Multi-task Cooperative Learning via Searching for Flat Minima
MEDICAL IMAGE COMPUTING AND COMPUTER ASSISTED INTERVENTION, MICCAI 2023 WORKSHOPS(2023)
摘要
Multi-task learning (MTL) has shown great potential in medical image analysis, improving the generalizability of the learned features and the performance in individual tasks. However, most of the work on MTL focuses on either architecture design or gradient manipulation, while in both scenarios, features are learned in a competitive manner. In this work, we propose to formulate MTL as a multi/bi-level optimization problem, and therefore force features to learn from each task in a cooperative approach. Specifically, we update the sub-model for each task alternatively taking advantage of the learned sub-models of the other tasks. To alleviate the negative transfer problem during the optimization, we search for flat minima for the current objective function with regard to features from other tasks. To demonstrate the effectiveness of the proposed approach, we validate our method on three publicly available datasets. The proposed method shows the advantage of cooperative learning, and yields promising results when compared with the state-of-the-art MTL approaches. The code will be available online.
更多查看译文
关键词
Multi-Task,Cooperative Learning,Optimization
AI 理解论文
溯源树
样例
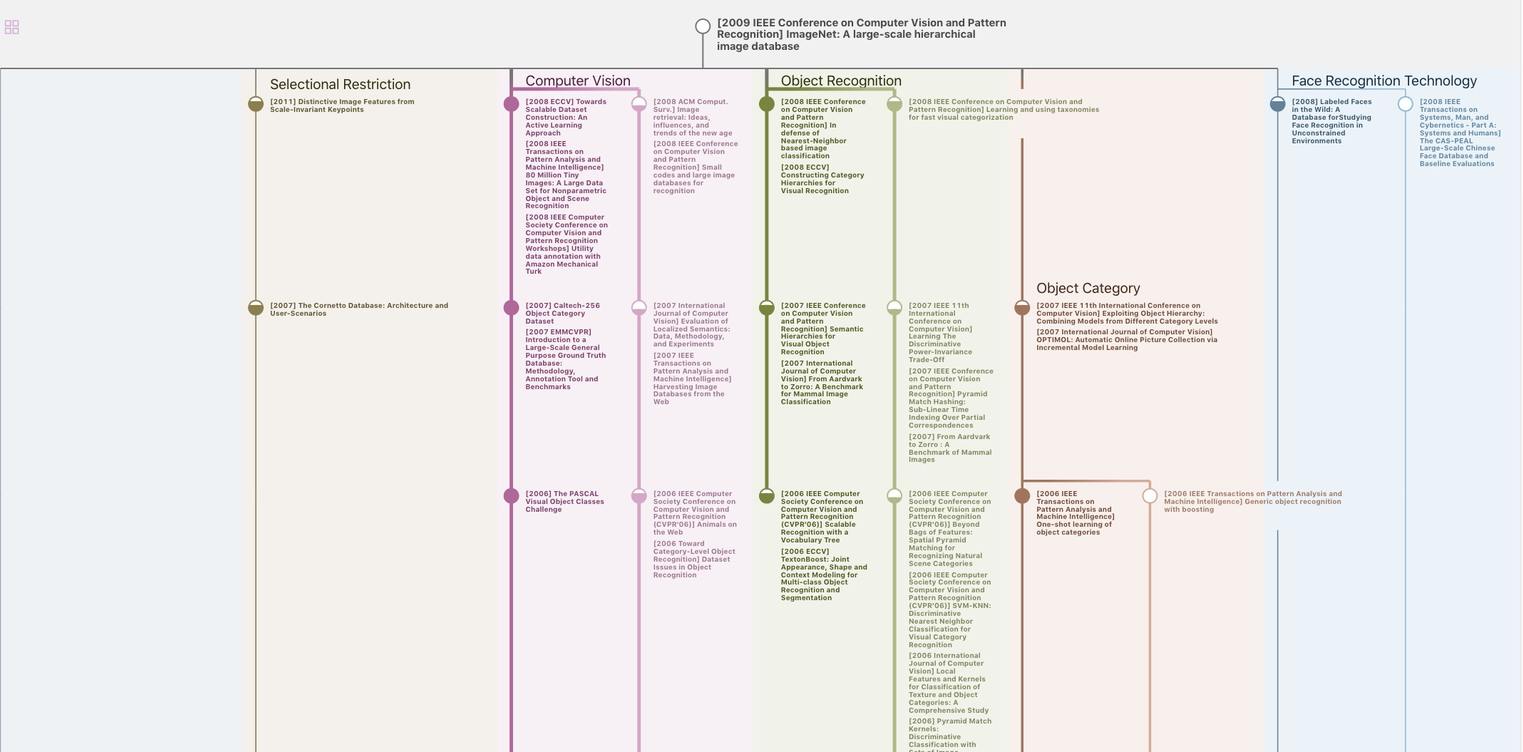
生成溯源树,研究论文发展脉络
Chat Paper
正在生成论文摘要