HHSD: Hindi Hate Speech Detection Leveraging Multi-Task Learning
IEEE Access(2023)
Abstract
Hate speech is now a frequent occurrence on social media. Recently, the majority of study was devoted to identifying hate speech in languages with abundant resources (e.g., English). However, relatively few works are developed for languages with limited resources (e.g., Hindi, the third most widely used language on earth). In this study, Hindi Hate Speech Dataset (HHSD) is created following a novel hierarchical fine-grained four-layer annotation approach. The top layer separates the posts into hateful and non-hateful categories. The second layer further categorises hateful posts into explicit hateful and implicit hateful. The third layer is the multilabel tagging of the post into topics, such as political, religion, racism, or sexism. The fourth layer involves the identification of the targeted named entity, either explicitly or implicitly. Additionally, a thorough evaluation of the data annotation schema for trustworthy annotation is provided. The HHSD data is the largest multi-layer annotated corpora in Hindi compared with the existing multi-layer annotated data. Experiments on the dataset using the transformer-based approaches in single-task learning (STL) attain encouraging performances in accuracy and weighted-f1 score. The experiment leveraged multi-task learning (MTL) by including multiple related hate speech detection tasks from high-resource English and languages from the same linguistic family such as Urdu and Bangla with a transformer encoder as the shared layers to obtain a significant increment of 5.31% and 5.35% over STL in accuracy and weighted-f1 for layer A, 8.20%, and 22.83% for layer B. The MTL surpasses STL by 8.98% and 4.07% in exact match and hamming loss for layer C.
MoreTranslated text
Key words
Transformers,multi-task learning,F1 score,accuracy,Shared layers
AI Read Science
Must-Reading Tree
Example
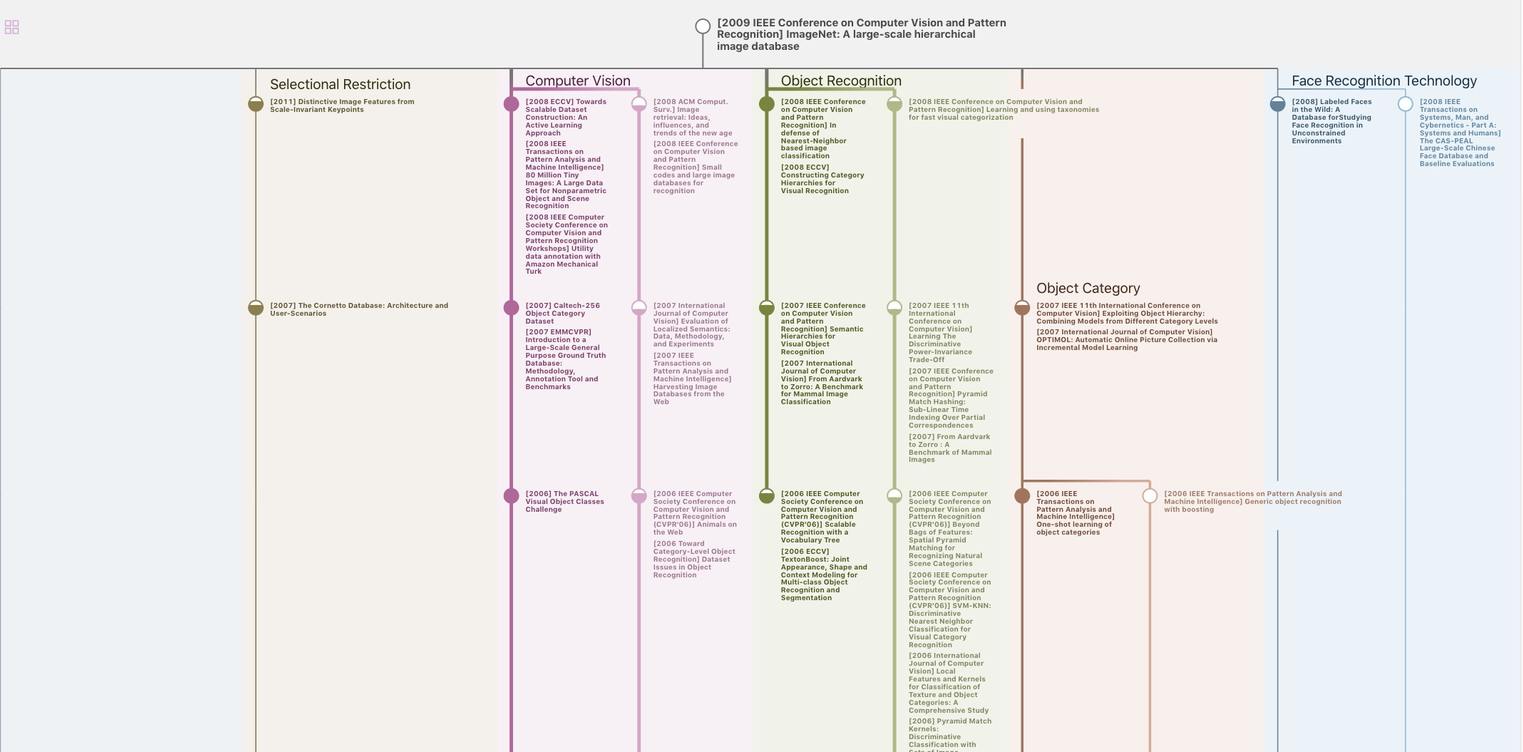
Generate MRT to find the research sequence of this paper
Chat Paper
Summary is being generated by the instructions you defined