2D-3D Pose Tracking with Multi-View Constraints
CoRR(2023)
摘要
Camera localization in 3D LiDAR maps has gained increasing attention due to its promising ability to handle complex scenarios, surpassing the limitations of visual-only localization methods. However, existing methods mostly focus on addressing the cross-modal gaps, estimating camera poses frame by frame without considering the relationship between adjacent frames, which makes the pose tracking unstable. To alleviate this, we propose to couple the 2D-3D correspondences between adjacent frames using the 2D-2D feature matching, establishing the multi-view geometrical constraints for simultaneously estimating multiple camera poses. Specifically, we propose a new 2D-3D pose tracking framework, which consists: a front-end hybrid flow estimation network for consecutive frames and a back-end pose optimization module. We further design a cross-modal consistency-based loss to incorporate the multi-view constraints during the training and inference process. We evaluate our proposed framework on the KITTI and Argoverse datasets. Experimental results demonstrate its superior performance compared to existing frame-by-frame 2D-3D pose tracking methods and state-of-the-art vision-only pose tracking algorithms. More online pose tracking videos are available at \url{https://youtu.be/yfBRdg7gw5M}
更多查看译文
关键词
tracking,constraints,multi-view
AI 理解论文
溯源树
样例
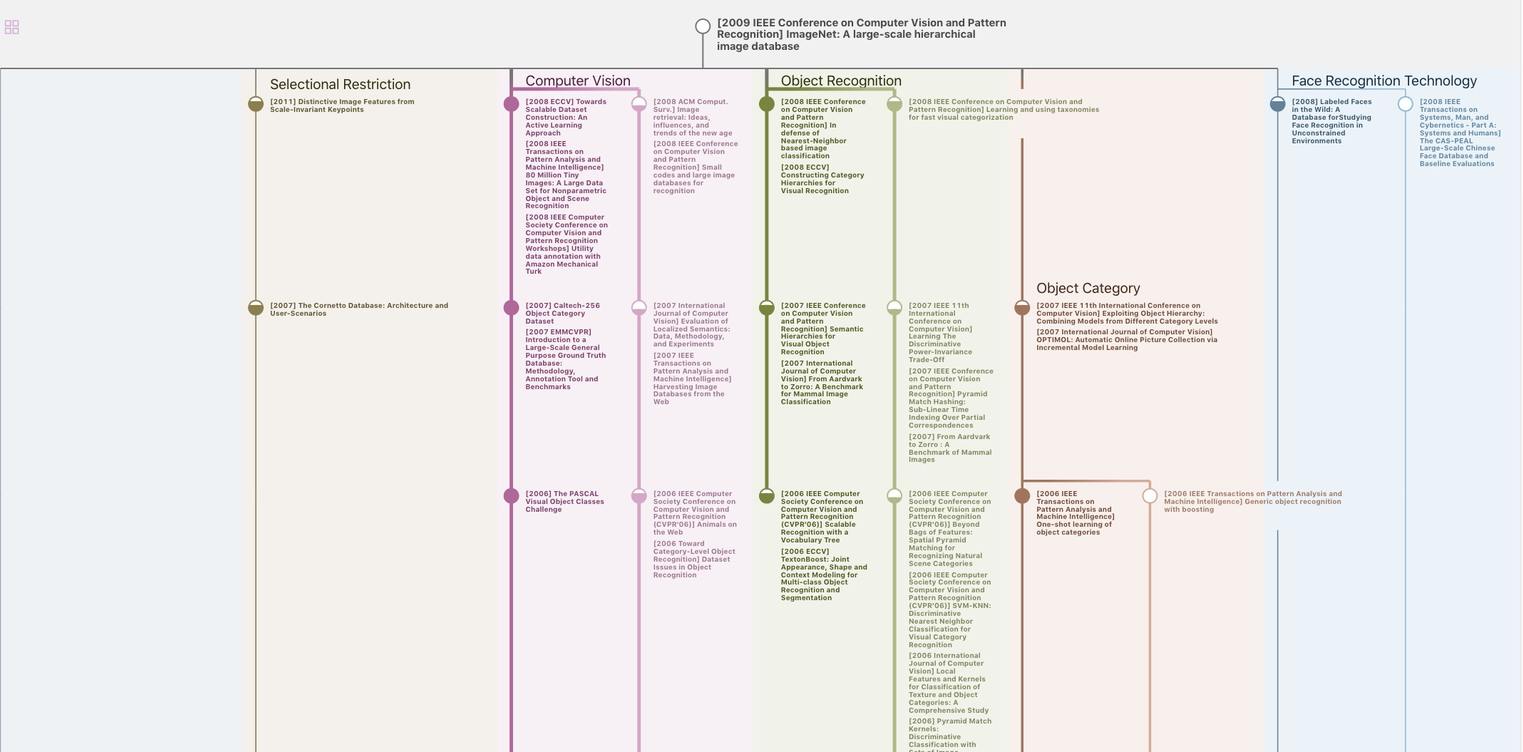
生成溯源树,研究论文发展脉络
Chat Paper
正在生成论文摘要