Cross-Modal and Cross-Domain Knowledge Transfer for Label-Free 3D Segmentation
PATTERN RECOGNITION AND COMPUTER VISION, PRCV 2023, PT III(2024)
摘要
Current state-of-the-art point cloud-based perception methods usually rely on large-scale labeled data, which requires expensive manual annotations. A natural option is to explore the unsupervised methodology for 3D perception tasks. However, such methods often face substantial performance-drop difficulties. Fortunately, we found that there exist amounts of image-based datasets and an alternative can be proposed, i.e., transferring the knowledge in the 2D images to 3D point clouds. Specifically, we propose a novel approach for the challenging cross-modal and cross-domain adaptation task by fully exploring the relationship between images and point clouds and designing effective feature alignment strategies. Without any 3D labels, our method achieves state-of-the-art performance for 3D point cloud semantic segmentation on SemanticKITTI by using the knowledge of KITTI360 and GTA5, compared to existing unsupervised and weakly-supervised baselines.
更多查看译文
关键词
Point Cloud Semantic Segmentation,Unsupervised Domain Adaptation,Cross-modal Transfer Learning
AI 理解论文
溯源树
样例
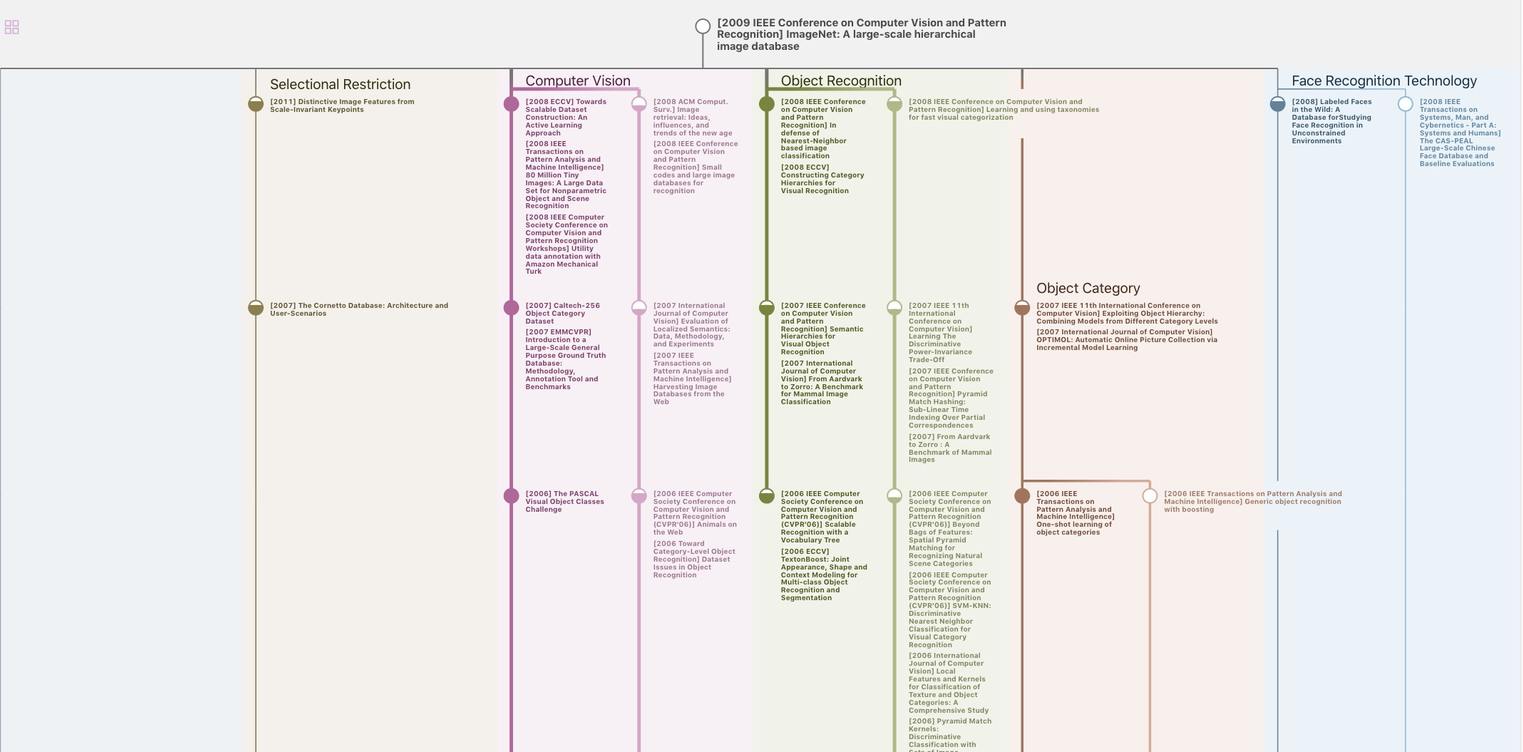
生成溯源树,研究论文发展脉络
Chat Paper
正在生成论文摘要