TH-SLP: Web Service Link Prediction Based on Topic-aware Heterogeneous Graph Neural Network
2023 IEEE International Conference on Web Services (ICWS)(2023)
摘要
With the emergence of more and more Web services, finding suitable services becomes a difficult problem. Service link prediction is employed to disclose relationships among services, which facilitates the further development of service composition, selection, and recommendation. But the existing link prediction approaches simply utilize the structural features of the service network. In reality, the rich text content in service node description documents also carries latent but fine-grained semantics generated by multifaceted topic-aware factors, yet few efforts are committed to mining them. In this paper, we propose a Web service link prediction method based on a topic-aware heterogeneous graph neural network. Specifically, the method consists of two main layers, including the meta-path intra-decomposition and the meta-path inter-mergence. Meta-path intra-decomposition aims to mine the topic distribution of the meta-paths-based context while capturing fine-grained topic-aware semantics. Meta-path inter-mergence uniquely aggregates topic-aware factors according to the mined distribution and adopts a multifaceted attention mechanism to aggregate different meta-paths, enabling service nodes to generate multifaceted topic-aware embeddings that preserve not only the structure and but also the topic-aware semantics. In addition, a topic prior guidance regularization item is set up for quality assurance of multifaceted topic-aware embedding that depends on global knowledge of the unstructured text content in description documents. Experimental results on real datasets show that our proposed model outperforms other existing baselines methods in the link prediction task, successfully validating the effectiveness of our proposed method.
更多查看译文
关键词
web service,service link prediction,heterogeneous graph neural network,topic-aware model,attention mechanism
AI 理解论文
溯源树
样例
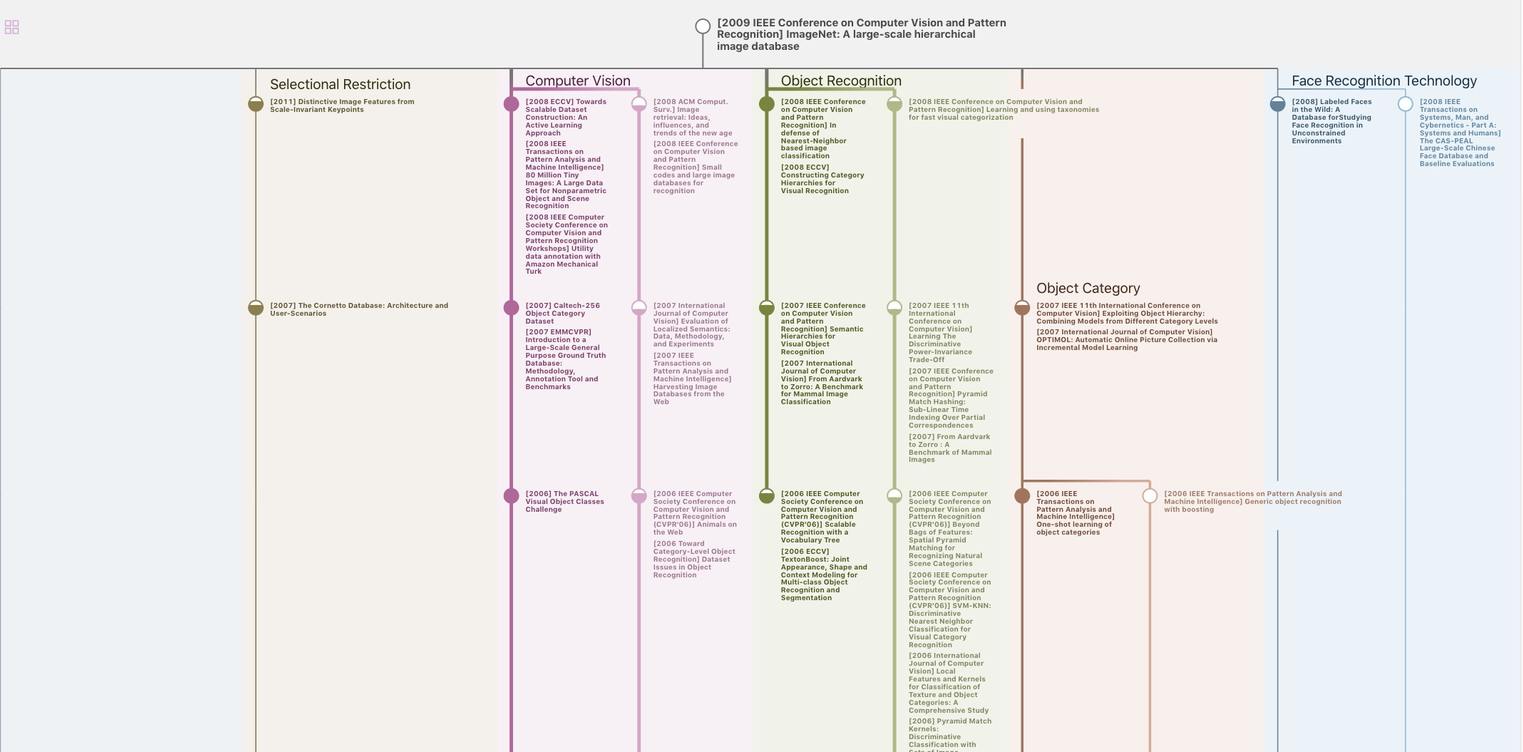
生成溯源树,研究论文发展脉络
Chat Paper
正在生成论文摘要