Efficient Long-Short Temporal Attention network for unsupervised Video Object Segmentation
PATTERN RECOGNITION(2024)
摘要
Unsupervised Video Object Segmentation (VOS) aims at identifying the contours of primary foreground objects in videos without any prior knowledge. However, previous methods do not fully use spatial-temporal context and fail to tackle this challenging task in real-time. This motivates us to develop an efficient Long -Short Temporal Attention network (termed LSTA) for unsupervised VOS task from a holistic view. Specifically, LSTA consists of two dominant modules, i.e., Long Temporal Memory and Short Temporal Attention. The former captures the long-term global pixel relations of the past frames and the current frame, which models constantly present objects by encoding appearance pattern. Meanwhile, the latter reveals the short-term local pixel relations of one nearby frame and the current frame, which models moving objects by encoding motion pattern. To speedup the inference, the efficient projection and the locality-based sliding window are adopted to achieve nearly linear time complexity for the two light modules, respectively. Extensive empirical studies on several benchmarks have demonstrated promising performances of the proposed method with high efficiency.
更多查看译文
关键词
Unsupervised video object segmentation,Long temporal memory,Short temporal attention,Efficient projection
AI 理解论文
溯源树
样例
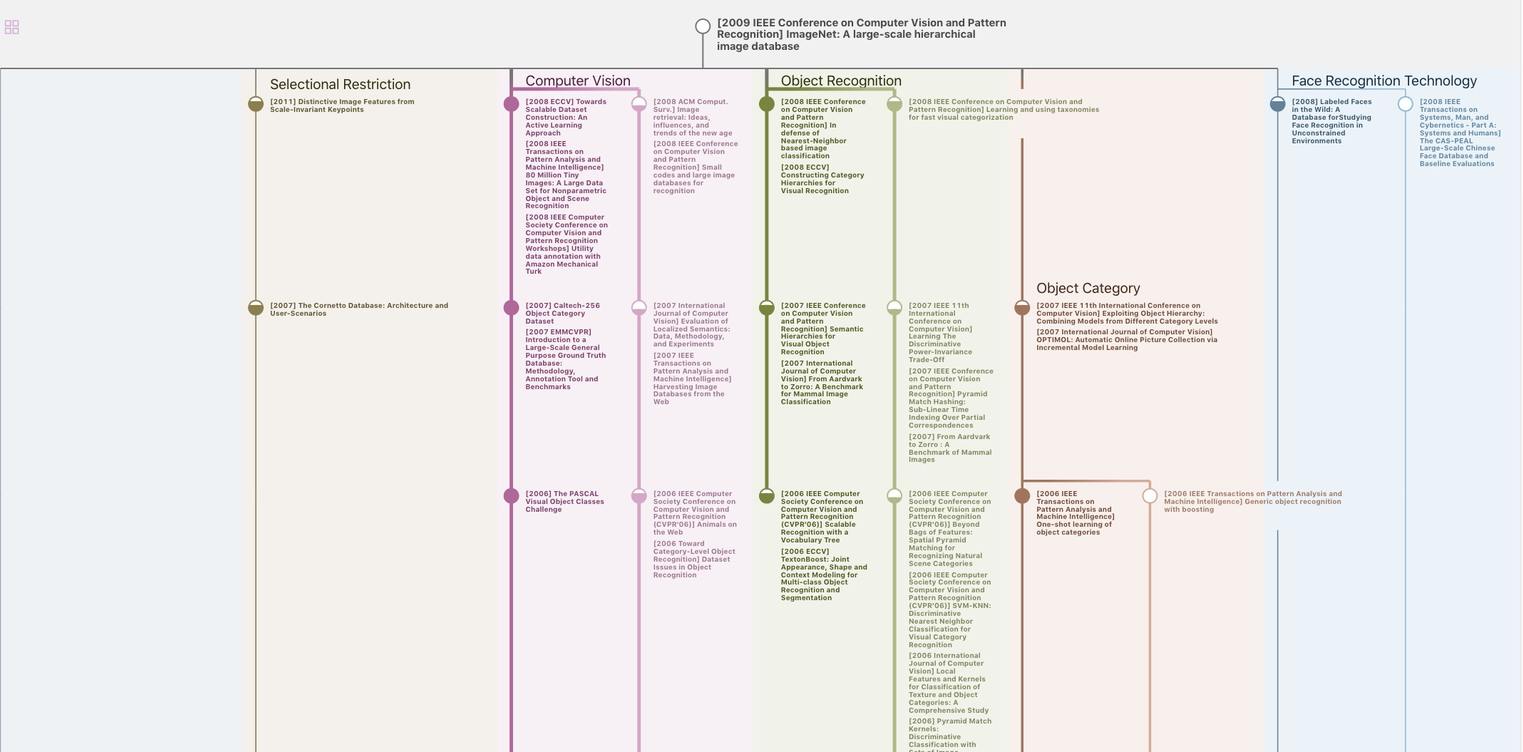
生成溯源树,研究论文发展脉络
Chat Paper
正在生成论文摘要