Kernel Extreme Learning Machine Based Prediction for Dynamic Multi-Objective Optimization
2023 9th International Conference on Control Science and Systems Engineering (ICCSSE)(2023)
摘要
Dynamic multi-objective optimization problems (DMOPs) are a class of special multi-objective problems (MOPs) in which constraints and parameters may change over time. Most existing approaches prefer to employ simple linear models to depict the relationships between consecutive environments, although the non-linear model seems more in line with reality. In this paper, the kernel extreme learning machine (KELM) is used as a predictor to learn the potential non-linear correlation between the constructed time series and then estimate the new positions of Pareto solutions. KELM enables efficient and accurate mapping of non-linear time series from low to high dimensional space and kernel mapping in high dimensional space. Specifically, Specifically, based on the reference point approach, the historical solutions are correlated to form multiple time series, which can maintain the diversity and distribution of the predicted population. Then, the constructed time series are fed into KELM as training samples to extract the moving trends of the time series solutions. When the environment changes, the trained KELM quickly predicts the positions of the solutions in the new environment and generates the corresponding initial population, enabling the algorithm to adapt to the dynamic environment better and faster. Comprehensive experiments are conducted on 14 benchmark problems of DF by comparing the proposed method with six popular algorithms. The experimental results demonstrate the superiority of the proposed method.
更多查看译文
关键词
dynamic multi-objective optimization problems,kernel extreme learning machine,time series,nonlinear mapping
AI 理解论文
溯源树
样例
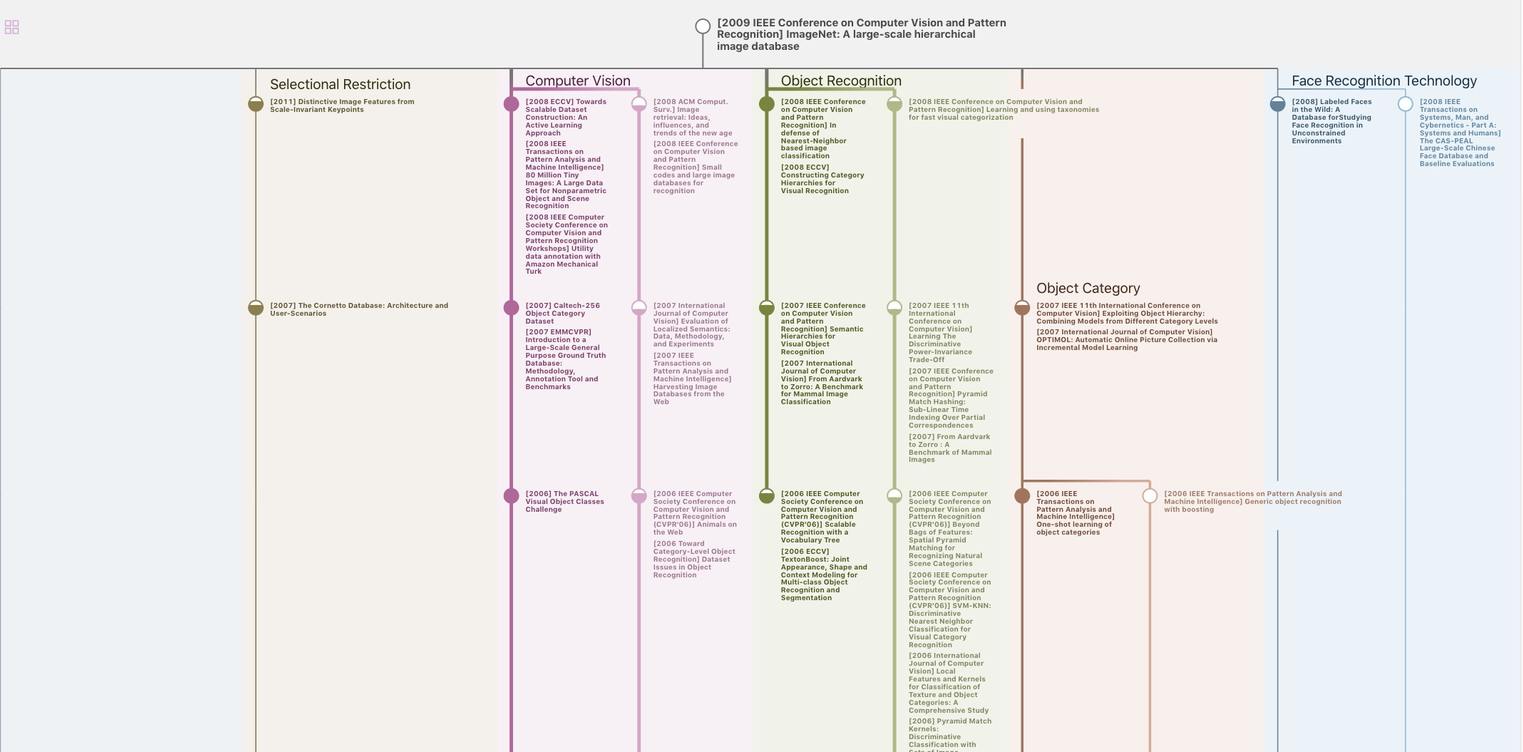
生成溯源树,研究论文发展脉络
Chat Paper
正在生成论文摘要