Communication-Efficient Federated Learning on Non-IID Data Using Two-Step Knowledge Distillation
IEEE Internet of Things Journal(2023)
摘要
Federated learning (FL) has shown its great potential for achieving distributed intelligence in privacy-sensitive IoT. However, popular FL approaches, such as FedAvg and its variants share model parameters among clients during the training process and thus cause significant communication overhead in IoT. Moreover, nonindependent and identically distributed (non-IID) data across learning devices severely affect the convergence and speed of FL. To address these challenges, we propose a communication-efficient FL framework based on Two-step Knowledge Distillation, Fed2KD, which boosts the classification accuracy through privacy-preserving data generation while improving communication efficiency through a new knowledge distillation scheme empowered by an attention mechanism and metric learning. The generalization ability of Fed2KD is analyzed from the view of domain adaption. Extensive simulation experiments are conducted on Fashion-MNIST, CIFAR-10, and ImageNet data sets with various non-IID data distributions. The performance results show that Fed2KD can reduce the communication overhead and improve classification accuracy compared to FedAvg and its latest variants.
更多查看译文
关键词
Classification, communication efficiency, federated learning (FL), knowledge distillation
AI 理解论文
溯源树
样例
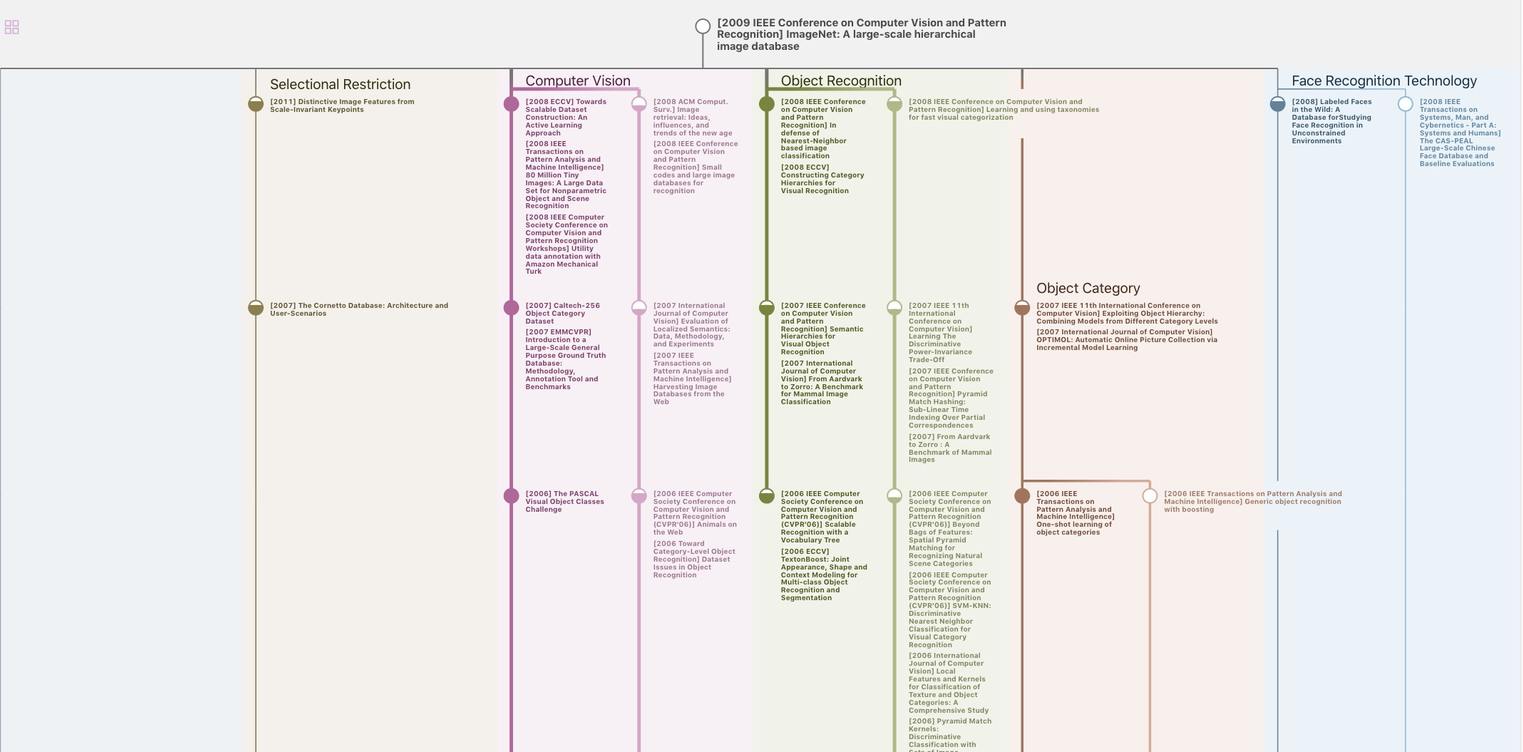
生成溯源树,研究论文发展脉络
Chat Paper
正在生成论文摘要