Solar-Mixer: An Efficient End-to-End Model for Long-Sequence Photovoltaic Power Generation Time Series Forecasting
IEEE Transactions on Sustainable Energy(2023)
摘要
The expansion of photovoltaic power generation makes photovoltaic power forecasting an essential requirement. With the development of deep learning, more accurate predictions have become possible. This paper proposes an efficient end-to-end model for solar power generation that allows for long-sequence time series forecasting. Two modules comprise the forecasting model: the anomaly detection module and the forecasting module. Singular values are detected and corrected by the anomaly detection module. And in the forecasting module, the series is first divided into different intervals. After that, the divided data is passed through a decomposition module embedded in the neural network, and each interval is encoded as high-dimension vectors. These vectors are fed into the mixing layer, which is used to learn the relationship between intervals and channels after the encoding process. Every mixing layer comprises two different layers: the channel-mixing layer allows communication between different channels, and the interval-mixing layer allows communication between different intervals. This neural network is purely dependent on the multilayer perceptron, resulting in a network with low system latency and low training cost. The experiments results not only show the model proposed in this paper can make accurate long-sequence time series forecasts for photovoltaic power generation at three sites but also show that the model can defeat the state-of-the-art model in long-sequence photovoltaic power generation time series forecasting work.
更多查看译文
关键词
forecasting,time series,solar-mixer,end-to-end,long-sequence
AI 理解论文
溯源树
样例
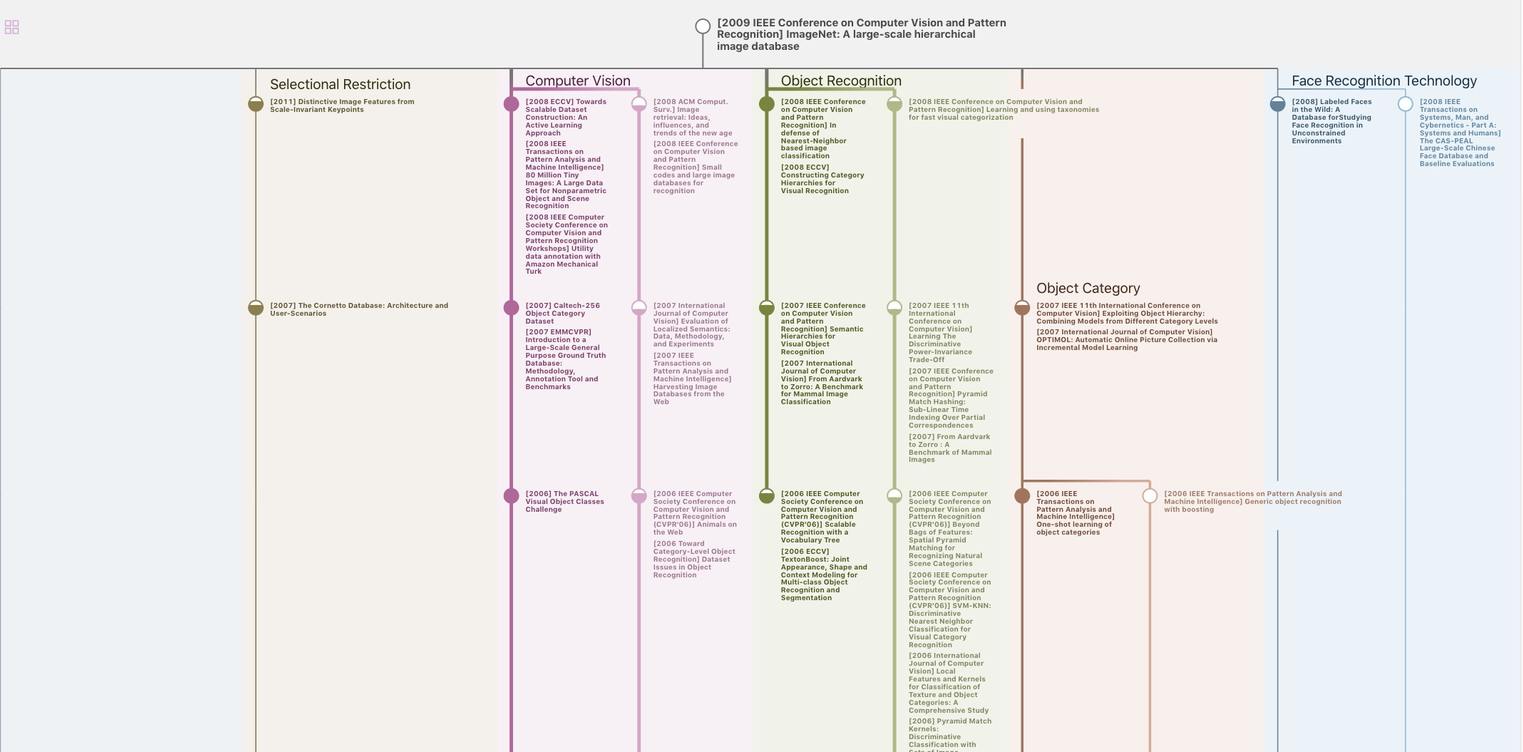
生成溯源树,研究论文发展脉络
Chat Paper
正在生成论文摘要