Enhancing quantum state tomography via resource-efficient attention-based neural networks
arXiv (Cornell University)(2023)
摘要
Resource-efficient quantum state tomography is one of the key ingredients of future quantum technologies. In this work, we propose a new tomography protocol combining standard quantum state reconstruction methods with an attention-based neural network architecture. We show how the proposed protocol is able to improve the averaged fidelity reconstruction over linear inversion and maximum-likelihood estimation in the finite-statistics regime, reducing at least by an order of magnitude the amount of necessary training data. We demonstrate the potential use of our protocol in physically relevant scenarios, in particular, to certify metrological resources in the form of many-body entanglement generated during the spin squeezing protocols. This could be implemented with the current quantum simulator platforms, such as trapped ions, and ultra-cold atoms in optical lattices.
更多查看译文
关键词
quantum state tomography,neural networks,resource-efficient,attention-based
AI 理解论文
溯源树
样例
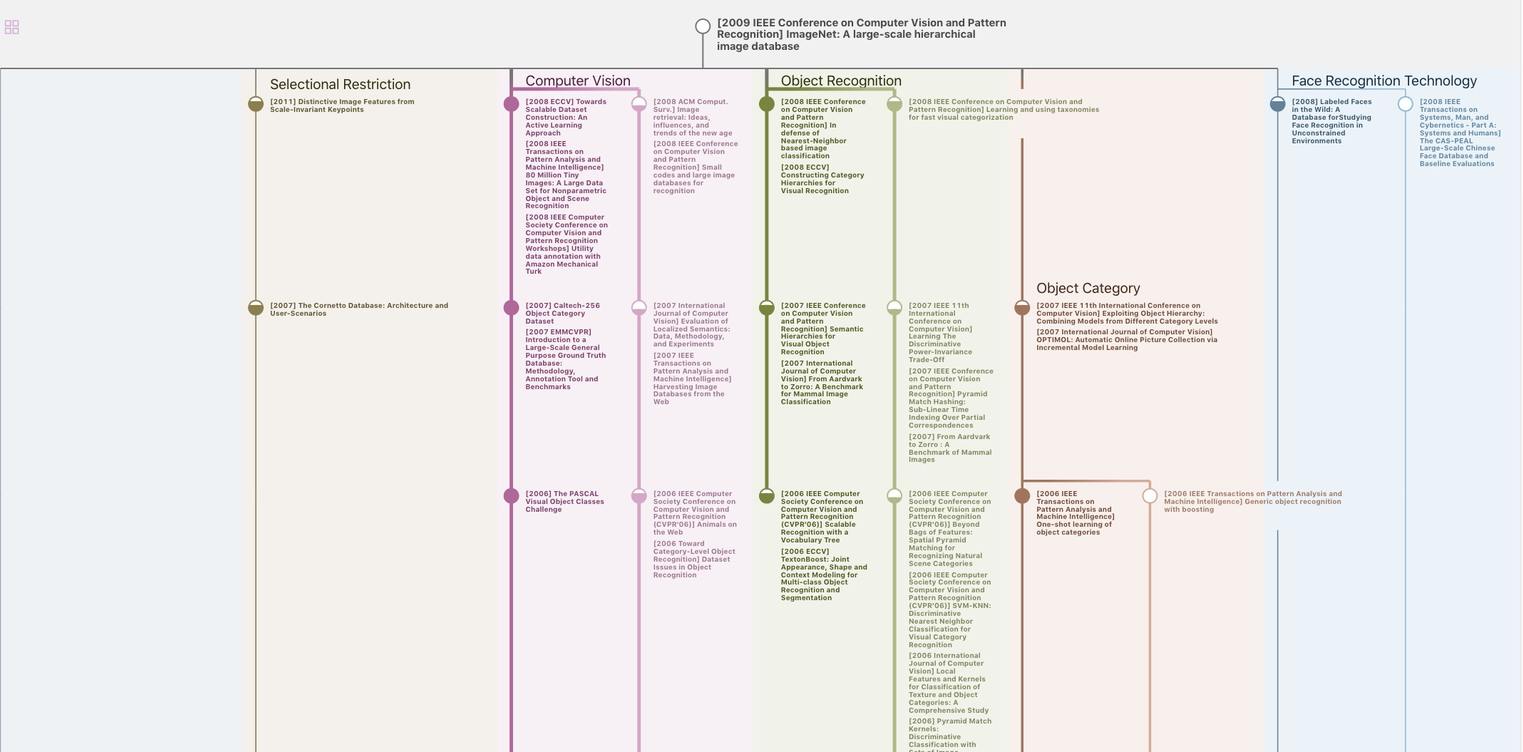
生成溯源树,研究论文发展脉络
Chat Paper
正在生成论文摘要