A New Dual-Input Deep Anomaly Detection Method for Early Faults Warning of Rolling Bearings
SENSORS(2023)
摘要
To address the problem of low fault diagnosis accuracy caused by insufficient fault samples of rolling bearings, a dual-input deep anomaly detection method with zero fault samples is proposed for early fault warning of rolling bearings. First, the main framework of dual-input feature extraction based on a convolutional neural network (CNN) is established, and the two outputs of the main frame are subjected to the autoencoder structure. Then, the secondary feature extraction is performed. At the same time, the experience pool structure is introduced to improve the feature learning ability of the network. A new objective loss function is also proposed to learn the network parameters. Then, the vibration acceleration signal is preprocessed by wavelet to obtain multiple signals in different frequency bands, and the two signals in the high-frequency band are two-dimensionally encoded and used as the network input. Finally, the unsupervised learning of the model is completed on five sets of actual full-life rolling bearing fault data sets relying only on some samples in a normal state. The verification results show that the proposed method can realize earlier than the RMS, Kurtosis, and other features. The early fault warning and the accuracy rate of more than 98% show that the method is highly capable of early fault warning and anomaly detection.
更多查看译文
关键词
rolling bearing,dual-input deep anomaly detection,unsupervised learning,zero fault samples,CNN
AI 理解论文
溯源树
样例
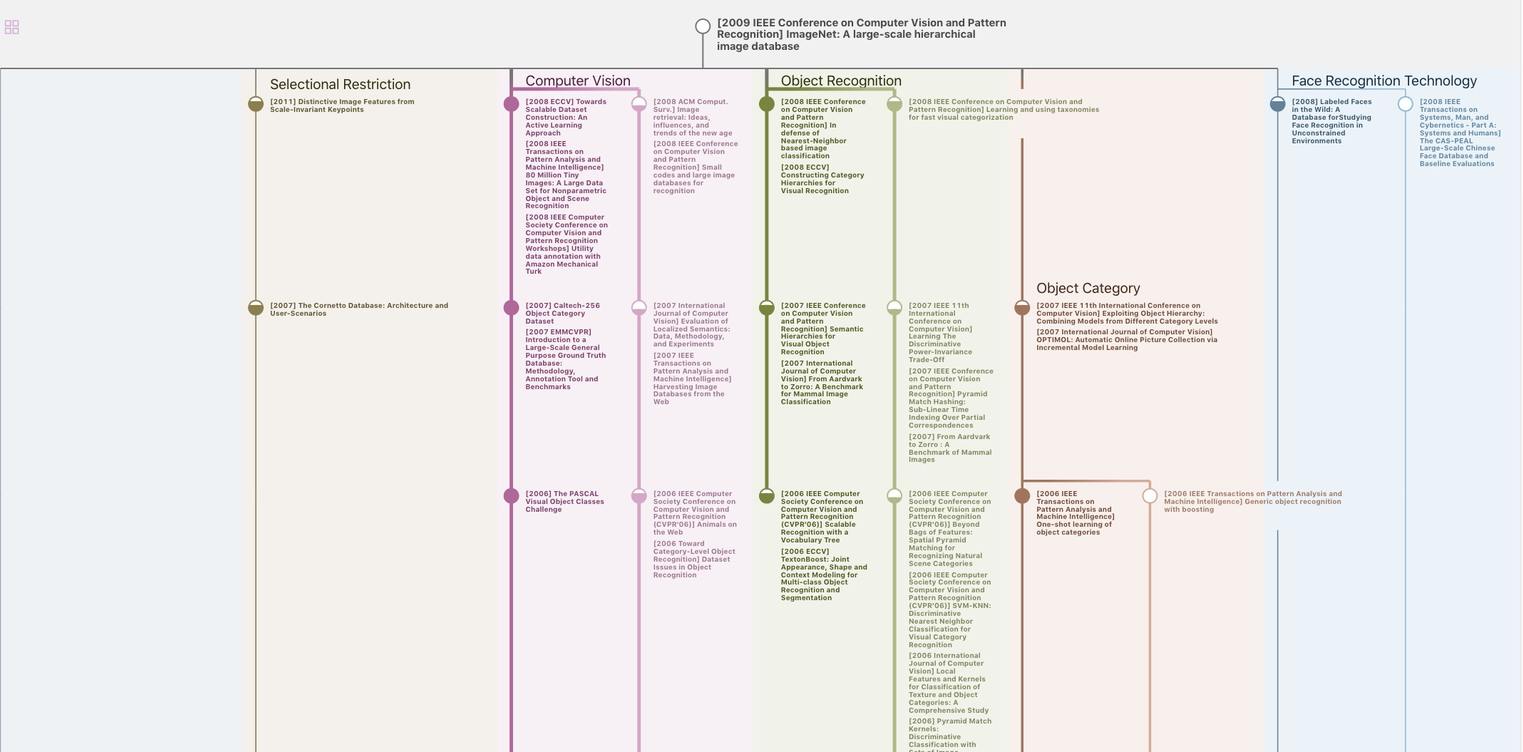
生成溯源树,研究论文发展脉络
Chat Paper
正在生成论文摘要