Semisupervised Subspace Learning With Adaptive Pairwise Graph Embedding.
IEEE transactions on neural networks and learning systems(2023)
摘要
Graph-based semisupervised learning can explore the graph topology information behind the samples, becoming one of the most attractive research areas in machine learning in recent years. Nevertheless, existing graph-based methods also suffer from two shortcomings. On the one hand, the existing methods generate graphs in the original high-dimensional space, which are easily disturbed by noisy and redundancy features, resulting in low-quality constructed graphs that cannot accurately portray the relationships between data. On the other hand, most of the existing models are based on the Gaussian assumption, which cannot capture the local submanifold structure information of the data, thus reducing the discriminativeness of the learned low-dimensional representations. This article proposes a semisupervised subspace learning with adaptive pairwise graph embedding (APGE), which first builds a k -nearest neighbor graph on the labeled data to learn local discriminant embeddings for exploring the intrinsic structure of the non-Gaussian labeled data, i.e., the submanifold structure. Then, a k -nearest neighbor graph is constructed on all samples and mapped to GE learning to adaptively explore the global structure of all samples. Clustering unlabeled data and its corresponding labeled neighbors into the same submanifold, sharing the same label information, improves embedded data's discriminative ability. And the adaptive neighborhood learning method is used to learn the graph structure in the continuously optimized subspace to ensure that the optimal graph matrix and projection matrix are finally learned, which has strong robustness. Meanwhile, the rank constraint is added to the Laplacian matrix of the similarity matrix of all samples so that the connected components in the obtained similarity matrix are precisely equal to the number of classes in the sample, which makes the structure of the graph clearer and the relationship between the near-neighbor sample points more explicit. Finally, multiple experiments on several synthetic and real-world datasets show that the method performs well in exploring local structure and classification tasks.
更多查看译文
关键词
Adaptive neighbors,label propagation,local embedding learning,semisupervised dimension reduction
AI 理解论文
溯源树
样例
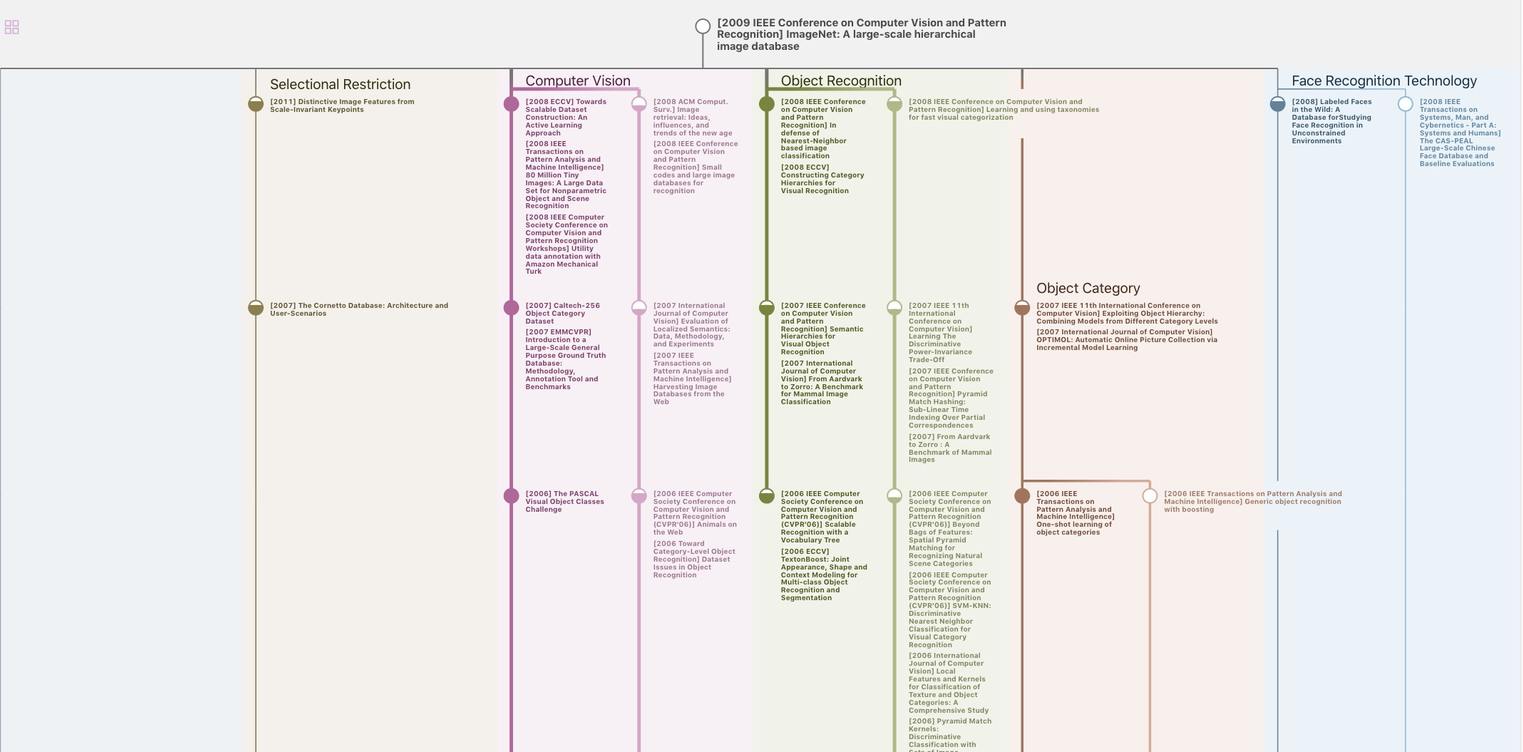
生成溯源树,研究论文发展脉络
Chat Paper
正在生成论文摘要