TURACO: Complexity-Guided Data Sampling for Training Neural Surrogates of Programs
CoRR(2023)
Abstract
Programmers and researchers are increasingly developing surrogates of programs, models of a subset of the observable behavior of a given program, to solve a variety of software development challenges. Programmers train surrogates frommeasurements of the behavior of a program on a dataset of input examples. A key challenge of surrogate construction is determining what training data to use to train a surrogate of a given program. We present a methodology for sampling datasets to train neural-network-based surrogates of programs. We first characterize the proportion of data to sample from each region of a program's input space (corresponding to different execution paths of the program) based on the complexity of learning a surrogate of the corresponding execution path. We next provide a program analysis to determine the complexity of different paths in a program. We evaluate these results on a range of real-world programs, demonstrating that complexity-guided sampling results in empirical improvements in accuracy.
MoreTranslated text
Key words
training neural surrogates,data sampling,complexity-guided
AI Read Science
Must-Reading Tree
Example
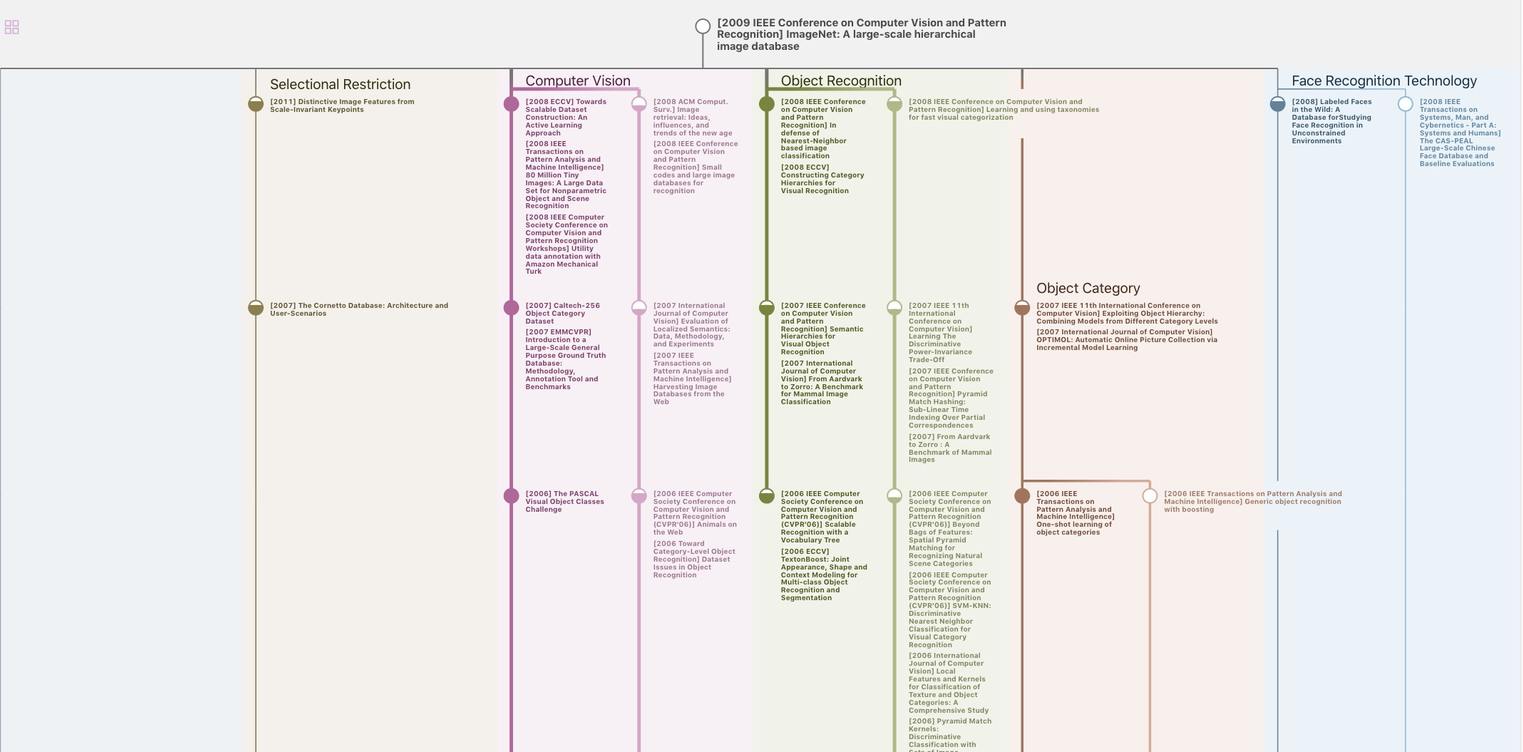
Generate MRT to find the research sequence of this paper
Chat Paper
Summary is being generated by the instructions you defined