Energy-Harvesting-Aware Adaptive Inference of Deep Neural Networks in Embedded Systems
2023 IEEE/ACM International Symposium on Low Power Electronics and Design (ISLPED)(2023)
摘要
In energy harvesting IoT and sensor devices, energy influx is continuously changing and difficult to predict. Recently, the use of deep neural networks (DNNs), which consumes a large amount of energy, has increased in such devices. If a lightweight DNN model is used anticipating low energy influx, it may not achieve satisfactory inference accuracy in the ample energy flow condition. Conversely, using highly accurate sophisticated models may result in frequent inference failures due to energy depletion in situations with low energy influx. In this paper, for energy harvesting embedded systems that periodically perform DNN inference on sensor inputs, we propose an energy-harvesting-aware adaptive inference scheme to maximize inference accuracy while minimizing inference failures due to energy depletion in the long term. The model selector in the proposed scheme, which is a reinforcement learning (RL) agent, selects a DNN model from a model pool in consideration of the energy harvesting state and the accuracy and energy requirement of each model in the model pool. We implemented the proposed scheme on a microcontroller system and evaluated it with six different DNN applications with various types of input data. In the energy harvesting simulation with the real-world solar power traces, our approach, on average across the six workloads, was able to achieve a 65.62% reduction in inference failure rate with only a 6.08% increase in average error rate compared to the base DNN models.
更多查看译文
AI 理解论文
溯源树
样例
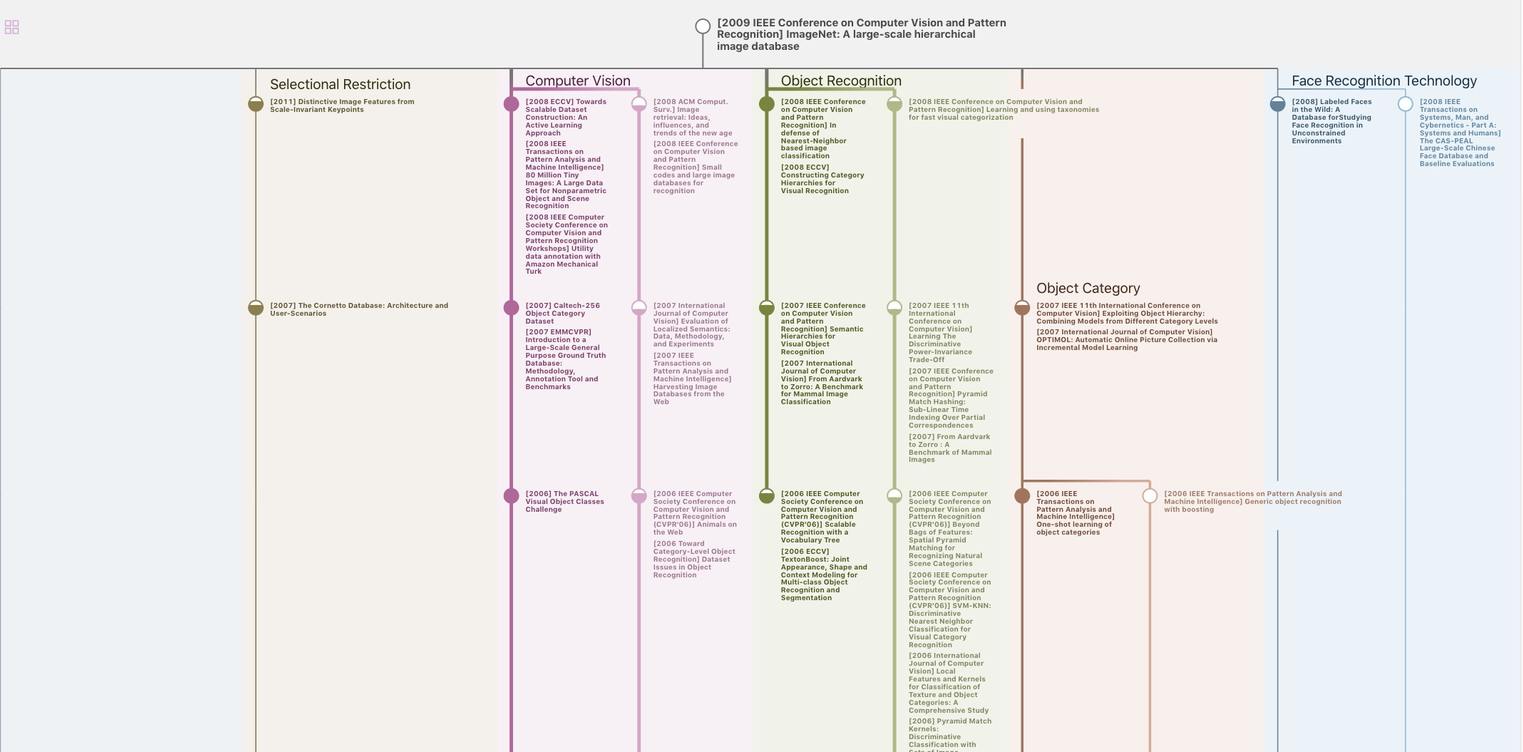
生成溯源树,研究论文发展脉络
Chat Paper
正在生成论文摘要