Load Balancing for Multiedge Collaboration in Wireless Metropolitan Area Networks: A Two-Stage Decision-Making Approach
IEEE Internet of Things Journal(2023)
摘要
Mobile edge computing (MEC) relieves the latency and energy consumption of mobile applications by offloading computation-intensive tasks to nearby edges. In wireless metropolitan area networks (WMANs), edges can better provide computing services via advanced communication technologies. For improving the Quality-of-Service (QoS), edges need to be collaborated rather than working alone. However, the existing solutions of multiedge collaboration solely adopt a centralized or decentralized decision-making way of load balancing, making it hard to achieve the optimal result because the local and global conditions are not jointly considered. To solve this problem, we propose a novel two-stage decision-making method of load balancing for multiedge collaboration (TDB-EC). First, the centralized decision making is executed with global information, where a deep neural networks (DNNs)-based prediction model is designed to evaluate the range of task scheduling between adjacent edges. Next, considering the global condition of load balancing, the decentralized decision making is executed with local information, where a deep
$Q$
-networks (DQN)-based
$Q$
-value prediction model of adjustment operations is developed to evaluate the load balancing plan among edges. Finally, the objective load balancing plan is obtained via feedback control. Extensive simulation experiments demonstrate the adaptability of the TDB-EC to various scenarios of multiedge load balancing, which approximates the optimal result and outperforms three classic methods.
更多查看译文
关键词
Deep learning, load balancing, multiedge collaboration, reinforcement learning, wireless metropolitan area networks (WMANs)
AI 理解论文
溯源树
样例
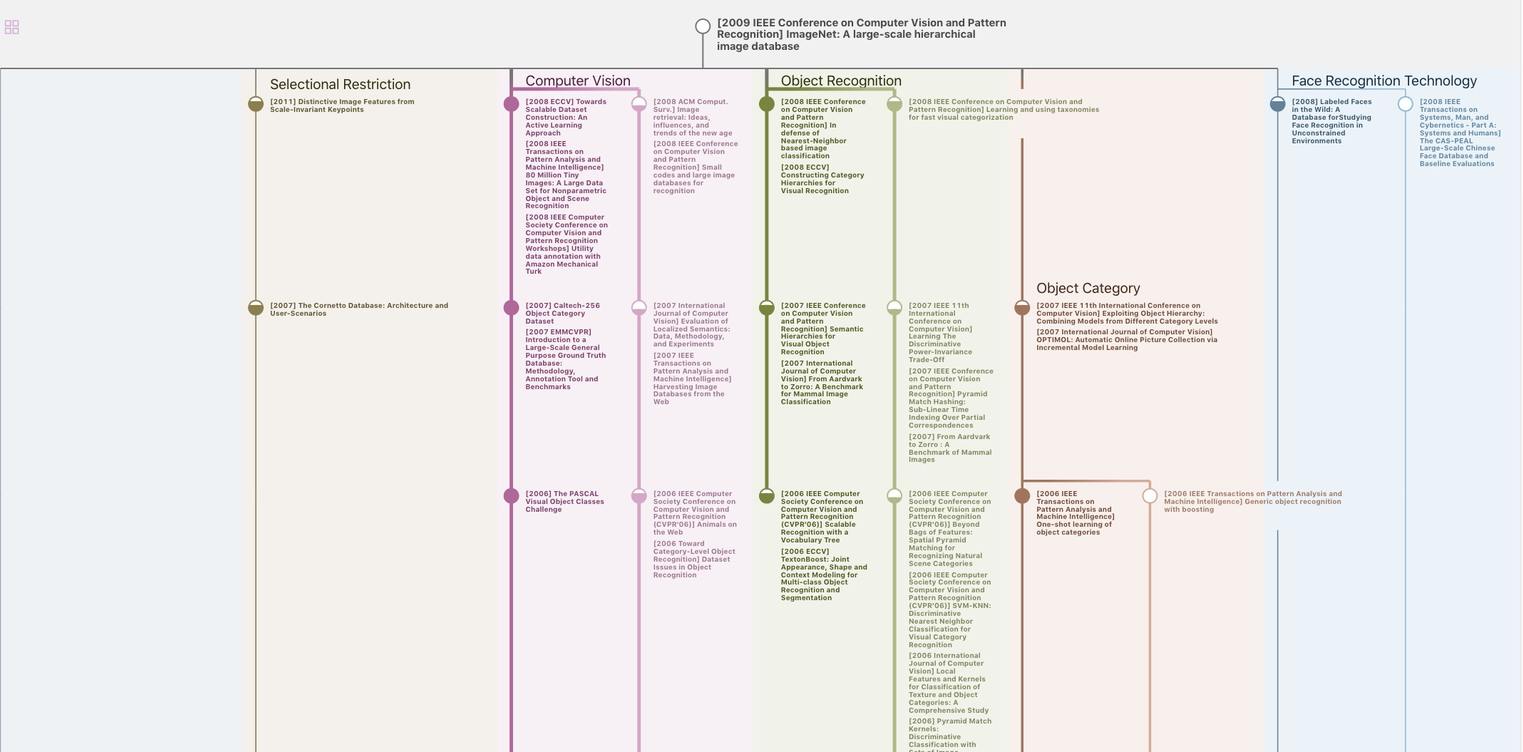
生成溯源树,研究论文发展脉络
Chat Paper
正在生成论文摘要