Privacy-Preserving In-Context Learning with Differentially Private Few-Shot Generation
arXiv (Cornell University)(2023)
摘要
We study the problem of in-context learning (ICL) with large language models
(LLMs) on private datasets. This scenario poses privacy risks, as LLMs may leak
or regurgitate the private examples demonstrated in the prompt. We propose a
novel algorithm that generates synthetic few-shot demonstrations from the
private dataset with formal differential privacy (DP) guarantees, and show
empirically that it can achieve effective ICL. We conduct extensive experiments
on standard benchmarks and compare our algorithm with non-private ICL and
zero-shot solutions. Our results demonstrate that our algorithm can achieve
competitive performance with strong privacy levels. These results open up new
possibilities for ICL with privacy protection for a broad range of
applications.
更多查看译文
关键词
learning,privacy-preserving,in-context,few-shot
AI 理解论文
溯源树
样例
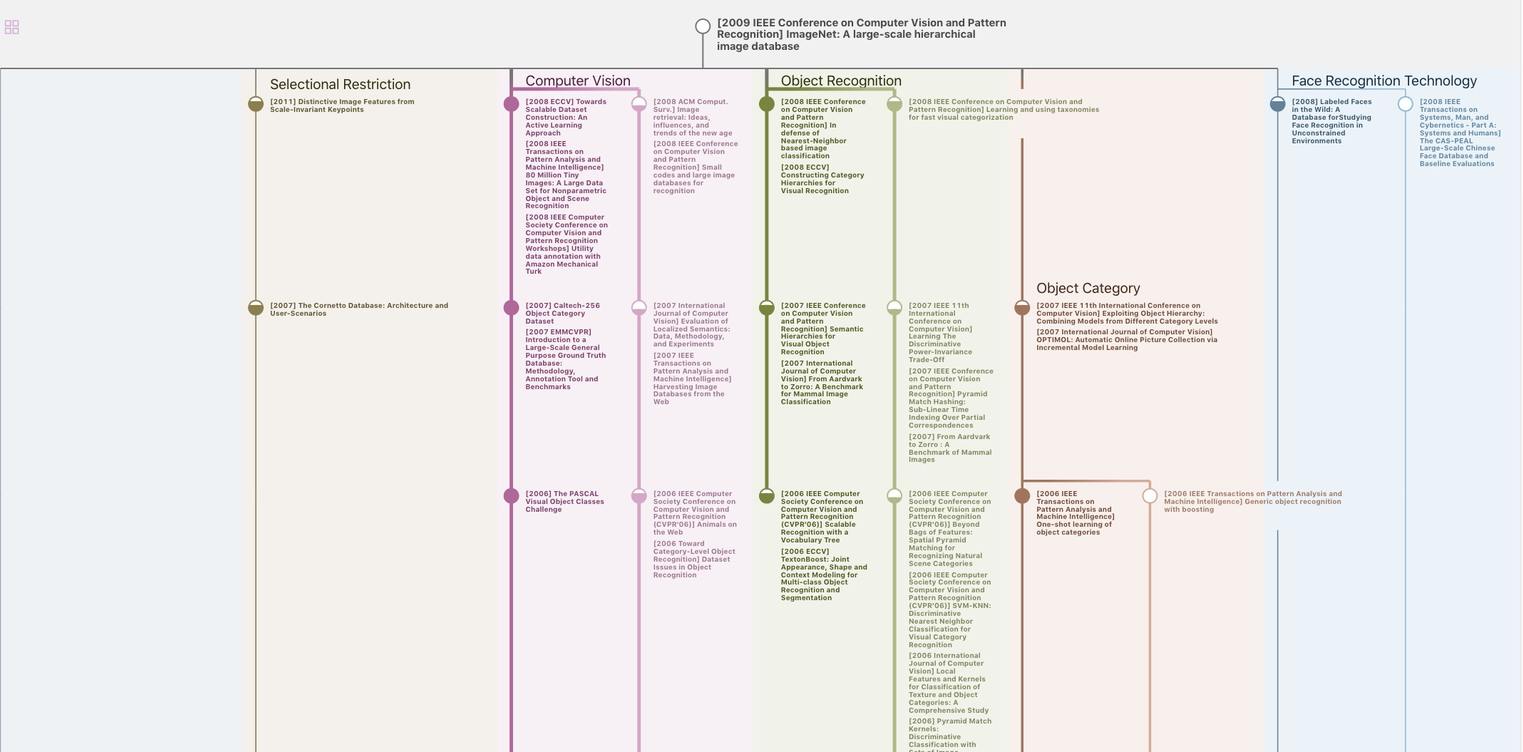
生成溯源树,研究论文发展脉络
Chat Paper
正在生成论文摘要