Efficient Low-Rank GNN Defense Against Structural Attacks
2023 IEEE INTERNATIONAL CONFERENCE ON KNOWLEDGE GRAPH, ICKG(2023)
摘要
Graph Neural Networks (GNNs) have been shown to possess strong representation abilities over graph data. However, GNNs are vulnerable to adversarial attacks, and even minor perturbations to the graph structure can significantly degrade their performance. Existing methods either are ineffective against sophisticated attacks or require the optimization of dense adjacency matrices, which is time-consuming and prone to local minima. To remedy this problem, we propose an Efficient Low-Rank Graph Neural Network (ELR-GNN) defense method, which aims to learn low-rank and sparse graph structures for defending against adversarial attacks, ensuring effective defense with greater efficiency. Specifically, ELR-GNN consists of two modules: a coarse low-rank estimation module and a fine-grained estimation module. The first module adopts the truncated Singular Value Decomposition (SVD) to initialize a low-rank estimate of the adjacency matrix, which serves as the starting point for optimizing the low-rank matrix. In the second module, the initial estimate is refined by jointly learning a low-rank sparse graph structure together with the GNN model. Sparsity is enforced on the learned low-rank adjacency matrix by pruning weak connections, which can reduce redundant data while maintaining valuable information. As a result, instead of using the dense adjacency matrix directly, ELR-GNN can learn a low-rank and sparse estimate of it in a simple, efficient, and easy to optimize manner. The experimental results demonstrate that ELR-GNN outperforms the state-of-the-art GNN defense methods in the literature, in addition to being very efficient and easy to train.
更多查看译文
关键词
Graph Neural Networks,Adversarial Attacks,Low-rank Estimation
AI 理解论文
溯源树
样例
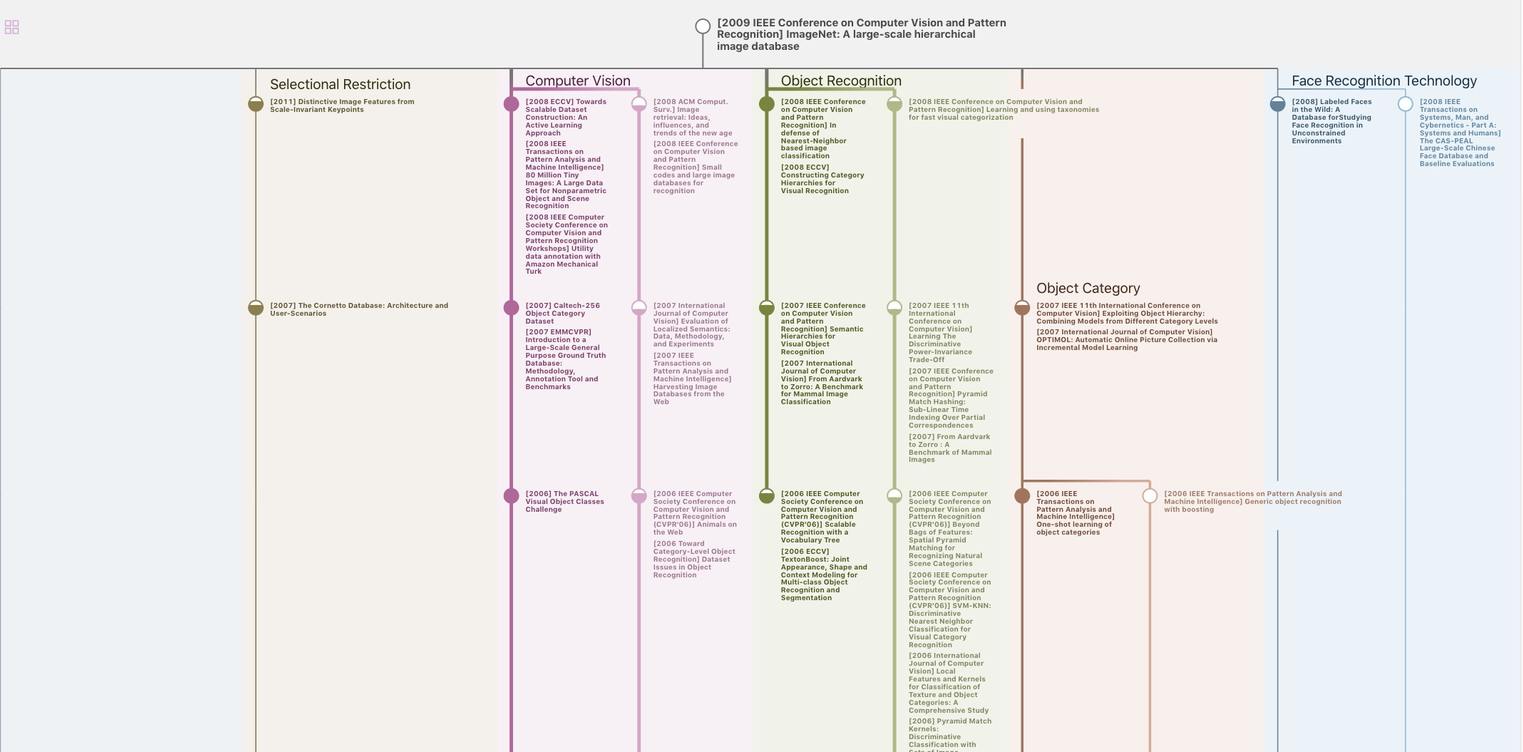
生成溯源树,研究论文发展脉络
Chat Paper
正在生成论文摘要