Monte-Carlo tree search with uncertainty propagation via optimal transport
CoRR(2023)
摘要
This paper introduces a novel backup strategy for Monte-Carlo Tree Search (MCTS) designed for highly stochastic and partially observable Markov decision processes. We adopt a probabilistic approach, modeling both value and action-value nodes as Gaussian distributions. We introduce a novel backup operator that computes value nodes as the Wasserstein barycenter of their action-value children nodes; thus, propagating the uncertainty of the estimate across the tree to the root node. We study our novel backup operator when using a novel combination of $L^1$-Wasserstein barycenter with $\alpha$-divergence, by drawing a notable connection to the generalized mean backup operator. We complement our probabilistic backup operator with two sampling strategies, based on optimistic selection and Thompson sampling, obtaining our Wasserstein MCTS algorithm. We provide theoretical guarantees of asymptotic convergence to the optimal policy, and an empirical evaluation on several stochastic and partially observable environments, where our approach outperforms well-known related baselines.
更多查看译文
关键词
uncertainty propagation,transport,tree,monte-carlo
AI 理解论文
溯源树
样例
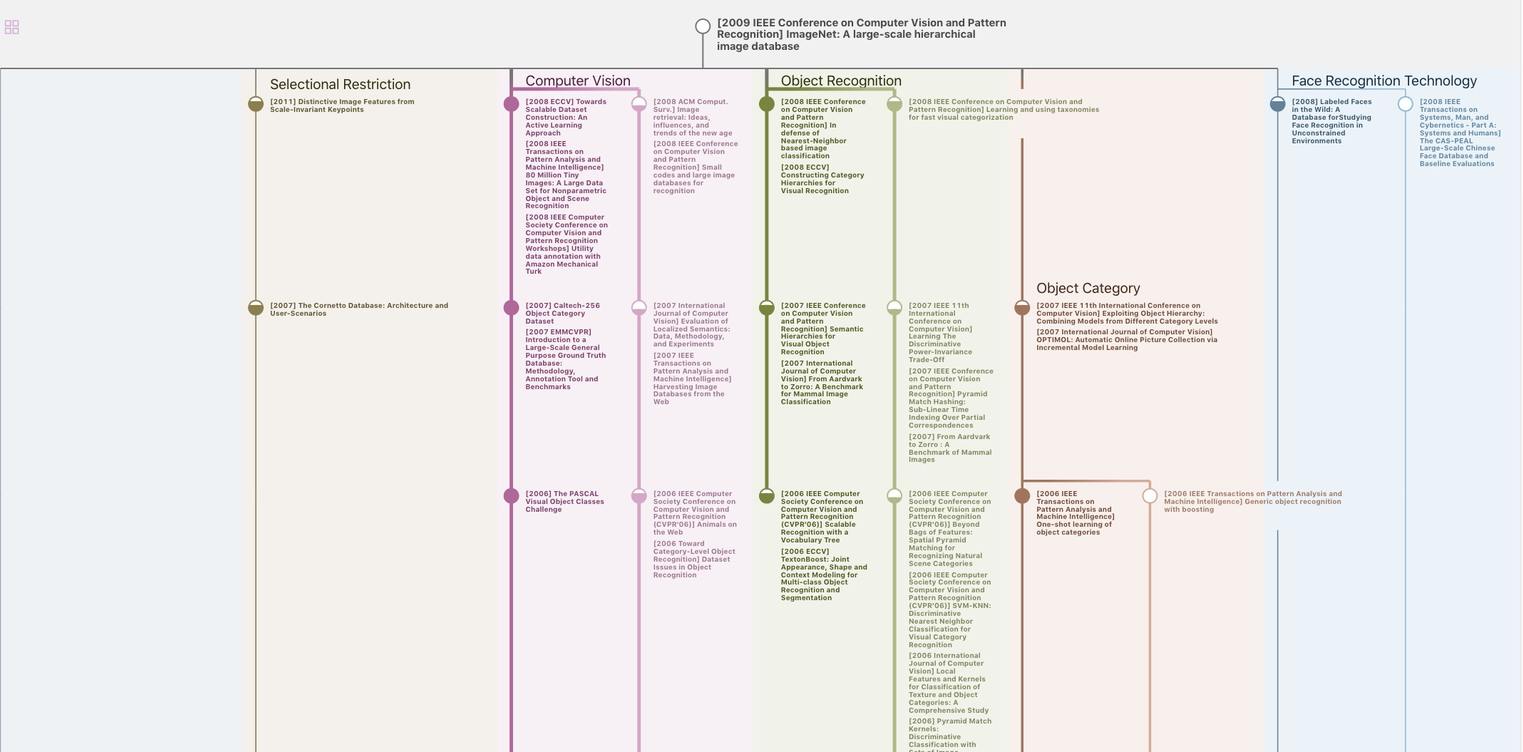
生成溯源树,研究论文发展脉络
Chat Paper
正在生成论文摘要