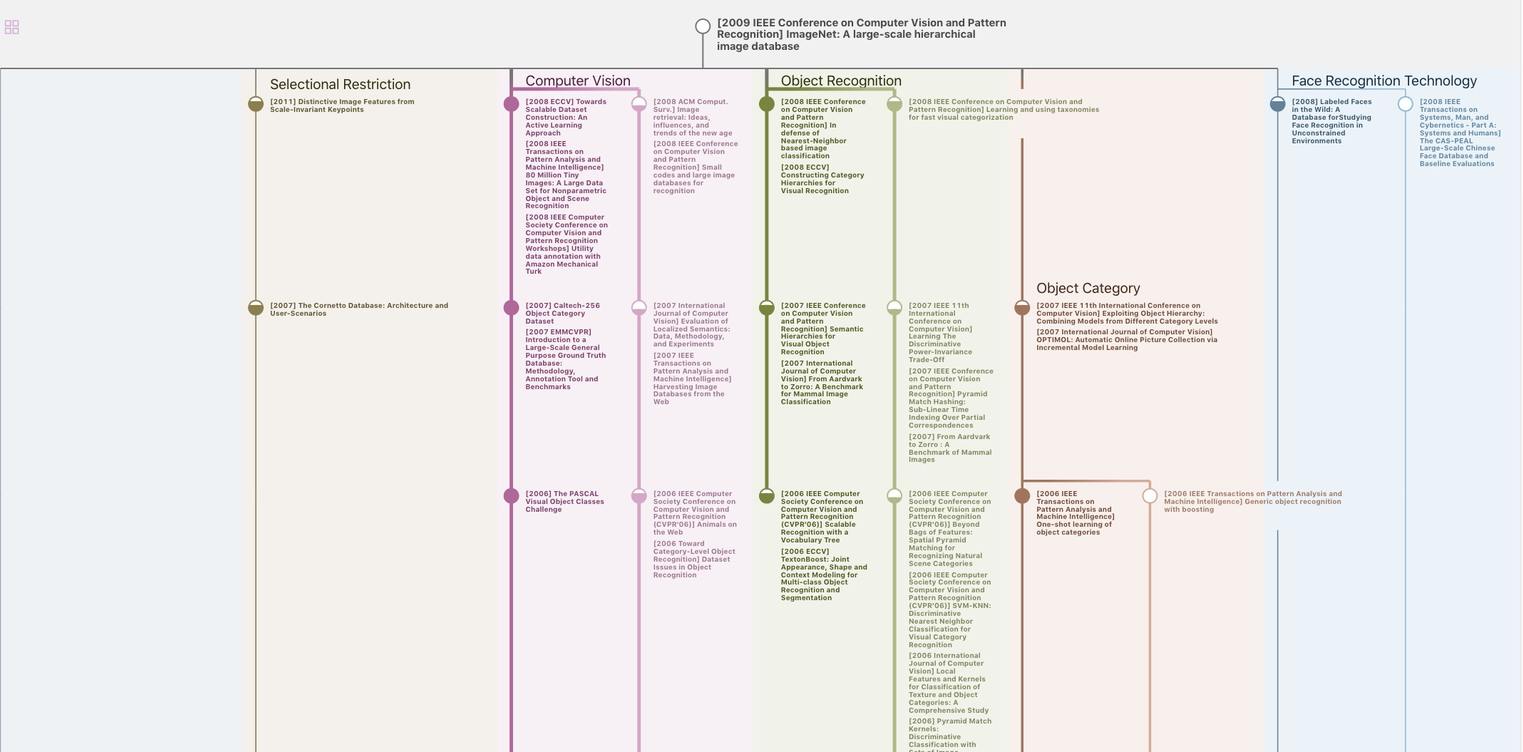
生成溯源树,研究论文发展脉络
Chat Paper
正在生成论文摘要