Next POI Recommendation Based on Spatial and Temporal Disentanglement Representation
2023 IEEE International Conference on Web Services (ICWS)(2023)
Key words
POI recommendation,disentangled representation,graph neural network,cold start,sequential recommendation
AI Read Science
Must-Reading Tree
Example
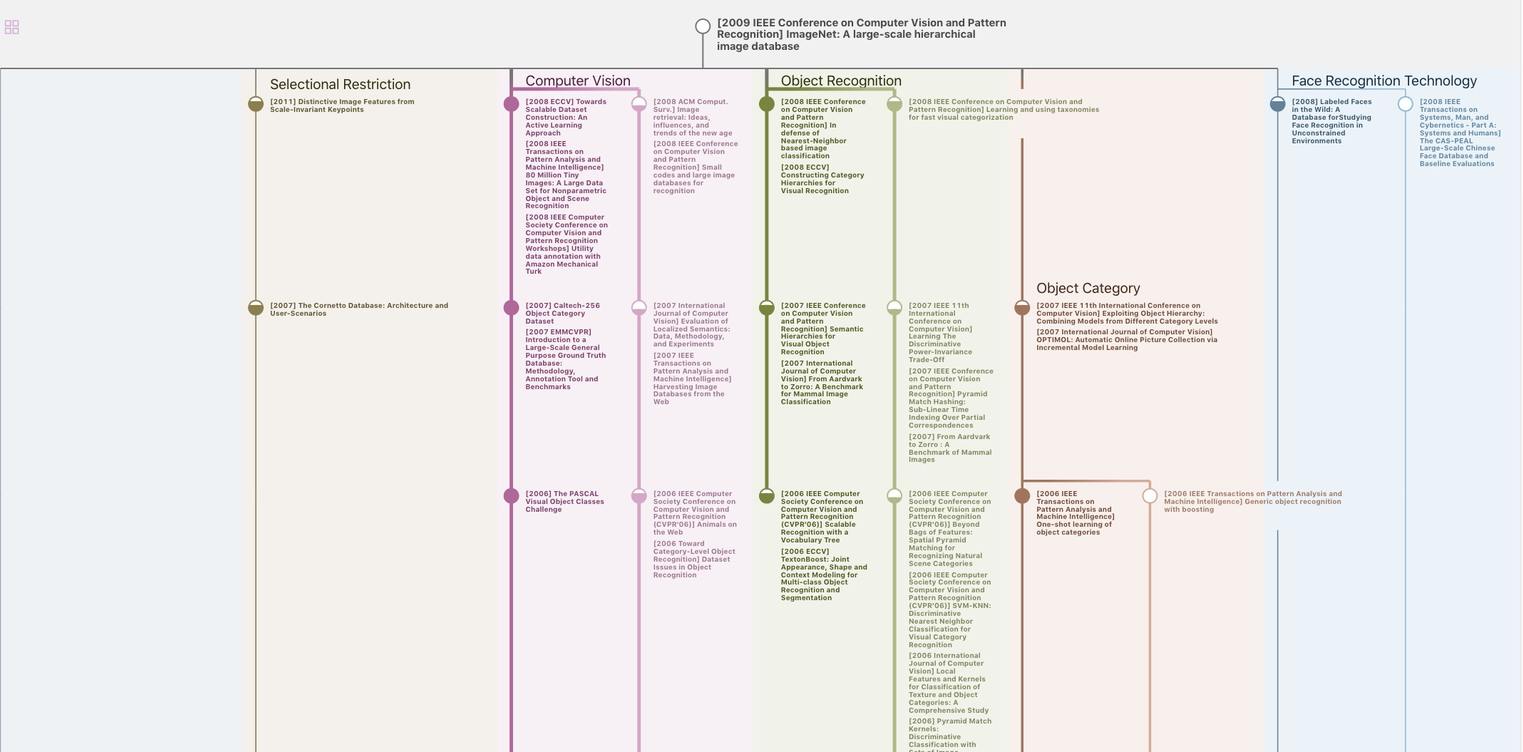
Generate MRT to find the research sequence of this paper
Chat Paper
Summary is being generated by the instructions you defined