ES-MPQ: Evolutionary Search Enabled Mixed Precision Quantization Framework for Computing-in-Memory
2023 IEEE 12th Non-Volatile Memory Systems and Applications Symposium (NVMSA)(2023)
摘要
Network quantization can effectively reduce the complexity without changing the network structures, which is conducive to deploying deep neural networks (DNN) on edge devices. However, most of the existing methods set the quantization precision manually and rarely consider the case that the computing array is limited, such as computing-in-memory (CIM). In this paper, we introduce a novel method named ES-MPQ, which employs evolutionary search to achieve mixed precision quantization with a small calibration dataset. The ES-MPQ can optimize multiple objectives to achieve better hardware efficiency. The experimental results for ResNet-18 on CIFAR-10 show that the proposed ES-MPQ can reduce the parameter size and energy consumption by up to 1.89x and 2.81x, respectively, compared with the fixed bit-width (8 bits) quantization, while losing only 0.59% accuracy.
更多查看译文
关键词
Mixed precision quantization, evolutionary search, computing-in-memory
AI 理解论文
溯源树
样例
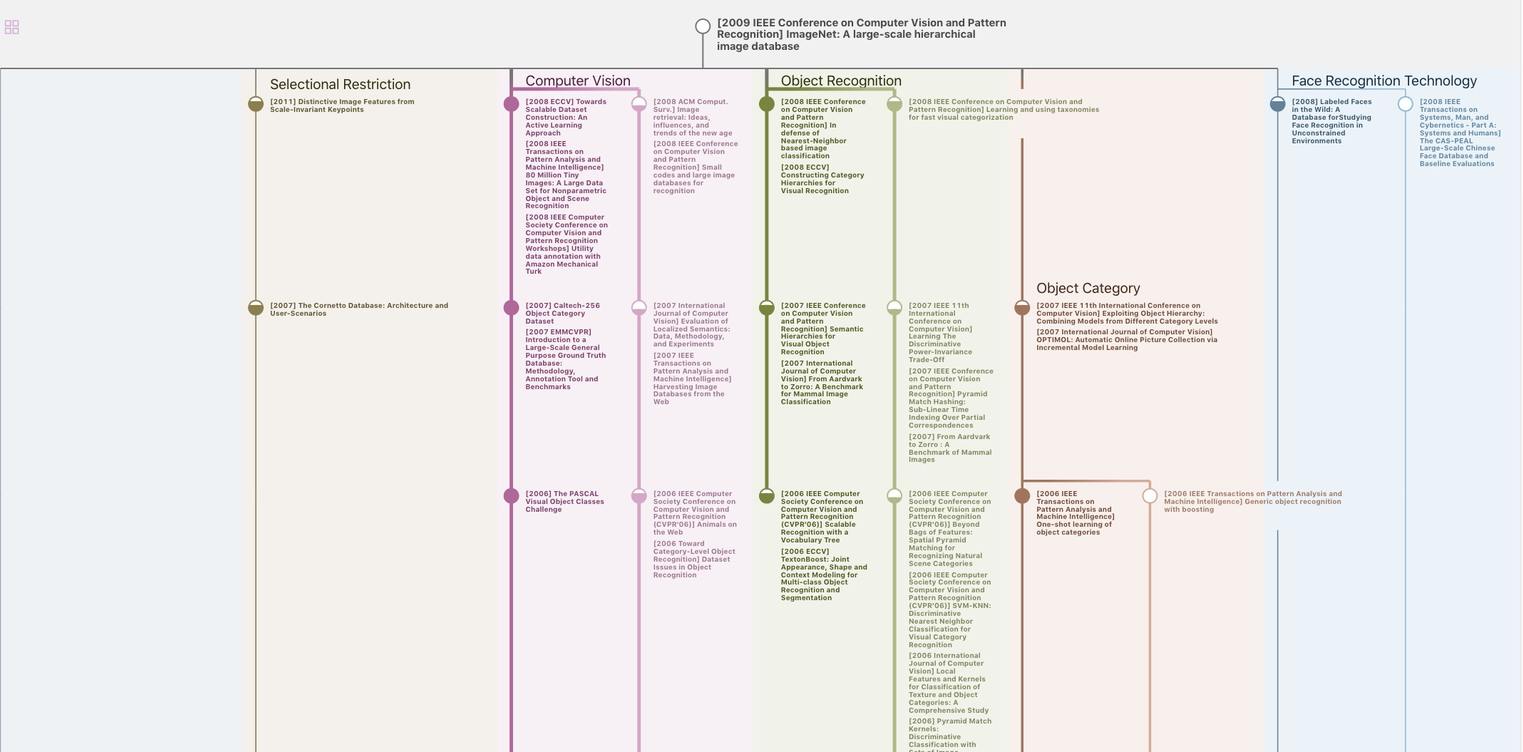
生成溯源树,研究论文发展脉络
Chat Paper
正在生成论文摘要