Neural fingerprinting on MEG time series using MiniRocket
Frontiers in Neuroscience(2023)
摘要
Neural fingerprinting is the identification of individuals in a cohort based on neuroimaging recordings of brain activity. In magneto- and electroencephalography (M/EEG), it is common practice to use second-order statistical measures, such as correlation or connectivity matrices, when neural fingerprinting is performed. These measures or features typically require coupling between signal channels and often ignore the individual temporal dynamics. In this study, we show that, following recent advances in multivariate time series classification, such as the development of the RandOm Convolutional KErnel Transformation (ROCKET) classifier, it is possible to perform classification directly on short time segments from MEG resting-state recordings with remarkably high classification accuracies. In a cohort of 124 subjects, it was possible to assign windows of time series of 1 s in duration to the correct subject with above 99% accuracy. The achieved accuracies are vastly superior to those of previous methods while simultaneously requiring considerably shorter time segments.
更多查看译文
关键词
meg time series,minirocket
AI 理解论文
溯源树
样例
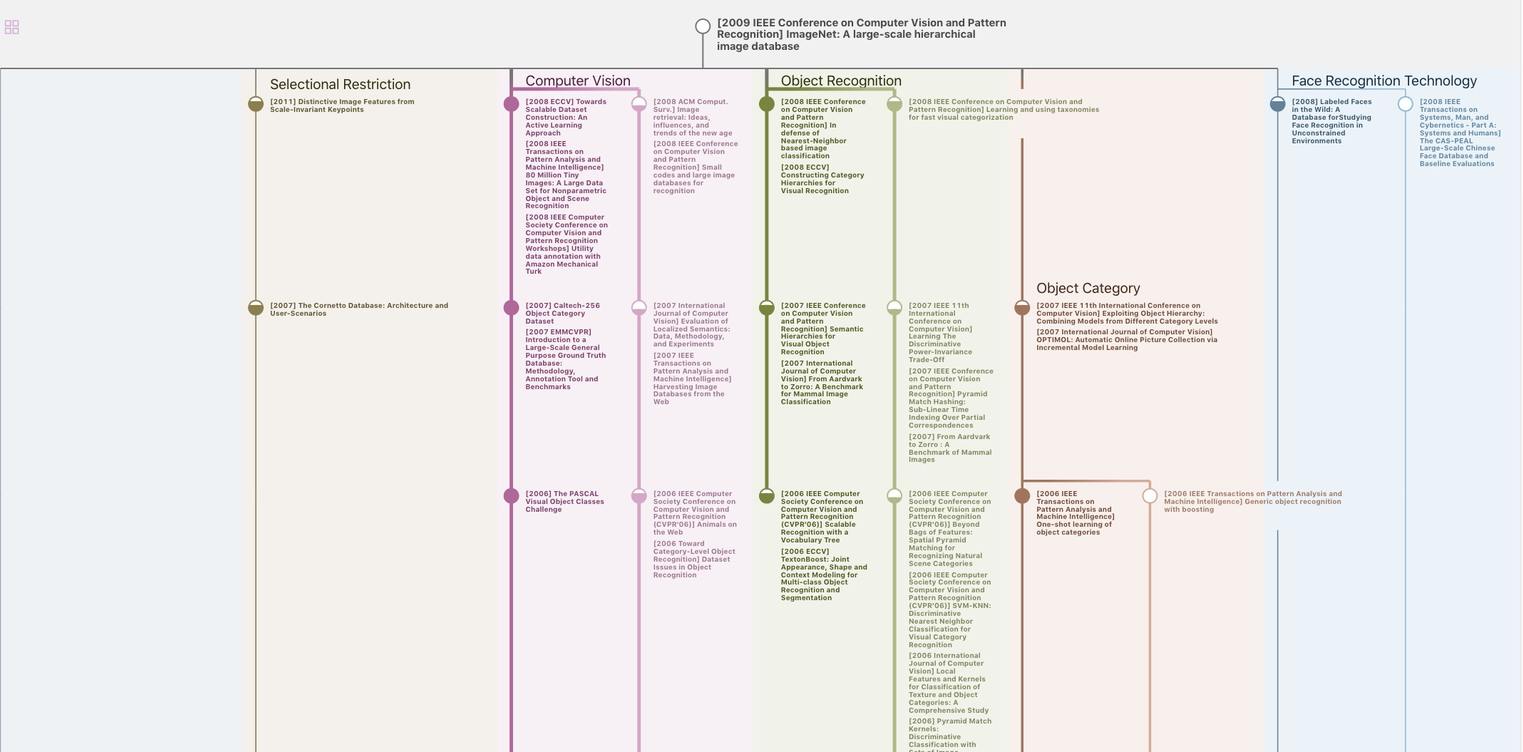
生成溯源树,研究论文发展脉络
Chat Paper
正在生成论文摘要